Making changes to any department of an organization is fraught with numerous difficulties due to the links between the operations carried out in these departments. Changes, however, are a part and parcel of economic growth, which any organization must sustain to remain competitive in the environment of the global economy. Carrying out a statistical analysis is essential to identify whether changes in question are required at the moment or whether entrepreneurship may attain success without drastic alterations to its system.
The organization under analysis can be defined as a private company limited by shares. Typically defined as a standard corporation type, which is the most common among entrepreneurs, the specified phenomenon is also viewed as the organization, which is controlled by shareholders (Erwine, 2015).
It should be noted, though, that the corporation under analysis needs an urgent solution to its current problems. Particularly, the fact that the company managers cannot identify the required amount of supplies deserves to be mentioned. Particularly, the members of the organization responsible for the provision of energy fail to come to an agreement regarding the number of transformers to be purchased (Mangan, Lalwani, & Butcher, 2011).
Defining the factors that may possibly inhibit the performance processes occurring at the organization in question, one must mention that the company clearly lacks strong leadership. The inconsistency in the analysis of the tools and supplies required to meet the customers’ demands points to the lack of efficacy of the corresponding department, i.e., Customer Relations.
Herein the need to rearrange the firm’s key processes by altering the leadership approach lies. As soon as the proper management strategy is introduced, the rates of corporate social responsibility (CSR) can be increased.
Seeing that the latter phenomenon is identified as “achieving commercial success in ways that honour ethical values and respect people, communities, and the natural environment” (Dahlsrud, 2015, p. 8), it is bound to affect the organizational behavior of the staff members in a positive manner, therefore, changing their concept of their roles and responsibilities and improving their organizational behavior patterns.
Additionally, the reconsideration of the statistical analysis approach adopted in the firm must be viewed as a priority. Despite its efficacy in gathering data, the organization members may need a more efficient tool to predict the outcomes of the approach that is currently adopted. Particularly, the methods of statistical analysis need to be redesigned, and a very strong emphasis must be put on the identification of the tendencies that can be observed in the company in terms of sales at present.
When it comes to analyzing the problem mentioned above, one must admit that the cohesive leadership style, which could serve as the tool for bridging the gap between the opponents in the organizational and promote an objective evaluation of the existing options must be brought up. Both parties involved in the conflict seem to act on behalf of the organization and for its greater good; however, their lack of critical skills points to the need to adopt an analytical approach, which will help resolve the situation and improve the current state of affairs.
Indeed, on the surface, the company lacks a cohesive and clear statistical analysis process. While one of the company members insists on carrying out the ANOVA analysis, which leads to a specific outcome, the other one points to the necessity to conduct both the ANOVA and the linear regression analysis.
The difference in the analysis results can be explained by the differences in the focuses of each; however, having a better understanding of the problem is not enough. What the organization needs at present is the creation of a negotiation approach that will guide the staff members through the procedure of choosing the statistical analysis tool that meets the company’s needs to the greatest degree.
Hence, negotiation based on the principle of a compromise and the further identification of observable trends in the number of transformers required should be viewed as the adequate steps to be taken. The analysis mentioned above should be carried out with the help of correlation and regression models (MacFarland, 2011).
The choice of the latter is predetermined by the fact that the specified models help identify the trends that can be observed among variables based on the changes in their value; thus, a comparatively accurate forecast can be made with the help of the data gathered over the course of the firm’s experience.
The type of analysis mentioned above belongs to the ANOVA model of data analysis (Calberg, 2014). According to the definition provided by Land, Smith and Waltz (2012), the specified model of data analysis is the analysis of variance in a specific set of data. In other words, the model in question offers a rather accurate analysis of the information retrieved over several years of a company’s operations.
However, when it comes to defining the disadvantages of the approach in question, one must admit that it can be deemed as rather general. On the one hand, the ability to predict future demand based on the overall trend can be viewed as a rather trustworthy method to adopt. On the other hand, generalized linear model, in general, and the ANOVA double-factor statistical analysis, in particular, have several limitations, which reduce the accuracy of the outcome.
For instance, the very manner, in which the data is organized during the analysis, i.e., the double factor inclusion, triggers a significant drop in accuracy of the estimations since the shape of functions cannot be inferred (Chan, Lettice, & Durowoju, 2011).
