Abstract
As firms are increasingly focusing on core competencies, there has been a growing interest among organizations to outsource a broader gamut of logistics services. The logistics fraternity is evolving innovative models tailored to meet end customer solutions and adhere to channel wide requirements in the supply chain network. Conceptualizing such a paradigm, 4PL operators have built on a foundation of providing supply chain info-mediary services that act as a barometer of channel collaboration. Such an inter-mediator requires competency to manage multi tiered echelons through an efficient and effective intelligent network, apart from providing real time information sharing, optimizing channel resources and enhancing decision synchronization. Choosing the right lead logistics partner is thus a major strategic decision. A few organizations consider qualitative criteria for supplier decision making and majority treat it as an optimization problem. For optimum decision making both quantitative and qualitative measures need to be integrated. This study provides taxonomy of comprehensive metrics arranged hierarchically in a process based systematic perspective to build a framework for selection and evaluation of a lead logistics partner. The metrics are aligned based on the organizations business strategy. The chosen set of metrics is then used to evaluate the performance of the 4PL operator. The objective of this study is to introduce a comprehensive decision making methodology for the selection of a 4PL logistics service provider. The relevant metrics based on a model is selected and categorized using factor analysis.
Introduction
To focus on core competencies, firms are increasing looking at outsourcing or externalization of services. In order to meet the growing requirements of its users, logistics service providers are also offering customized expertise and experience which would otherwise be difficult to acquire or costly to develop in-house by its users. Depending upon user requirements, logistics service providers are offering various forms of solutions. The most common of these are warehousing, outbound transportation, customs brokerage and inbound transportation.
This study will endeavor to differentiate the core offerings of two leading forms of logistics service providers namely Third Party Logistics and the emerging Fourth Party Logistics, report on the criteria that have been used for evaluation of logistics partner and the current evaluation process used for selection.
4PL is a business process outsourcing. It redesigns the supply chain network by aligning business strategy with Supply Chain strategy and integrates operations inter and intra participating supply chains. It optimizes the network to take charge of full operational responsibility for multiple functions and processes of the supply chain.
4PL forms association, alliances and consortiums with key stake holders of the chain including 3PL operators at various levels and links, in managing the supply chain processes to a measured set of activities required for a specific output. In other words the 4PL operator would utilize best of breed approach in meeting end user requirements for a cost effective and efficient management.
Although 3PL logistics service providers have been able to provide asset based resource oriented services, they could not meet the increased requirements of its clients to manage a global supply chain, integrating multi-echelon entities without favoring its own assets. In addition, customers need increased visibility, network optimization and decision synchronization which is imperative for a comprehensive supply chain solution. Gattorna (1998) claims that while the business practice of outsourcing 3PL is the norm, 4PL has emerged to tackle supply chain challenges and provide an overall benefit to its clients. Thus, it may be inferred that in the realm of current supply chain imperatives, a lead logistics provider in the form of 4PL architecture is an important development to meet the growing requirements of its clients.
Aims of study
The aim of the research of this paper is to:
- To identify currently adopted algorithm used for multi criteria decision making in evaluation of logistics service provider.
- To develop the most appropriate evaluation framework taking into account the choosing logistics problem and selection appropriate model.
Goal of the Solution
The study is to investigate and understand probability of goods reaching the destination without delay and how Bayesian decision making model is used solve the problem. It will also help organizations understand how statistics and probabilities are used in real business environment
Criteria for selection of transshipment route
Several criteria have been proposed for selection of logistics partner, however the quantity and nature of measurements proposed differed significantly. Huang et al., (2005) enumerated Cost, Quality, On-Time Delivery and Flexibility as key factors for selection of a vendor. Jharkharia et al., (2005) has emphasized Compatibility, Cost and reputation as determinants for selection of 4PL. Zhang et al., (2005) has identified several criteria like client relationship, labor relations, quality, capabilities and general company considerations like price, financial stability, location, etc for evaluation of a logistics vendor. Aghazadeh (2003) proposed 4 criteria – similar value, information technology, key management and relationship. Knemeyer et al., (2004) has provided to assess the selection of logistics vendor from relational marketing perspective with six dimensions. A few authors have highlighted the significance of price. A few others have proposed service performance and quality requirements as key criteria The selection of a service provider based on criteria which meets the organizational goals of the outsourcing company is complex because of conflicting nature of criteria and contrasting performance of different vendors on these criteria (Huang and Keskar, 2006). Different methodologies have been adopted to address the complexity of decision making to handle the vast multitude of criteria (Weber et al., 1991).
