Professional Summary
Readmission trends carry major implications, especially in medical situations. They have an impact on healthcare outcomes and related costs, serving as a stand-in for measuring the effectiveness of patient care. Understanding these patterns is critical since readmissions burden the healthcare system by increasing costs. Pugh et al. (2021) highlight the importance of evidence-based practices in reducing readmissions and note that employing a more comprehensive approach can provide superior outcomes.
These trends also demonstrate a hospital’s dedication to continuous quality improvement. The SNHU Hospital’s readmission patterns are the main subject of the quality evaluation. The research will comprise data collection, analysis, and benchmarking to evaluate the standard of patient care with reference to readmissions at SNHU Hospital.
Rationale for Data-driven Approach
For a variety of compelling reasons, the data-driven approach is regarded as the most appropriate and successful methodology for this quality assessment. According to Lejarza et al. (2023), the data-driven approach highlights the importance of evidence-based decision-making in the healthcare business, where patient outcomes are critical. The strategy also allows for the identification of systemic flaws in organizational procedures, patient care, and post-discharge protocols (Lejarza et al., 2023). A data-driven strategy ensures flexibility and responsiveness to new scenarios in the ever-changing environment of healthcare, where patient demographics and medical processes are always altering.
Justification for Quality Assessment
There are various reasons to conduct a quality review on readmission patterns at SNHU Hospital, all of which have significant implications. Readmissions, for example, are a symptom of potential issues in the healthcare system, such as premature discharges, insufficient post-discharge planning, and structural defects in patient care. These factors have a direct influence on the quality and safety of patient care (Lejarza et al., 2023). Furthermore, readmissions have a financial impact on both the hospital and the patients because they increase medical costs.
Quality Assessment
Data Collection
As per the data, electronic health records (EHRs) were utilized to collect the information. Electronic health records (EHRs) ensure data accuracy and allow for real-time digital record-keeping. In this scenario, I would utilize a similar process to collect data because they provide structured, real-time data on patient admissions and readmissions.
In-depth data analysis is facilitated by their capacity to decrease human error, ensure data integrity, and allow for seamless integration with other medical systems. Alam et al. (2023) conducted studies that illustrate the reliability and integration potential of EHRs for acquiring medical data. As a result, EHRs play a significant role in valuing patient feedback in healthcare quality assessments.
Data Approach Analysis
The Plan, Do, Study, Act (PDSA) method is advised for analyzing the data that was acquired. The PDSA technique is a systematic, iterative problem-solving strategy that works well in the rapidly changing healthcare business (Sagun & Prudente, 2023). According to Sagun and Prudente (2023), PDSA requires defining specific goals, carrying out interventions, assessing results, and standardizing effective approaches. Given that the PDSA technique is adequate for assessing healthcare quality, I would absolutely utilize it for data analysis using a comparative data analysis approach.
Other methodologies, such as Lean Six Sigma, might also be considered. According to McDermott et al. (2022), the Lean Six Sigma technique is one of the most efficient methods in healthcare quality improvement. It provides a high emphasis on process optimization and variability reduction, which may be effective in addressing healthcare quality challenges.
Benchmark
A valid benchmark should include a comparison of readmission rates within and outside of the state. An example of such a comparison may be the average readmission rate at the state level. Research by Gai et al. (2019) shows the advantages of comparing readmission rates with state level averages. The study states that performance gaps and opportunities for development may be identified by benchmarking (Gai et al., 2019). Furthermore, an institution’s performance may be evaluated in connection to broader healthcare trends by utilizing benchmarks like state or national averages.
Reviewing the readmission trends data from SNHU Hospital revealed that the inpatient readmission rate was 506 over a 30-day period spanning eight months. By dividing the inpatient readmissions by the inpatient eligible discharges, this data was utilized to calculate the unplanned readmission rate, which came out to be 14%. Subsequently, this figure may be contrasted with the information offered by rival hospitals around the state.
Threshold
In order to guarantee service reimbursement, SNHU Hospital must maintain a certain threshold even if its readmission rate is lower than the 15.5% national average. A threshold is a value that is established to indicate the lowest level of performance that an organization can tolerate. There may be fines for very frequent patient readmissions if this criterion is not reached.
Medicare’s readmission threshold is 1%, which means that a facility has more readmissions than expected if its readmission ratio is greater than 1.0, fewer readmissions than expected if its ratio is less than 1.0, and the number of readmissions as expected if it is equal to 1.0 (Health Home Technical Assistance, n.d.). Based on the available data, the readmission rate at SHHU Hospital was determined to be 1.66% lower than the national threshold rate.