Nevertheless, the approach in question can be deemed as fairly efficient as it helps outline the key trend in a set of data and, therefore, locate the tendency expressed with the help of a detailed ANOVA analysis. Speaking of the tools, which can be used to calculate the trend observable in the designated set of data, the use of the MS Excel data analysis tools has to be brought up.
The reasons for using the above-mentioned tools re quite basic; while being very simple, they allow for a very accurate analysis of the information. Moreover, the adoption of the above-mentioned set of tools helps increase the speed of the analysis and, therefore, deliver the required results within a relatively short amount of time. The specified characteristics of the test to be adopted are especially significant in the realm of the global economy since a range of competitive organizations may take the place of the firm in question while its leaders are trying to identify the further set of actions to be taken.
Apart from ANOVA, the tool known as the linear regression model deserves to be mentioned as the means of analyzing the data retrieved in the course of the analysis. While it is the ANOVA test that will help define whether the approach used currently can be deemed as viable, it is the linear regression model that will allow making assumptions regarding the existing tendencies and the further change in the number of transformers used.
The tool mentioned above belongs to the family of the generalized linear model set of tools. The advantages and problems of the model are quite obvious; while providing clear directions concerning the further change in the trends for the phenomenon in question, it lacks accuracy and needs substantial support.
Similarly, the linear regression analysis is limited to its linear shape and, therefore, cannot offer an exponential point of view on the subject matter. However, it still offers a fairly reasonable evaluation of the current tendencies and, thus, should be considered a rather important tool in the statistical analysis.
As it has been stressed above, an ANOVA double-factor statistical analysis has to be used to identify whether the number of transformers suggested by the operations manager may actually help produce the amount of goods that will meet the customers’ demands. The process of the analysis can be characterized as including the necessary data into the Excel file.
In order to deliver the results required in the assignment, one will have to adopt the ANOVA tool with the help of the above-mentioned program. Specifically, the analysis of the two types of data provided in the assignment description, i.e., the number of transformers and the sales of refrigerators, will be compared so that a correlation between the two could be drawn.
Indeed, the F-value is far above the critical one, as the test outcomes show (≈57.99 compared to the required critical value, i.e., 4). The p-value, in its turn, is very low, reaching approximately 5E-10. Therefore, it can be assumed that the null hypothesis can be deemed as proven wrong and the data retrieved in the course of the experiment cannot be obtained with the help of random sampling.
Figure 1. ANOVA: Two-Factor Analysis of Variance
In addition, adopting the linear variable tool and charting the scatter plot will lead to the identification of tendencies in the above-mentioned data sets. Specifically, one will be able to identify whether he number of transformers grows and declines in direct proportion to the number of refrigerator sales.
More importantly, the exact correlation between the two values in question has been drawn; according to the analysis results, the relation between the data sets listed above can be defined as y= 0,3149x + 1233,5. The specified correlation points directly to a slow yet consistent growth of the number of transformers required as well as the fact that there is a direct relationship between the number of transformers and the number of sales (Roberts, & Russo, 2014).
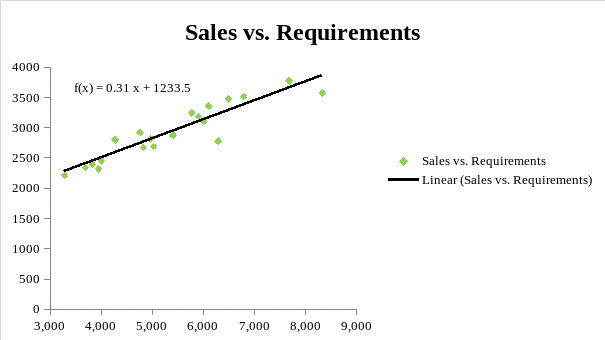
Likewise, it was crucial to use the Excel data analysis tools to carry out the ANOVA test and extract the necessary information. Although the specified tool may be viewed as lacking precision, it saves time greatly, which is of huge significance of the organization under analysis, which needs urgent assistance and has to take a decision instantly. In other words, the approach that is currently adopted in the organization and viewed as credible cannot be used further due to its lack of efficacy.
