This thesis explores the trends, status of big data in airlines and its implications in aviation investment. The paper highlights areas of interest based on these submissions, which include technologies, used cases, applications, products, suppliers, research and development. Within these areas of interest, the researcher categorises point objectives for the exploration.
Objectives of the Thesis
The thesis seeks to:
- Identify the type and amount of data generated and transmitted in aviation.
- Establish how airline data are transmitted and analysed under communication networks.
- Identify the value chain system in aviation.
- Explore research and development projects in aviation.
- Explore data maintenance procedures in aviation.
- Explore big data trends, applications, technologies and used cases.
- Present recommendations for big data in airlines.
Analysing the Objectives of the Study
Objective 1: Technologies, Use Cases, and Applications
The thesis investigates the impact of big data on aircraft. The study objectives have been categorised into specific areas of knowledge. First, the researcher seeks to examine data transmission, its technologies, used cases, and applications. The paper examines data types, processes, data load, data transmission, data architecture and locations under this section. Data transmission have a continuous process in aviation.
The need for sufficient documentation facilitates aircraft efficiency, safety, and maintenance. Data transmission during flight operations can be classified under specific domains. The network architectures relay and classify accumulated data into its operational domain. Data types are classified under an operational domain, priority level, size, security sensitivity, data flow, and phase. These categorisations determine the collective efficiency of flight operations. Data transmissions are coded for maintenance information, flight description, cabin security, or distress information under the operational domain. This information is then coded based on the priority level. Data with high priority clearance are secured and transmitted continuously.
Data mining has been adopted to analyse bytes of information into useful contents for business intelligence. The aviation sector’s integration and globalisation rely on data evaluation (Aktürk et al., 2014; Arikan et al., 2017). Aircrafts armed with flight recording gadgets and devices gather, record, store, and transmit over 400 variables of information. Data gathered and transmitted could be phased interval, elevation level, vertical acceleration, and passenger counts.
According to Marinus and Poppe (2015), airline investors utilise data mining for fuel rates optimisation, weather conditions, passenger investigation, freight improvement, revenue per excursion, gain per excursion, the price per seat or much more comprehensive one catering and managing expenses each chair. Within the following guide, the present uses of data mining in civil aviation industries have been assessed based on specific critical facets such as airports, airlines, freight, passenger, service efficiency, and security. According to Kumar & Zymbler (2019), data mining in aviation has not been deployed as it maintains its early phases of the studies to create knowledge. The knowledge of data mining provides the framework for flight data exchange in airlines.
Flight Data Exchange
Data recording has extensive use in aviation, and investors rely on technology to enhance its use cases. The flight data exchange (FDX) is a messaging tool that provides comparative summaries to indicate regions with a high priority level based on global benchmarking evaluations (Abdelghany et al., 2017; Dou, 2020; Ho-Huu et al., 2018). The program permits airlines to assess flight problems or business trends. Flight data can be operational, business, maintenance, or other related information (Dou, 2020).
Therefore, airlines benchmark their performance against different operators’ aggregate using similar aircraft forms, locations, database, and regions. Therefore, the impact of big data on aviation would advance business investments, global integration mitigates security challenges and enhance cost efficiency. The flight information is gathered from participants and processed from a pre-defined terminal and database. These outcomes are merged into a central database, enabling market drivers, aviation expert, airline regulators, and investors examine the statistics and benchmark against business performance (Dou, 2020). The need for reliable and accurate data management accounts for the use of high-efficiency data recorders.
Consequently, data recording manufacturers highlight the objectives of meeting the requirements of internal stakeholders. These adjustments’ objective is to supply data, which is invaluable in mitigating security dangers and supporting the aviation sector. The platform makes it possible for airline operators to filter information based on the set benchmark, category, and classification forms. Research by FAA reveals that an aircraft generates over 20 terabytes per flight (Badea et al., 2018).
Air traffic controllers cannot utilise most of the information generated during flights because such data coded and unstructured. As a result, big data analytics must be applied to decode and forecast the error encrypting information obtained from flight sensors. Data analyses are evaluated, and errors are identified and corrected where it exists. Airlines gather huge volumes of information such as during flight operations, and such data are relayed to relevant databases for processing and evaluation. Big data analytics make it easier to understand and decipher recorded information for management use.
Hrastovec and Solina (2016) stated that there is a growing demand to move from traditional analysis of predictive frameworks that provide precise and reliable information. Therefore, airline businesses must adopt an integrated perspective of passengers’ data covering distinct points and sources from databases. For example, passenger profile, trade information, shared behaviour, criticisms, and psychographic information are distinct analysis generated from encrypted tones of flight data. These used cases are made simple via advanced technologies of big data applications. Thus, big data makes it simpler to decode unstructured data collections characterised by substantial volume, higher speed, and selection accuracy.
