Executive Summary
Information collected and stored by companies is usually used to optimize its production and service delivery procedures to attain desired outcomes. The generation of significant data amounts poses considerable challenges; it adversely impacts the proper management and meaningful use of this information. Big data surpasses the conventionally used storage capacity and the traditional analytical and processing power. Since big data cannot be managed using traditional techniques and approaches, organizations must develop and adopt new strategies for handling it. This paper provides a comprehensive overview of the obstacles encountered by companies when managing big data. It also presents a feasible solution for addressing these challenges as well as recommendations for best practices.
Introduction
Data collection is a crucial corporate practice: companies can utilize this information to optimize their production and service delivery procedures to ensure they attain desired outcomes. The data collected during this process can also be used to predict specific parameters’ current trends and forthcoming events. However, the generation of significant data amounts poses considerable challenges in its management and appropriate use. Batistič and Van der Laken (2018) identify these unmanageable and substantial data amounts as big data. To meet society’s future and present needs, organizations must develop and adopt new strategies for organizing this data and optimizing its meaningful use.
Analysis
Background
Every day, individuals working in various organizations globally generate, replicate, and consume massive data amounts. According to the International Data Corporation (IDC), the digital universe’s estimated size during the year 2005 totaled around 130 exabytes (BB); this value expanded significantly to sixteen zettabytes or 16,000 EB in 2017 (Batistič & Van der Laken, 2018). The phrase digital universe quantitatively elucidates the massive data amounts replicated, created, and utilized within a single year. The IDC further speculated the likelihood of the digital universe expanding to nearly 40,000 EB before 2022 (Batistič &Van der Laken, 2018). These large data amounts comprise big data, typically used by pertinent institutions to develop critical information to generate substantial company revenues.
Big data represents substantial data amounts that cannot be managed effectively using conventional Internet-based programs or software. According to Sivarajah et al. (2017), big data surpasses the conventionally used storage capacity and the traditional analytical and processing power. Big data typically grows in three major dimensions: variety, velocity, and volume as indicated in figure 1 below (“Big data definition,” n.d.).
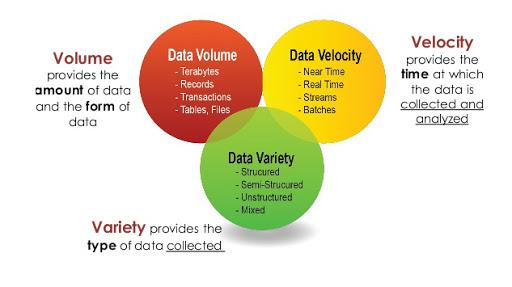
As indicated earlier, the most challenging task associated with big data is its management. Given that this information is unmanageable utilizing conventional software, there is an increasing need for organizations to adopt technically advanced software and applications capable of using cost-efficient and fast high-end computational power to execute such tasks.
Solution
Implementing up-to-date big data analytics tools and artificial intelligence (AI) applications such as machine learning (ML) can facilitate the effective management of big data. Shahbaz et al. (2020) define big data analytics as a complex procedure of evaluating big data to acquire important information, including consumer preferences, market trends, correlations, and hidden patterns that can assist companies in making informed decisions related to their business operations. Big data analytics is an advanced form of analytics; it entails complex applications using elements including what-if analyses, statistical algorithms, and predictive models powered or supported by analytics systems. Data analytics techniques and technologies provide corporations with a practical approach for examining data sets and collecting new insights.
Analytics professionals collect, clean, process, and evaluate growing volumes of different data forms not utilized by conventional analytics and business intelligence platforms. The big data analytics process comprises the data preparation procedure, which consists of four major phases:
- The collection of relevant information from different sources containing unstructured and semi-structured data. Typical data sources include cloud applications, web server logs, texts from consumer survey responses and emails, and machine information captured by sensors linked to the Internet of Things (IoT) (Hariri et al., 2019).
- Data processing: Following the collection and storage of information in a data lake or data warehouse, data experts typically organize, partition, and configure data appropriately for analytical purposes. According to Sivarajah et al. (2017), comprehensive data processing approaches enhance the efficacy and proper selection of analytical tools.