The traditional measures of criteria development have been mired with the following shortcomings.
- Lack of alignment with organizational strategy
- Too much focus on cost factors
- Lack of balanced approach
- Lack of process based approach in a cross functional framework
- System thinking perspective
- More oriented towards local optimization rather then channel optimization.
- Lack of customer and market orientation.
Based on above factors, it may be inferred that since the nature of existing criteria and measurement methods have been developed for resource oriented logistics service providers, the current standards may not be enough and applicable for evaluation of 4PL which is based on customer development resources. The competence of 4PL is different to 3PL core offerings. The measurement system for assessing the performance of 4PL should provide a multi dimensional tool to identify and facilitate the understanding of processes aligned to customer centric supply chain strategy.
The supply chain council in 1997 provides for a process based approach to supply chain management through an effective and cross industry framework called Supply-Chain Operations Reference model. This model was based on a process oriented language for communicating between supply chain partners. The SCOR Model aims to measure the performance objectively, identify performance improvement areas and influence future Supply Chain Management software development. It is developed around 5 main management decision areas – Plan, Source, Make, Deliver and Return. It is a hierarchical process model with 4 levels. Level 1 is a top level that deals with process types, level 2 is a configuration level and deals with process categories, level 3 is a process element level and is a lowest level in the SCOR Model. Level 4 is the implementation level and many be customized based on the organization’s practices and thus is not defined in the SCOR Model. The merits of SCOR model are:
- Process Reference Model which integrates Business Process Reengineering, benchmarking, and characterize management practices for best performance into a cross functional framework.
- The model encompasses customer interactions, Product (Physical material and service) transactions from supplier’s supplier to end consumer, market interactions from aggregate demand to order fulfillment.
- Addresses the performance measurement of key processes spanning multi echelons and multi function in five categories -reliability, responsiveness, flexibility, cost and assets. Currently, there are 13 level 1 metrics in these categories.
Several leading organizations including global conglomerates have adopted SCOR framework to improve their Supply Chain processes. Few examples include AT & T Wireless aligned their operations strategy using SCOR to turnaround its faltering organization, Daimler Chrysler introduced SCOR to improve its lean distribution process, Ford Motor Company used SCOR as a cross function metric integration tool for performance management (Supply Chain Council, 2007). There are a host of other empirical evidences regarding the efficacy of SCOR as a driving tool to improve and manage supply chain processes.
Thus, it is only logical that our construct of criteria development for evaluation of 4PL operator be based on SCOR model. As 4PL will be responsible for entire operational management of Supply Chain network, hence the metrics to measure the performance of the network may be used as criteria for evaluating the ability of 4PL to deliver the required output. Reasons for development of criteria based on SCOR and its application for evaluation are:
- It is a standardized Supply Chain model
- It is based on process oriented framework
- It is system thinking approach for identifying, evaluating and monitoring supply chain performance.
- The SCOR model links process description and definition with best practice, metrics and technology.
- It is an ideal tool for describing, analyzing and improving supply chain.
The objective of this study is to provide a comprehensive methodology, through a set of performance metrics based on SCOR framework that firms can employ for decision making in selection and evaluation of a 4PL operator.
Limitations of the study
Because this is an exploratory study it will mean that more time will be required to carry out the research in order to find the relevant information that will reflect the issue. Also another limitation is that of availability of funds because the study will require the researcher.
Literature review
Bayesian data distribution considers situations where modeling and inferences are different from the normal or classical inference. Bayesian inference considers conditions distribution with probabilities involved for the data observed. The model considers factors such as confidence levels of 99% or any percentage. This model considers joint distribution for the observed data which may be non distributive and may consider multi levels. However in some instances, prior distributions is used where academic or expert knowledge to measure the parameters of the knowledge (Arjas, E. and Heikkinen, J. 1997).
Prior distributions
In prior distribution or prior probability the structure is different from posterior because it used prior information of the structure of the system. Prior distribution has two forms of distribution that is conjugated prior and informative prior. One of them considers hierarchy of a model while other considers only limited information. One normally have uniform prior distribution which considers a number of factors or choices that will be used in coming up with a solution to the problem.