Tones of data are generated from a single flight of an airline. The data volume and its importance have been a growing concern for aviation experts, investors, regulatory agencies, and security experts. The rise in terror attacks has increased the need for quality security architecture to prevent events that trigger attacks and plane highjacks (Lee, 2017; Marinus & Poppe, 2015; Mitroff & Sharpe, 2017; Ni et al., 2019; S. Wang et al., 2018). Consequently, the need for improved necessitated the research on the impact of big data in aviation. Given the need for quality data transmission, aircraft applications provide channels to collate, gather, and generate flight records.
Aircraft applications include maintenance tools, decision apps, performance assessment, fault diagnostics, trend prediction, and reliability analysis. Several sensor units attached to the aircraft enable these applications to generate unstructured data for flight operations. A typical commercial aircraft has smart sensors, strain sensors, vibration sensors, RFID sensors, piezoelectric sensors, and temperature and humidity sensors (Marinus & Poppe, 2015). These sensors relay unstructured data via complementary assess points and gateways to portable devices, cockpit displays and control system. This interface acts as remote servers that transmit communication links to air traffic centre, satellite imaging system, and ground station.
Consequently, data collection systems within the aircraft are categorised into a data acquisition engine and rules. The parallel calculation engine could be SQL, OLAP, predictive analysis, test analysis, search content, graphical presentation, and rule systems. Under storage, the file system that manages large flight data includes HDFS, NoSQL, In-memory database, metadata management, and tenant space management. Data sources for flight recording include QAR, ARCARS reports, reliability data, maintenance manual, design documents, business data, and other related information.
Big Data Technologies and Used Cases
- Data analyses allow the cabin crew and pilot to reinforce their decision-making procedure with accurate information and business intelligence. For example, decoded data generated during flight form the basis for real-time decisions and flight patterns. The computational procedures to optimise flight routes enhance cost minimisation on fuel, reduce air transportation, prevent bad weather flights, and access safer destinations.
- Airlines create enormous quantities of data during online communication, online cost comparison and ticket buying, online check and seat preference, personalisation of supplies, and beverage selection. Most airline businesses use sophisticated algorithms to accumulate and analyse the huge amounts of information made by flight detectors and data sensors attached to each aircraft. These sensors and recording device detectors track flight movements, forecast maintenance, identify errors decrease gas consumption, and re-evaluate turn-around-time at airports.
- Data evaluation as a form of business intelligence has been a formative decision in aviation. The competitive edge of most airlines relies on data analytics. The capacity to analyse quantum data into specific points creates the difference in price regime, promotion packages, customer requirements, travel needs, and quality. An investor gains knowledge by applying informed decisions on flight operations and management. As a result, the management enhances the passenger’s travel experience using data analytics. By implication, investors such as Emirates airlines utilise business intelligence gathered across many touch-points to execute behavioural promotions, product differentiation, customised services and solutions.
- As part of the used cases for flight data applications and evaluation data, recorders ad programs help airlines identify security trends and present information for safety performance indexes. The aggregate review enables operators to verify flight information beyond their restricted databases. Investors or airline management could use benchmarking tools to evaluate the safety performance for international flight routes and security patterns. These applications permit pilots to compare and escalate the decision-making process for unbiased and valid functionality. Data assembly allows collaborative frameworks and partnership among airlines as it offers comprehensive diagnoses when required.
- Predetermined maintenance accepts the collapse pattern of most aircraft is predictable. The PM design presumes that aircraft declines inevitably via a defined order. The deduction varies with other predisposing factors such as aircraft ageing, ecological effects, procedure drift, and intricate communications between components and systems. Surveys on failing behaviour argued that maintenance challenges are caused by arbitrary procedures. Based on these facts, corrective maintenance is prone to ‘inadequate repairs’, while PM triggers ‘over-maintenance’. Thus, big data bridges the gap between CM and PM fault-detection approaches because the technology forecasts future failings based on the aircraft’s health status during routine checks. The distinction between traditional repair approaches and big data induced checks is that signals are triggered by data evaluation and analysis. The principle of predictive maintenance utilises innovations to support a shift from ‘fail and fix’ procedures to a dynamic design that predicts, prevent and fix engine challenges. Based on this understanding, the layers of the existing maintenance framework are summarised. Aircraft maintenance describes a mix of technological, management and supervisory schedules of equipment to retain, recover, or maintain the operation’s status. Therefore, mix approaches sustain the shift from rehabilitative maintenance methods to predictive pattern. Data accumulation during flights provides resources for most predictive maintenance routine. The uses of sensors and other observatory equipment enhance data retrieval processes. For example, a Boeing 787 provides data on equipment status, gas levels, altitude ratings or pressure gauge during flight operations. Big data technologies utilise smart intelligence designs to detect fault lines and hidden signals or anomalies of potential errors to maintain and prevent significant failures and engine collapse. Due to the catastrophic nature of mid-air disturbances, airlines are continuously designed to screen, evaluate, predict, and rate equipment performance. Most manufacturers are adopting a continuous shift in predictive approaches using advanced learning and technology.