- Data cleansing for quality purposes: this phase involves scrubbing information utilizing enterprise software or scripting tools. Data experts often look for inconsistencies or errors, including formatting mistakes or duplications, and tidy up and organize the data during this process.
- Data analysis: During this phase, data professionals typically gather, process, and clean information using various analytics software, including tools used for:
- Predictive analytics, an approach that facilitates the development of models used to predict consumer deportments and other prospective advancements.
- Data mining, a technique used to sift through different sets of data to identify relationships and patterns.
- Machine learning, a technique that utilizes algorithms to examine substantial data sets.
- Deep learning, a more sophisticated machine learning offshoot.
- Statistical analysis and text mining software.
- Artificial intelligence.
- Data visualization and mainstream BI software.
An Example of Big Data Analytics Tools and Technologies
Hadoop is an example of a big analytics tool used to process distributed vast sets of data across several commodity servers; it functions on various machines simultaneously. It is an open-source model for processing and storing big information sets; it can manage large unstructured and structured data amounts. To process information, one should submit the program and data to Hadoop. Yarn divides or categorizes tasks, MapReduce processes the information, and HDFS stores the information: figure 2 below provides a visual description of this process (“How Hadoop works,” n.d.).
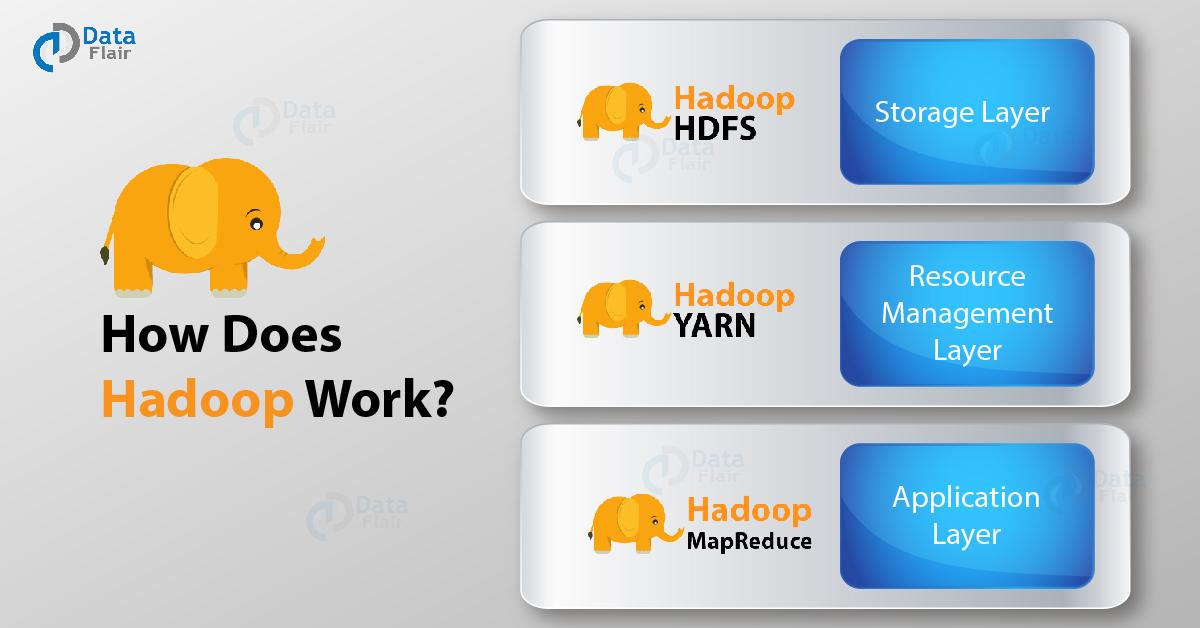
Advantages of the Proposed Solution
There are various benefits associated with using big data analytics techniques within the organization to optimize business processes. First, it facilitates the prompt analysis of large data amounts from different data sources in various formats. Second, big data analytics enhance a company’s decision-making process; it provides corporations with comprehensive data used to make better-informed decisions used to improve their operations, supply chain, and other strategic decision-making areas. Third, big data analytics enhances cost savings which, according to Kruse et al. (2016), could result from the optimization and efficiencies in new business procedures. Fourth, it results in improved and informed risk management approaches derived from massive data samples. Data analytics may also help organizations better understand their consumers’ behaviors and needs, which could, in turn, provide data for product development and generate better marketing insights.