Data Analysis
Variations
It is clear from the statistics that the readmission data is based on ratios that the SHNU Hospital’s readmission data for the months of January through August. To compute the ratio, divide the number of thirty-day readmissions for the given disorders that SNHU Hospital anticipates by the total number of projected cases. Common cause risk factors and special cause risk factors are the two types of risk variables that could have an impact on the readmissions statistics at SNHU Hospital.
According to Shaw and Carter (2019), special cause variations are elements that are unexpected and exclusive to a single patient, whereas common cause variations are patterns that naturally occur throughout the healthcare process. It is clear from the data provided about SNHU Hospital that common cause risk factors such medication mistakes or patient noncompliance with medication might be the source of data variability. However, the possibility of special cause variations raises the possibility that specific incidents or modifications to patient referral trends may have had an impact on the data.
Frequency Measures
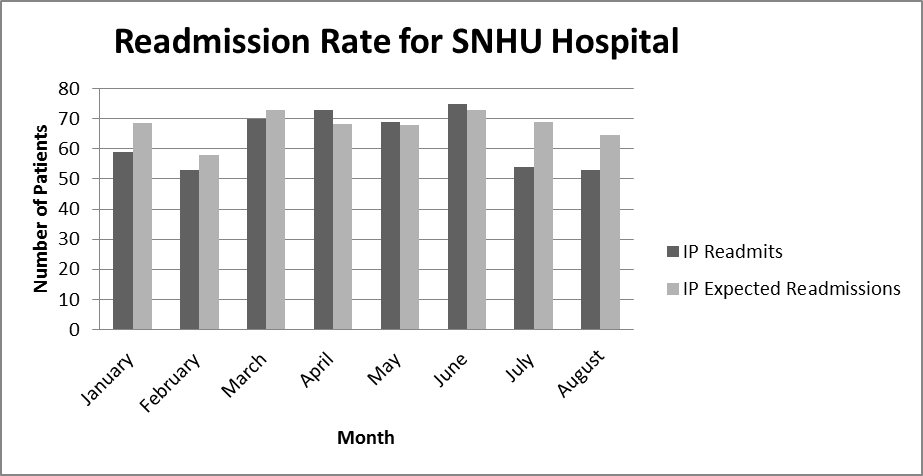
As shown in the above graphic (see Figure 1), the readmission rates for the months of April, May, and June were higher than anticipated out of the eight months of data. According to the statistics, there were 73 readmissions in April compared to the predicted 68.32. The computed readmission rate for May was 69, which is higher than the predicted figure of 67.96. Finally, the readmission rate for June was 75, while the predicted figure for this month was 72.96. For the months where the readmission rate was greater than anticipated, the following table shows the difference between the actual and predicted readmission rates.
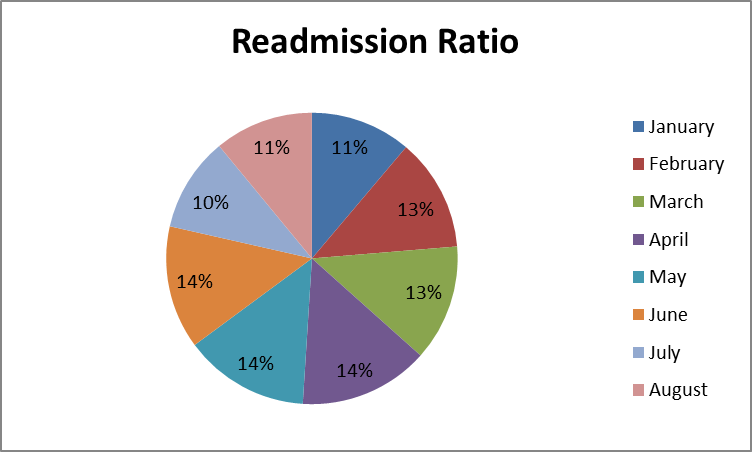
Numerous reasons might account for the differences in readmission rates, as seen in the pie chat above (see Figure 2). Since many health insurance policies have deductibles and out-of-pocket maximum amounts that reset at the beginning of the year, it is possible that overall hospital admissions may be lower in the months of January and February if readmission rates are lower than anticipated. Most people will postpone any elective surgery that would need hospitalization until at least a portion of their deductible or out-of-pocket maximum is satisfied, unless there is an emergency. However, because more people are seeking care for their health difficulties, there were high in April, May, and June.