Conjugate distribution – conjugate distribution considers parameters which have a single element or elements from a single family. The condition is that these elements must be of the same class in order to computation or prior distribution to be successive. This conjugate distribution is important because it helps the expanded model to be reserved or remain the same. The model can be expanded using the same class of parameters without changing the system very much. Informative prior – informative prior involved a provision of information which is intentionally aimed at making the model knowledge known. This is useful because it provides information which may change the model under use.
Directed acrylic graphs
This is graphical presentations of prior or posterior probability distribution of data between some variables. These graphs use some nodes and encodes. It represents random variables with conditionals dependant relationship among each other. The graph has directed edges meaning that they restrict the conditions of variables. This means that they use conditional probability as well as directed mart of analysis. For example the graph below shows directed acrylic graph.
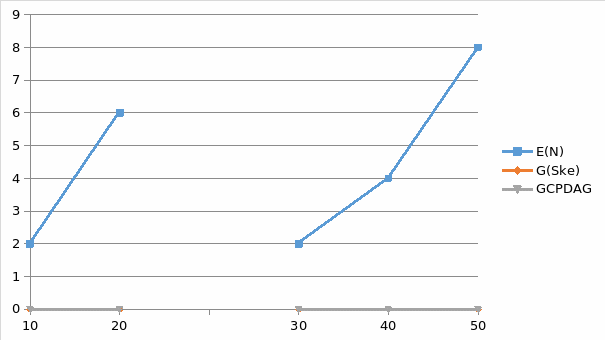
The posterior distribution
In modern Bayesian statistics posterior distribution is the distribution which comes after the analysis. It gives additional information which may changes the analysis of the probabilities chosen.
Bayesian inference
Bayes factors: – Bayes factors are those factors in the Bayesian model which are dominant when the model is being tested. They are ratio like and they are used in testing the model to ensure prior and posterior information has been taken into consideration when the model is being made. These factors allow multiple testing or multiple hypotheses. This is when comparing the two models. When testing the Bayesian model it becomes easier because bayes factors are known. The factors always use the same dependent variable and different independent variables.
For example in the case of apple corporation wishing to choose two competing route, Routs R 1 and Route R 2 which relates to transporting electronic equipment from Los Angeles to an East African country different parameter will be considered. Q 1 and Q2. The aim will be to know which route is best in ensuring that the goods reach east Africa without much problem.
First we shall estimate prior dist5ributiosn for Q 1 and Q2 and prior probabilities for R 1 and R2.
The posterior odd ratio in favor of R1 over R2 = posterior prior odd divide by data times Bayes factor. The bayes factor is found by the following formula.
Bayes factor = B (x) n(R1 (x)/ p (R1) n(R2 /x) / p (R2)
Confidence regions:– In hypothesis testing in Bayesian model confidence regions or intervals are very important. Confidence regions is a region which shows a relationship between confidence regions and hypothesis testing for examples when carrying out Bayesian hypothesis test and is rejected the confidence region for the means will not be outside the hypothesis mean. The same case when now hypothesis’ is not rejected the level of confidence as the hypothesized mean (Walker, S., Damien, P., Laud, P. and Smith, A. 1999).
Markov chain Monte Carlo
The Monte Carlo method is a simulation technique using random numbers. Monte Carlo simulation techniques are used in business and industry to solve problems that are extremely difficult or involve a large number of variables. The steps for simulating real-life experiments in the Monte Carlo method are
- List all possible outcomes of the experiment.
- Determine the probability of each outcome
- Set up a correspondence between the outcomes of the experiment and the random numbers.
- Select random numbers from a table and conduct the experiment.
- Repeat the experiment and tally the outcomes.
- Compute any statistics and state the convulsions
Statistical tests such as the z, t and f tests are called parametric tests. Parametric tests are statistical tests for population parameters such as mean, variances, and proportions that involve assumptions about the populations from, which samples were selected.
One assumption is that these populations are normally distributed. But what is the population is a particular hypothesis-testing situation is not normally distributed. But what if the population in a particular hypothesis testing situation is not normally distributed? Statisticians have developed a branch of statistics known as nonparametric statistics or distribution – free statistics to use when the population from which the samples are selected is not normally distributed. Nonparametric statistics can also be used to test hypothesis that do not involve specific parameters.