Relevant Theory Related to Disruption
Disruptive innovation refers to an invention that establishes a new marketplace and value network and interrupts a pre-existing value network or market by replacing the highly established and leading firms, products, and alliances. According to Kumaraswamy et al. (2018), Clayton Christensen coined the disruptive innovation theory to elucidate how new marketplace entrants can interrupt well-established businesses. Unconventional inventions are typically more accessible (with regard to their usability or distribution), affordable (from a consumer’s viewpoint), and use business models typified by structural cost advantages compared to their existing rivals within the marketplace. The above-mentioned attributes typify big data analytics techniques and tools.
This technology provides companies with an opportunity to attain a competitive advantage from the created disruption and pre-existing marketplace flux; its existence also abhors a vacuum. Big data innovations, including Hadoop and NoSQL, could be perceived as catalysts of the disruptive innovation; they are associated with significant cost savings and ultra-high scalability and usability (Hariri et al., 2019). Big data serves as a disruptive force in that people and companies require additional skills, tools, and technologies to achieve its associated benefits. They need an open mind to reconsider the process initially adopted in their respective workplaces and transform these operations.
Conclusion
The generation and storage of significant data amounts by companies pose considerable challenges in the management and appropriate use of the information. To address society’s future and present needs, organizations must develop and adopt new strategies for organizing this data and optimizing its meaningful use. Implementing proper big data analytical tools, technologies, and storage techniques, the insights and information obtained from big data can help improve significant business components by making them more efficient and interactive.
Recommendations
Although big data analytics techniques and tools present a feasible and practical approach for resolving issues related to big data management and meaningful use, its implementation may be hindered by various challenges. To address these obstacles and ensure the proper application of big data analytics, organizations should
- Organize big data seminars, workshops, and training programs for all workers handling data frequently.
- Purchase contemporary techniques, including deduplication, tiering, and compression, to facilitate the management of massive data sets.
- Select appropriate data analytics tools; this can be done by hiring experienced data professionals familiar with these technologies or opting for big data consulting.
- Invest in hiring skilled data experts and buy data analytics solutions powered by ML or AI.
- Recruit qualified cybersecurity professionals to safeguard company data. The organization can also implement the following steps to secure critical information: use big data security facilities, including IBM Guardian, actual-time security tracking, access, and identity control procedures, and data segregation and encryption approaches.
References
Batistič, S., & Van der Laken, P. (2018). History, evolution, and future of big data and analytics: A bibliometric analysis of its relationship to performance in organizations. British Journal of Management, 30(2), 229–251. Web.
Big data: Definition, importance, examples & tools. (n.d.). Research Data Alliance. Web.
Hariri, R. H., Fredericks, E. M., & Bowers, K. M. (2019). Uncertainty in big data analytics: Survey, opportunities, and challenges. Journal of Big Data, 6, 1–16. Web.
How Hadoop works internally: Inside Hadoop. (n.d.). Data Flair. Web.
Kruse, C. S., Goswamy, R., Raval, Y., & Marawi, S. (2016). Challenges and opportunities of big data in health care: A systematic review. JMIR Medical Informatics, 4(4), 1–10. Web.
Kumaraswamy, A., Garud, R., & Ansari, S. (2018). Perspectives on disruptive innovations. Journal of Management Studies, 55(7), 1025–1042. Web.
Shahbaz, M., Gao, C., Zhai, L., Shahzad, F., Abbas, A., & Zahid, R. (2020). Investigating the impact of big data analytics on perceived sales performance: The mediating role of customer relationship management capabilities. Complexity, 2020, 1–17. Web.
Sivarajah, U., Kamal, M. M., Irani, Z., & Weerakkody, V. (2017). Critical analysis of big data challenges and analytical methods. Journal of Business Research, 70, 263–286. Web.