Potential Risk Factors
There are two types of variables that might affect readmission rates in the healthcare industry: internal and external. The healthcare organization itself is the source of internal influences. According to Fu et al. (2023), internal factors that may impact readmission rates include staffing numbers, staff education, the discharge process, communication within the healthcare team, and the organizational structure as a whole. By addressing the internal causes that contribute to increased readmission rates, SNHU Hospital might improve patient outcomes by better satisfying the needs and goals of its patients.
Given that they are patient-related, external variables influencing SNHU Hospital’s readmission rates may be more difficult to resolve. According to Warchol et al. (2019), external factors such as patient socioeconomic characteristics, cooperation, disease, family support, culture, and transportation may have an impact on SNHU Hospital’s readmission rates. In order to decrease hospital readmissions, SNHU Hospital should continuously assess the quality of care being given and the procedures being followed during the patient’s encounter. This will help to address both internal and external concerns.
Recommendations and Conclusions
Recommendations
- Screening for socioeconomic determinants of health is one way to reduce patient readmissions. Staff and social services might identify patients who are at risk of hospital readmission by evaluating their fall risk and other social determinants of health if an institution used a screening method during the admissions process.
- Enhancing staff communication and streamlining care transitions are two further suggestions to lower the readmission rates at SNHU Hospital. Inadequate communication between caregivers and patients impedes the success of hospital readmission reduction programs and care transitions.
Basic Quality Dashboards
The SNHU Hospital may design a readmission dashboard with important performance metrics as its focal point. Key performance indicators are metrics that a healthcare institution may use to track, examine, and improve all pertinent procedures in order to improve patient outcomes and satisfaction. The number of patients per medical staff member, the kind of admission, and the time of admission are other variables that might be monitored. By monitoring every one of these data points on a dashboard, SNHU Hospital may be able to learn more about the reasons behind readmissions.
Conclusion
Reducing hospital readmissions is a vital goal for all healthcare systems. SNHU Hospital will be well on its way to developing a success blueprint through the use of performance measurements monitored by a healthcare dashboard, a performance improvement technique such as Lean Six Sigma, and a quality evaluation approach. Every healthcare facility should make identifying the causes of poor patient outcomes and hospital readmissions its top priority. Because of this, by concentrating on performance improvement metrics, SNHU would be able to better serve its patients’ needs by concentrating on the tactics and best practices required to lower hospital readmission rates, enhance patient outcomes, and increase financial performance.
References
Alam, S., Bhatia, S., Shuaib, M., Khubrani, M. M., Alfayez, F., Malibari, A. A., & Ahmad, S. (2023). An overview of blockchain and IoT integration for secure and reliable health records monitoring. Sustainability, 15(7), 1-20. Web.
Fu, B. Q., Zhong, C. C., Wong, C. H., Ho, F. F., Nilsen, P., Hung, C. T., Yeoh, E.K., & Chung, V. C. (2023). Barriers and facilitators to implementing interventions for reducing avoidable hospital readmission: systematic review of qualitative studies. International Journal of Health Policy and Management, 12, 7089. Web.
Gai, Y., & Pachamanova, D. (2019). Impact of the Medicare hospital readmissions reduction program on vulnerable populations. BMC Health Services Research, 19(1), 1-15. Web.
Health Home Technical Assistance. (n.d.). Medicaid.gov. Web.
Lejarza, F., Calvert, J., Attwood, M. M., Evans, D., & Mao, Q. (2023). Optimal discharge of patients from intensive care via a data-driven policy learning framework. Operations Research for Health Care, 38. Web.
McDermott, O., Antony, J., Bhat, S., Jayaraman, R., Rosa, A., Marolla, G., & Parida, R. (2022). Lean six sigma in healthcare: A systematic literature review on motivations and benefits. Processes, 10(10), 1-19. Web.
Pugh, J. A., Penney, L., Noël, P. H., Neller, S., Mader, M., Finley, E. P., Lanham, H. J., & Leykum, L. K. (2021). Evidence based processes to prevent readmissions: More is better, a ten-site observational study. BMC Health Services Research, 21(1). Web.
Sagun, R. D., & Prudente, M. (2023). Applying the plan-do-study-act (PDSA) action research model to re-structure the science classroom conforming to the metacognitive orientation standards. Educational Action Research, 31(1), 61-77. Web.
Shaw, P. L., & Carter, D. (2019). Quality and performance improvement in healthcare (7th ed.). AHIMA.
Warchol, S. J., Monestime, J. P., Mayer, R. W., & Chien, W. W. (2019). Strategies to reduce hospital readmission rates in a non-Medicaid-expansion state. Perspectives in Health Information Management, 16(1a), 1-20. Web.