Non parametric tests and statics can be used in pace of their parametric counterparts when the assumption of normality cannot be met. However, one should not assume that these statistics are a better alternative than the parametric statistics. There are both advantaged and disadvantaged in the use of non-parametric methods. There are advantages that nonparametric methods have over parametric methods:
- They can be used to test population parameters when the variable is not normally distributed.
- They can be used when the data are nominal or ordinal
- They can be used to test hypothesis’ that do not involve population parameters
- In most cases, the consumptions are easier that those for the parametric counterparts.
- They are easy to understand.
There are three disadvantaged of non parametric methods:
- They are less sensitive than their parametric counterparts when the assumptions of the parametric methods are met. Therefore, larger differences are needed before the null hypothesis can be rejected.
- They tend to use less information than the parametric tests. For example the sign test required the researcher to determine only whether the data values are above or below the median, not how much above the medial each value is.
- They are less efficient than their parametric counterparts when the assumptions of the parametric methods are met. That is larger sample sizes are needed to overcome the loss of information. For example the nonparametric sign test is about 60% as efficient as its parametric counterpart the z test. Thus, a sample size of 100 is needed for use of the sign test compared with a sample size of 60 for use of the z test to obtain the same results.
Since there are both advantages and disadvantages to the nonparametric methods, the researcher should use caution in selecting these methods. If the assumptions can be met, the parametric methods are preferred. However, when parametric assumptions can not be met, the non parametric methods are a valuable tool for analyzing the data.
Conditional probabilities
The conditional probability of an event B in relationship to an event A was defined as the probability that event B occurs after event A has already occurred. The conditional probability of an event can be found by dividing both sides of the equation for multiplication rule 2 by p (A), as shown:
- P(A)and B) = P (A.P (B) A)
- P(A)and B) = P (A.P (B) A)
- P(A) P(A)
- P(A AND b) = p (B/A
The Probability That The Second Event b occurs given that the first event A has occurred can be found by diving the probability that both events occurred by the first event has occurred. The formula is:
- P(B/A) = P(A and B)
- P(A)
Hence the probability of selecting a while chip on the second draw given that the first chip selected was black is 5/7. The probability that Sam parks in a no-parking zone and gets a parking ticket is 0.06 and the probability that Sam cannot find a legal parking space and has to park in the parking zone is 0.20. on Tuesday, Sam arrives at school and has to park in a no-parking zone. Find the probability that he will get a parking ticket.
Then:
- P(T/N) = P(N and T) = 0.06 = 0.30
- P (N) 0.20
Hence Sam has a 0.30 probability of getting a parking ticket, given that he parked in a no-parking zone.
The conditional probability of events occurring can also be computed when the data are given in a table form. Which is represented by the area in the intersection or overlapping part of the circles A and B, divided by the area of circle A. The reasoning here is that if one assumes A has occurred, then A becomes the sample space for the next calculation and is the denominator of the probability fraction P(A and B) The numerator (PA and B) represents the probability of the part of B that is contained in A. hence, P(A and B) becomes the numerator of the probability fraction P(A and B) imposing a condition reduces the samples.
Statistical analysis
The has a 70% chance of succeeding in the international market. In order to succeed in the international market there are five to make on strategic logistics partner and three of the choices are 4PLlogistic partner and two of them are 3PL logistic partners. The task is to simulate 50 sampling of the 5 choices and record the number 4PL logistic partners on each sampling, then calculate the relative frequency that the company selects 4PLlogistic partner or more of the 5 potential partners.
First assign the digits 0 to 6 (70%) to the outcome selection of 4PLlogistic partner and 7 to 9 (30%) to the outcome 3PLlogistic partner. Next clear lists L1 and L2 (Clrlist L1. L2) so that the results of our simulation can be stored there, and set a counter to 1 (1-C). The variable C is a count of the number of sampling (in programming, such a variable is known as a counter.) the command randint (0,9,5) – L1 simulates 5 choices and stores the results in L1. The command sum (L1 ≥ 7 and L1 ≤9) – C uses Boolean logic to count the number of 3PLlogistic partner (7, 8, or 9)_ in the 5 simulated choices and stores this counts as the C-the item in the list L2 (C is currently 1). Increase the count to 2 (1+C-C) and repeat the process. Continue executing these there commands until you have performed several sampling. When you stop, you should have several numbers in L2, one of each sampling.
