Introduction
According to Baus, Wood, Pollard, Summerfield, and White (2013), “diabetes mellitus is the seventh leading cause of death in the United States and is a major contributor to decreased life expectancy, increased rates of heart disease, stroke, kidney failure, lower-limb amputations, retinopathy, and adult-onset blindness” (p. 1f). Moreno-Iribas et al. (2017) also observe that according to the global statistics, the prevalence of this adverse condition reaches 9% among adults, age 18 and older, and only in 2012, the number of people died due to diabetes type 2 was approximately 1.5 million. It is possible to say that timely identification of pre-diabetic patients is of great value as it can largely help minimize the health risks and negative consequences of the disease. Nowadays, the data needed to prevent diabetes is usually collected throughout regular screenings conducted whenever a patient refers to a hospital, as well as by using various lab tests. While the conventional approach has some major disadvantages including time and cost inefficiency, the implementation of an electronic health record (EHR) database to systematically identify pre-diabetic patients may be of great assistance in diabetes prevention efforts.
EHR Database Integration
The EHR system can be regarded as computerized data related to medical records and related processes and shared through a hospital infrastructure. The research evidence demonstrates that EHR databases can now be effectively used to continuously monitor the clinical outcomes of patients. For instance, as stated by Kersting and Weltermann (2016), EHRs can extract and summarize various clinical data such as diagnosis codes, lab test results, and patient records, which may help evaluate the overall quality of care, identify specific populations of patients that may need extra care and provide hints to recall particular information. Following the given principle, the suggested EHR database will be managed to identify patients at risk of diabetes development. The information needed to intervene in the problem includes body mass index (BMI), age, family history, elevated blood glucose levels, previous records of such conditions as hypertension and hyperlipidemia, etc. (Baus et al., 2013). These data on clinical biomarkers, patient demographics, health history, and overdue follow-ups will serve as landmarks during the identification process. In case any of the health risks are found, a standardized online/print letter will be sent to a patient, as well as his/her healthcare provider, reminding them to obtain testing and repeat screening.
Entities and Attributes
The entities that will be pulled from the EHR database are patients (1) and interventions (2). The relationships between these entities will be defined by the attributes they have. The attributes of entity 1 will be determined by using previous medical records collected throughout 12 or more months. They will be selected by following the guidelines related to the baseline prevalence of clinical biomarkers for diabetes as stated in the International Classification of Diseases. Its attributes will include age; BMI; last blood glucose level ranging from 100 to 125 mg/dL; such documented conditions as “hypertension, hyperlipidemia, gestational diabetes, family history of diabetes, or cardiovascular disease” (Baus et al., 2013, p. 1f). Each of these categories will be linked to the attributes of entity 2 − various types of interventions and testing strategies.
Attributes of Entity 1
According to the American Diabetes Association, overweight and obese adults, and all adults over 45 years old should be regularly screened in a clinical setting implementing either a fasting plasma glucose test, A1C blood test, or oral glucose tolerance test (Kirkman et al., 2012). The attributes of age and BMI (more than 25) will thus be considered the crucial ones in the identification process. The operational definitions for them will be years and BMI respectively. The datasets will be structured: age <45 or =/> 45; and BMI <18.5 (underweight), 18.5-24.9 (normal), 25-29.9 (overweight), and >30 (obese).
Normoglycemic fasting plasma glucose levels in the range from 100 mg/dL to 125 mg/dL are usually observed in patients with diabetes and are associated with multiple cardiovascular complications (Shaye, Amir, Shlomo, & Yechezkel, 2012). Thus, the fact that a patient had this indicator above the normal range may be regarded as a sign of a pre-diabetic condition. The operational definition for the given attribute is mg/dL. The dataset will be structured: <98 (normal), 99 mg/dL (borderline high), 100–125 mg/dL (high).
Lastly, the unstructured data will be presented for the category of attributes associated with multiple co-morbid and predictive conditions: hypertension, genetic predisposition, etc. This type of information will be recorded in the form of notes. The operational definition of these data will be the presence of the previously established diagnoses in the medical record (present/not present). The given category of attributes is included because the listed health conditions have some common pathways with diabetes. For instance, both diabetes and hypertension may occur as a result of the stimulation of the sympathetic nervous system (e.g., by chronic stress), increased renin-angiotensin-aldosterone system activities, etc. (Cheung & Li, 2012). Since their pathways interact with each other, the presence of one condition may be a predictor of the other. Therefore, additional screening and testing should be conducted for those patients who have hypertension, hyperlipidemia, etc.
Attributes of Entity 2
Such targeted follow-up strategies as glucose and lipid content testing, lifestyle modifications, medication intake and adherence, and patient education can be included in the suggested database. The datasets in this category will be unstructured and recorded by a nurse in a free form. Each attribute will be operationally defined according to the framework suggested by Jonkman et al. (2016). The researchers proposed a model in which interventions are measured based on the quality of information transfer: symptom monitoring, enhancement of problem-solving skills, increase in physical activity, modification of dietary intake, and improvement of medication adherence (Jonkman et al., 2016). Different intervention attributes will be automatically selected considering the identified individual patient characteristics. For instance, in case a patient has a history of hypertension and is previously prescribed with medication, it may be required to revise his/her medication adherence to increase blood pressure control (Ramli, Ahmad, & Paraidathathu, 2012).