As the chart provided above shows, the sales of the company are in a direct proportion to the requirements set by the customers’ quality standards to the production offered by the entrepreneurship. In other words, A-Cat Corp. will have to update its transformers on a regular basis so that the quality of the company’s product should not drop and that the target audience should not lose their loyal customers.
More importantly, based on the above-mentioned graph, the entrepreneurship managers will be able to design the strategy that will help attract an increasingly large number of clients based on the unique price–quality ratio that A-Cat Corp. has to offer (Wang, 2012).
Specifically, the results of the experiment carried out above point graphically to the fact that the mean number of transformers has changed significantly over the specified time period. Consequently, at least 745 transformers are required to produce the necessary number of refrigerators; moreover, based on the trend line provided above, it is reasonable to assume that the designated number is only going to increase in the future.
A further analysis of the data shows that the supposition made by Mittra, the vice-president of the corporation, is quite correct, and the organization will have to deviate from the traditional pattern of product manufacturing by putting a stronger emphasis on producing a larger number of transformers. As the table provided above shows, the ANOVA test has proven the null hypothesis wrong.
The scatter plot above, in its turn, also makes it quite clear that the company will have to bear in mind that the number of transformers will only have to grow so that the refrigerator sales could increase and, therefore, contribute to the organization’s prosperity.
The reliability of the data provided is quite high. While one might question the credibility of the ANOVA test results retrieved from a rather scanty amount of information, the data set provided by the organization’s vice-president is quite enough to build, strong hypotheses on.
In other words, the fact that the data used for the analysis included sixteen entries and was gathered over the course of several (2006–2010) years shows that the information provided for the ANOVA test embraces a rather large time slot and, therefore, can be deemed as rather objective.
Consequently, there is no need to doubt the research outcomes. One must admit, of course, that there is a slight error rate in the calculations; as the ANOVA test has shown, the total error amounts to 8,538,277.1. However, given the overall test result, the error in question can be overlooked.
Making changes to any process in an organizational framework is a challenging task, since most of the processes are deeply integrated with each other and, therefore, the slightest alteration will trigger major consequences. However, when he time for a company to expand comes, the reassessment of the existing trends and the identification of the recent alterations in the demand rates, the production process, etc. have to be calculated.
Despite the fact that the changes suggested by the company’s vice-president might be viewed as unwelcome, they, in fact, allow for the further evolution of the organization and must be viewed as an opportunity for the firm to comply with the requests made by its target audience.
The choice of the ANOVA two-factor analysis of variance has proven that the organization is in desperate need for a change. Particularly, the ANOVA outcomes point to the fact that the null hypothesis regarding the lack of differences between the transformers number and the total number of the refrigerators produced by the firm is growing consistently.
Moreover, the test results allowed for the identification of a paradigm in the current performance delivered by the organization and the demand rates observed among the customers. In other words, the specified tools help identify the changes that the demand and, therefore, the production process will undergo in the future.
Reference List
Calberg, C. (2014). Statistical analysis: Microsoft Excel 2013. New York City, New York: Que Publishing.
Chan, H. K., Lettice, F., & Durowoju, O. A. (2011). Decision-making for supply chain integration: Supply chain integration. Berlin: Springer Science & Business Media.
Dahlsrud, A. (2015). How corporate social responsibility is defined: An analysis of 37 definitions. Corporate Social Responsibility and Environmental Management, 1(1), 1–14.
Erwine, C. (2015). Core statutes on company law 2015-16. New York City, New York: Palgrave Macmillan.
Land, S. K., Smith, D. B., & Waltz, J. (2012). Practical support for lean Six Sigma software process definition: Using IEEE software engineering standards. New York City, New York: John Wiley & Sons.
MacFarland, T. (2011). Two-way analysis of variance: Statistical tests and graphics using R. Berlin: Springer Science & Business Media.
Mangan, J., Lalwani, C., & Butcher, T. (2011). Global logistics and supply chain management (2nd ed.). New York City, New York: John Wiley & Sons.
Roberts, M., & Russo, R. (2014). A student’s guide to analysis of variance. New York City, New York: Routledge.
Wang, L. (2012). Application of LADAR in the analysis of aggregate characteristics. Washington, DC: Transportation Research Board.