- Industries such as financial investment or retail financial, gain from big information analytics in the location of threat administration because the analysis is a crucial element for investments as BD aid in choosing financial investments by analysing the chance of gains versus the likelihood of losses. Additionally, the network sources of BD can be analysed for appraisal of direct threat exposures. Accordingly, BD can benefit companies by allowing the metrology of threats. Performance analytics can be utilised to incorporate the risk profiles handled in isolation across different divisions and threat accounts. The strategy aids risk reduction since a detailed view of risk factors is given to decision-makers.
Additionally, BD tools can manage the rapid growth in network information and lower data challenges by increasing the capability to scale and store investment data. In addition to improving cyber observation and data-intensive remedies, organisations can incorporate streams of data and computerised analyses to protect equipment and workstations against attacks. Fraud detection department deploys BD to resolve sharp practices and detect unauthorised transactions. Analytics are utilised in automated detection. However, companies are harnessing the potentials of data mining capability and artificial intelligence to enhance their systems.
Stream computing describes the handling of enormous data from numerous sources with low latency in real-time. New sources of information producing situations, which include universality of area services, mobile tools, and sensor prevalence, a new paradigm necessitated. It can be related to the high-velocity circulation of information from real-time resources such as IoT, sensors, transaction data and mobile data.
Cargo vehicles could be offered best routes based on real-time web traffic info raising gas efficiency, delivery time, and reduced delivery costs. Investors could harness business profit using the appropriate smart device, which is powered by big data technology. Infrastructure could additionally be equipped with smart sensing units. A recent innovation is smart designs that showed the possibility of developing concrete casting with sensors that give security purposes information. The smart device allows investors to conduct operation within the no-fly wing as the concrete slabs relay movement and body heat to detect unauthorised movement.
Consequently, the smart device generates maintenance data required to sustain the strength integrity of the building. Automation is a significant impact on aviation workforce. Up until now, media attention on this subject has concentrated on concerns that robots and artificial intelligence could displace human intelligence. Nonetheless, a Next-Generation labour force’s production, requiring humans and smart devices, will be a crucial trend.
Developments in robotics, AI and IoT would create safer skies, prevent flight delays and enhance service delivery. An automated workstation supported by an attendant powered by online intelligence could offer cafeteria pointers based on their social media control. The capability of individuals and smart devices to cooperate properly would support the goal of the NextGeneration workforce. Capital funding and synergy with technologies would enhance employees’ ability to provide a spot-on assessment of data retrieved from flight cabins, weather details, and other information.
Data Applications in Airlines
According to Hong and Park, (2019), the capacity to decode encrypted messages during flight operations results in faster implementation in messaging validation. The author stated that efficient programs and data recorders aid quick resolutions of flight challenges. Therefore, big data analytics would enhance faster implementation of fight issues, route changes and a better travel experience. Ben Ahmed et al. (2017) argued that effective and effective data deployment strategies aid smooth communication and better compliance among the flight crew, cabin control towers, and ground control management.
The author stated that bad weather conditions require prompt resolution and guided communications. As a result, big data analytics could reduce the waiting time for faster conflict resolution to prevent traffic jams or poor visual and audio communication. The authors argued that the cabin crew understands flight requirements for standard compliance. Thus, effective standard-compliant systems may require fewer alterations that may affect compliance skills.
A review by De Bruecker et al. (2018) revealed that efficient data transmission systems could adjust operations’ cost. As a result, the predictive maintenance schedule can advert huge airline losses due to engine malfunction. The schedules for prompt aircraft maintenance, reduce operational cost and improve quality service delivery by preventing flight delays and cancellations. Therefore, big data in aircraft could mitigate maintenance challenges, reduce the cost of operations, and aid informed decision-making process. Mitroff and Sharpe (2017) revealed that airlines rely on reliable information for daily flight operations. The author revealed that aircraft data depend on harmonised systems that work with predictable server components.