Relationships Between Entities and Attributes
The relationships between each of the proposed attributes of entity 1 and entity 2are dynamic and multilateral, i.e., each of the identified health factors may require more than one intervention. Every attribute of entity 1 signifies that a patient is at risk of diabetes development. Subsequently, it leads to the selection of a multifaceted intervention strategy. The primary links between entities and their attributes can be schematically demonstrated as follows:
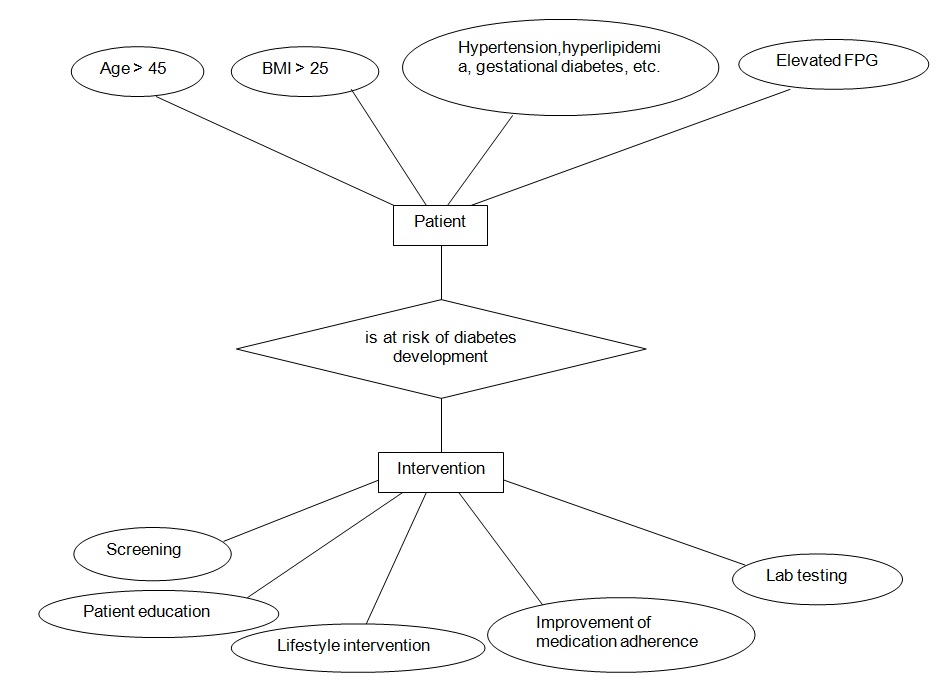
Conclusion
According to Albu et al. (2013), the utilization of the EHR database for the management of pre-diabetes can be associated with multiple advantages including the opportunity for timely and immediate intervention and prevention of significant threats to patients’ health, lifestyle changes at a broader community level, stimulation of new policy development that would support healthcare quality improvement initiatives and efficiency of service, etc. It is possible to say that the suggested EHR database management system can lead to such positive outcomes as well. However, the major advantage of this database is that it covers all the main areas of concern associated with the pre-diabetic condition and includes measurable attributes, which can help healthcare providers and population managers at the hospital easily integrate a new community-based prevention strategy at the hospital-wide level and significantly improve the quality of service.
References
Albu, J., Sohler, N., Matti-Orozco, B., Sill, J., Baxter, D., Burke, G., & Young, E. (2013). Expansion of electronic health record-based screening, prevention, and management of diabetes in New York City. Preventing Chronic Disease, 10, E13.
Baus, A., Wood, G., Pollard, C., Summerfield, B., & White, E. (2013). Registry-based diabetes risk detection schema for the systematic identification of patients at risk for diabetes in West Virginia primary care centers. Perspectives in Health Information Management, 10(Fall), 1f.
Cheung, B. M. Y., & Li, C. (2012). Diabetes and hypertension: Is there a common metabolic pathway? Current Atherosclerosis Reports, 14(2), 160-166.
Jonkman, N. H., Schuurmans, M. J., Jaarsma, T., Shortridge-Baggett, L. M., Hoes, A. W., & Trappenburg, J. C. A.-X. (2016). Self-management interventions : Proposal and validation of a new operational definition. Journal of Clinical Epidemiology, 80, 1-22.
Kersting, C., & Weltermann, B. (2016). Electronic reminders to facilitate longitudinal care: A mixed-methods study in general practices. BMC Medical Informatics and Decision Making, 16, 148.
Kirkman, M. S., Briscoe, V. J., Clark, N., Florez, H., Haas, L. B., Halter, J. B., … Swift, C. S. (2012). Diabetes in older adults. Diabetes Care, 35(12), 2650-2664.
Moreno-Iribas, C., Sayon-Orea, C., Delfrade, J., Ardanaz, E., Gorricho, J., Burgui, R., … Guevara, M. (2017). Validity of type 2 diabetes diagnosis in a population-based electronic health record database. BMC Medical Informatics and Decision Making, 17, 34.
Ramli, A., Ahmad, N. S., & Paraidathathu, T. (2012). Medication adherence among hypertensive patients of primary health clinics in Malaysia. Patient Preference and Adherence, 6, 613–622.
Shaye, K., Amir, T., Shlomo, S., & Yechezkel, S. (2012). Fasting glucose levels within the high normal range predict cardiovascular outcome. American Heart Journal, 164(1), 111-116.