The command sum (L2 ≥3 and L2 ≤5) /N calculates the relative frequency of 4PLlogistic partner and approximate the probability that the company will select 3 4PLlogistic partner or more 5 choices. Rather than continue accumulating results this way, do the following. First enter these two lines:
- Clrlist L1 , L2
- 1 – C
Next, combine the remaining commands, separated by a colon, as follows:
- randInt (0,9,5) – L1 : sum (L1 ≥ 7 and L1 ≤9) – L2 ( C ) : 1 + C —C
After you execute the third line, go to the STAT/EDIT mode and look at the two lists L1 and L2 simulating the first sampling of 5 choices, and there should be one number in L2. which is the count of 4PLlogistic partner in the first sampling. Quit to the home screen, press 2nd/ENTRY to recall the last command, and press ENTER. Continue with the keystrokes 2nd ENTRY/ENTER until you have 25 sampling. Then you can either scroll through list L2 or count the number of 3s, 4s, and 5s, or you can have the calculator determine the relative frequency with the command sum (L2 ≥ 3 and L2 ≤ 5) 25. Compare your results with those of your classmates. If you worked Exercise 5.57 using the random table, compare those results with your calculator.
Results
- randInt (0,9,5) – L1 : sum (L1 ≥ 7 and L 1 ≤ 9) — ( c) : 1 + C — C2
To augment the limitations of the Bayesian model and use it as a platform to construct our metrics and analyze the performance of model on these measures, I propose one more performance dimension – Information Technology in addition to Reliability, Responsiveness, Flexibility, Cost and Assets. We categorize the metrics based on these six dimensions. The procedure for metrics development follows:
- A thorough literature review compiling taxonomy of metrics from academic journals, research papers and articles, including web based sources. The definition of each metric is classified.
- The relevant measures were then categorized according to their applicability based on its characteristics, according to the six dimensions in their relevant category on the hierarchy model.
- Each metric under respective category is reviewed to avoid duplicity of the same measurement.
The research approach for this study follows the process of investigation concerned with metrics and measurement systems for evaluation. Taxonomy of metrics published in research articles, journals, books and web based materials will be collected. The respective metrics were categorized according to six dimensions of the model (Reliability, Responsiveness, Flexibility, Cost, Assets and Information Technology) as elucidated. The model is also validated through an illustrative example.
Results (output) and discussion
I have simulated this case study using Monte Carlo simulation to arrive at the data for the criteria chosen. The data has been analyzed and has been tested and prior and distribution using confidence levels, quantitative and qualitative variables for both routes.
The logistic partner were compared using compatibility costs quick delivery and safety of the product to reach the market quickly. The core functionality of the system or model chosen was tested using resources that are available to the company. The logistic partner were not paired in terms of details but differences in means of costs were subjected to confidence intervals.
The results has been obtained and analyzed from this case sturdy. The graph for the data has been drawn.
Recommendation and conclusion
The results obtained from this analysis are not adequate to give a generalization in relation to the two routes. Data research is need to be able to conclusively state that the model failed or worked.
To obtain information and make inferences about a large population, researchers select a sample. A sample is a subgroup of the population. Using a sample rather than a population, researchers can save time and money, get more detailed information and get information that otherwise would be impossible to obtain.
The four most common methods researchers use to obtain samples are random, systematic, stratified, and cluster sampling methods. In random sampling, some type of sampling, the researcher selects every kth person or item after selecting the first one at random. In stratified sampling, the population is divided into subgroups according to various characteristics and elements are then selected at random from the subgroups. In cluster sampling, the researcher selects an intact group to use as a sample. When the population is large, multistage sampling (a combination of methods) is used to obtain a subgroup of the population.
Researchers must use caution when conducting surveys and designing questionnaires, otherwise, conclusions obtained from these will be inaccurate.
Most sampling methods use random numbers, which can also be used to simulate many real-life problems or situations. The basic method of simulation is known as the Monte Carlo method. The purpose of simulation is to duplicate situations that are too dangerous, too costly, or too time-consuming to study in real life. Most simulation techniques can be on the computer of calculator, since they can rapidly generate random numbers, count the outcomes, and perform the necessary computations. Sampling and simulation are two techniques that enable researcher to gain information that might otherwise be unobtainable.