For example, API, designs restrict unconfirmed machine information. Thus, aviation experts and control tower managers must comply with data interrogation standards to avoid human errors. Big data removes human complacency due to ineffective data interrogation by decoding and verify the level of reliability after processing flight-encrypted information. These advanced technologies enhance flight operations and communication reliability.
As stated earlier, huge volumes of data are transmitted during flight operations. The encrypted and unstructured data are processed based on the airline’s data transmission channel via an open-ended connection. For example, data transmission encryption includes the aircraft’s location, engine information, flight gauge, and aircraft health status. After logging and transmission, information from data centres is routed offline. The flight information is evaluated and examined by the airline’s data centre and conclusive results sent to the cabin crew (Nikolopoulos & Petropoulos, 2018; Park & Pan, 2018; S. Wang et al., 2018).
The database centre transmits data retrieval information and recommendations to the airport control towers for periodic aircraft maintenance. To offer the aircraft precise and accurate maintenance, the aircraft’s flight information and other usable variables form the basis and source of information for flight configurations. According to Dubey et al. (2018), big data analytics support SAP predictive repairs. Aircraft maintenance is controlled to guarantee safe flight performance.
The International Civil Aviation Organization has organised national regulations according to global standards (Sun et al., 2018). Aviation Data Exchange programs’ launching opened the door to information sharing using a reliable third party to acquire accumulated statistical evaluation of the business performance via benchmarking applications to enhance safety standards (Arikan et al., 2017). ASIAS was established to enhance aviation security and operational efficiency. The FAA empowered the ASIAS system to track flight risks, identify movements, suggest mitigation activities, and confirm the effect of its execution (Badea et al., 2018). To guarantee the security of patented information, procedural data are de-identified to avoid infringement. These data exchange systems provide enabling channels for safer and efficient data transmission.
Data4Safety is another exchange system that contains IT infrastructure, which will allow the information sharing process, such as information assemblage, storage, processing, and security. In the execution stage, the exchange system enhances security via mining strategies. The impact of distinct data serving systems has been pivotal in enhancing safer flight operations (Bertsimas & Gupta, 2016; Bogicevic et al., 2017; Marinus & Poppe, 2015).
The GADM is an aviation management program that provides reliable geographical data for airline operators. According to Anderson (2019), over 92 per cent of IATA members have consented to share information from over 500 organisations. The program permits data providers to code and decodes reports on security metrics and trends, such as analyses on events, operational intelligence and ground maintenance document. These programs foster a model change in security evaluation, shifting from a responsive strategy based on investigating incidents to a pre-emptive strategy based on data evaluation.
The aviation PHM programs assemble distinct data from aviation companies, airports, aircraft, spare parts warehouse, fix mill, maintenance centres, overhaul foundation, and other relevant sources. The data are recorded and transmitted as a ground data reference to terminal databases for pre-emptive and predictive evaluation (Arikan et al., 2017; Atkinson et al., 2016; Benlic, 2018; Bertsimas & Gupta, 2016). The program relies on the cloud-computing host, as it utilises data mining to acquire knowledge and needs of various aircraft. Based on its architecture, the program offers support for aviation, government agencies, and investors.
The PHM centre provides integrated solutions using large data exploration to comprehend the efficient utilisation of reliable information and enhance aviation investments’ performance efficiency. The program utilises predictive programs to improve repair services. Sternberg et al. 2017 reviewed several flight prediction procedures and identified distinct designs to evaluate their strengths, flaws, and performance. The authors found that machine-learning strategies showed substantial growth in the past ten years. The findings showed that the system transmits data to several regression models using the recurrent neural network (RNN).
A study by Khanmohammadi et al. (2014) demonstrates that data collection during flights is the bedrock for a sustainable aviation market. The author argued that big data technology would enhance data learning and mitigate system challenges caused by human error. Thus, big data would enhance data evaluation and accurate readings in aircraft management. The need for big data architecture has been overdue, and its impact on flight operations is strategic and effective.
An airliner avionics program is an incorporated design with multiple backup units. The backup units are called the central control computers (CCC). The system is designed to retrieve tons of data from the aircraft via sensor gadgets. The sensor recorders provide data for flight navigation, communication relay, command calculations, and video signals. The system is designed to mitigate data failures of input uncertainty.
As a result, the backup systems resume play when the master device collapses. This integrated structure impacts and controls the FDR structure. As the information is processed at central control computers, the developer makes an exchange evaluation of the CCC recording form. According to Hong and Park (2019), big data deployment in multi-source operational systems promotes the air transportation system. An aircraft is a complex systems design to move above gravity and strong weather presence. An aircraft is designed to operate manually and automatically, and it is an assembly of many different components and subsystems that complements the function of several related parts.