References
Aghazadeh, S.M (2003) ‘How to Choose an Effective Third Party Logistics Provider’, Management Research News, 26:7, 50-58
Arjas, E. and Heikkinen, J. (1997). An algorithm for nonparametric bayesian estimation of apoisson intensity, Computational Statistics 12: 385–402.
Barron, A., Schervish, M. J. and Wasserman, L. (1999). The consistency of posterior distributions in nonparametric problems, The Annals of Statistics 27: 536–561.
Gelman G., Carlin J. B, and Stern H and Rubin D.B;.Bayesian Data Analysis, second edition.
Berger, J. and Guglielmi, A. (2001). Bayesian testing of a parametric model versus nonparametric alternatives, Journal of the American Statistical Association 96: 174–184.
Carota, C. and Parmigiani, G. (2002). Semiparametric regression for count data, Biometrika 89: 265–281.
Chipman, H. A., George, E. I. and McCulloch, R. E. (1998). Bayesian CART model search (C/R:p948-960), Journal of the American Statistical Association 93: 935–948.
Clyde, M. and George, E. (2000). Flexible empirical bayes estimation for wavelets, Journal of theRoyal Statistical Society, Series B 62: 681–698.
Conigliani, C., Castro, J. I. and O’Hagan, A. (2000). Bayesian assessment of goodness of fit against nonparametric alternatives, Canadian Journal of Statistics 28: 327–342.
DiMatteo, I., Genovese, C. R. and Kass, R. (2001). Bayesian Curve Fitting with Free-Knot Splines,Biometrika 88: 1055–1071.
Dominici, F. and Parmigiani, G. (2001). Bayesian semi-parametric analysis of developmental toxicology data, Biometrics 57: 150–157.
Florens, J.-P. and Rolin, J.-M. (2001). Simulation of posterior distributions in nonparametric censored analysis, Technical report, Universit´e des Sciences Sociales de Toulouse.
Gattorna, J (1998) Strategic Supply Chain Alignment, Gower Publishing Ltd, Hampshire
Gelfand, A. E. and Kottas, A. (2002). A Computational Approach for Full Nonparametric Bayesian Inference under Dirichlet Process Mixture Models, Journal of Computational and Graphical Statistics 11: 289–305.
Giudici, P., Mezzetti, M. and Muliere, P. (2003). Mixtures of dirichlet process priors for variable selection in survival analysis, Journal of statistical planning and inference 111: 101–115.
Huang, S.H, Sheoran, S.K and Keskar, H (2005) ‘Computer-Assisted Supply Chain Configuration Based on Supply Chain Operations Reference Model Model’, Computers & Industrial Engineering, 48, 377-394
Huang, S.H and Keskar, H (2007) ‘Comprehensive and Configurable Metrics for Supplier Selection’, International Journal Production Economics, 105, 510-523
Ishwaran, H. and Takahara, G. (2002). Independent and identically distributed Monte Carlo algorithmsfor semiparametric linear mixed models, Journal of the American Statistical Association 97: 1154–1166.
Ishwaran, H. and Zarepour, M. (2000). Markov chain Monte Carlo in approximate Dirichlet and beta two-parameter process hierarchical models, Biometrika 87: 371–390.
Knemeyer, A.M and Murphy, P.R (2004) ‘Evaluating The Performance of Third-Party Logistics Arrangements: A Relationship Marketing Perspective’, Journal of Supply Chain Management, 40:1, 35-5
Jharkharia, S and Shankar, R (2005) ‘Selection of Logistics Service Provider: An Analytic Network Process (ANP) Approach’, The International Journal of Management Science, 35, 274-289
Paddock, S., Ruggeri, F., Lavine, M. and West, M. (2003). Randomised Polya Tree Models for Nonparametric Bayesian Inference, Statistica Sinica 13: 443–460.
Salinas-Torres, V. H., Pereira, C. A. B. and Tiwari, R. (2002). Bayesian Nonparametric Estimation in a Series System or a Competing-Risks Model, Journal of Nonparametric Statistics 14: 449–458.
Walker, S., Damien, P., Laud, P. and Smith, A. (1999). Bayesian nonparametric inference for distributions and related functions (with discussion), Journal of the Royal Statistical Society, Series B 61: 485–527.
Zhang, H, Li, X, Liu, W, Bing, L and Zhang, Z (2004) ‘An Application of AHP in 3PL Vendor Selection of A 4PL System’, Systems, Man and Cybernetics, IEEE International Conference on, 2, 1255-1260