A commercial aircraft’s main components include the fuselage, wings, empennage, power plant, and the landing gear. These components are designed to collate data for its operations and functionality. Given the transmission of data tones, an aircraft generates information and relays composite data to the ground control tower for informed decision-making. A Boeing 787 Dreamliner aircraft has several functional parts designed to transmit specific data.
A Boeing 787 Dreamliner has its avionics system, flight control systems, landing gear system, furnishing system, environment control unit, anti-ice unit, engines, auxiliary power compartment, wheels brakes, engines starting systems, electric systems, hydraulic system, and pneumatic system. The complementary part of an airline subsystem includes the navigation unit, instrumentation and recording, vacuum system, safety compartment, fire protection unit, communication and electrical system (Blasch et al., 2015; Cimmino et al., 2020; Dubey et al., 2018).
As stated earlier, these systems rely on data transmission from one data bus to another. The pilot and crew utilise these data transmission to understand the engine’s health status, navigation systems, and the environment. The mechanical components include fuel and hydraulics, safety systems, flight control unit, armament and escape unit, anti-icing unit, parachute systems, landing gear and environmental control unit.
Aircraft Systems and Subsystems
The ECU enhances the travel experience of the passengers, animal, and other cargoes. The ECU regulates the airline’s ozone content, control emergency oxygen within the aircraft, humidity, temperature, and pressure. Given the ECU’s nature and function, it could be designed as a vapour cycle systems or air cycle unit. A life support system is an aircraft component that regulates oxygen, pressure gauge and other toxic constituents. Based on these assumptions, aircraft stores liquid oxygen, gaseous oxygen chemical and on-board oxygen systems. Information transfer guides the unit regulation from one component to another. The pilot relies on a multiform network that relays information from the airline’s body sensors. These sensors collect, stores, and records different unstructured data for interpretation.
The anti-ice unit regulates the movement and position of an aircraft. The system provides resistance against ice formation on the airline’s probes, radar unit, and other components. Data communication writing the aircraft sends command information to each part of the ice protection unit (Cimmino et al., 2020). As a result, the thermal system relates to each component’s temperature level against the regulated benchmark. When the systems receive a distress signal, it performances routine maintenance by triggering backup activations to remove or defrost a certain part. These systems prevent ice formation on fuel lids, cabin decks, and landing gears.
The Aircraft Hydraulic System (AHS) includes the airline’s landing brakes, gear and flight control panels. AHS is a major component that guarantees flight safety. As a result, sensitive data are retrieved from the aircraft’s body sensors and relayed to other parts that trigger heat exchanges, pump activations, and oil treatment. For example, an aircraft’s operating pressure varies between 3500 – 8500 psi. The AHS controls the psi level based on data projection from each related sensor points. The aircraft fuel system (FS) regulates the fuel movement’s pump pressure within the engine component.
The FS relays valuable information about fuel consumption, engine combustion, and related components. The fuel tank is fitted with body sensors to collect information regarding fuel transmission, cockpit display, and engine temperature and fuel gauge. The continuous monitoring proves that aircraft data in the huge volume are transmitted between systems and ground control towers. Based on each aircraft’s design, body sensors are fitted in fuel tanks, pumps, and fuel valves. As explored in the thesis, airline data systems and subsystems would be inefficient where systems attacks and data theft are not controlled and prevented. Therefore, it is a growing concern that big data could fuel data confidentiality challenges and security issues.
Data Confidentiality and Security Breach
Big data management could create security challenges in aviation and other sectors. A survey by Dubey et al. (2018) observes that air transportation is a sensitive sector that requires confidential data approaches. The authors note that security issues go beyond data theft to modern business vulnerabilities. The authors argue that fake data generation is a concern for aviation experts. Consequently, access to authorised flight data records makes hinder the advancement of big data in aviation. The cost of conducting security audits to prevent data theft is expensive, and investors may resort to traditional data processing channels as an alternative.
The use of distributed frameworks creates avenues for data theft. As a result, most organisations are unwilling to share confidential information with other communities. Watson (2019) introduced safety problems with large information and recommended ways of averting safety risks. The safety issue inherent in large data incorporates source collection points and cloud management. The design for data transmission makes it challenging to close vulnerable source points. As a result, data breaches influence numerous businesses and human safety. The paper recommends data tracking via end-to-end encryption to prevent unauthorised data access during flight operations.
The paper recommends that data investors verify to avoid unqualified personnel. Data encryption slows large volume transfers from source points. As a result, data transfers are sometimes not encrypted during transmission (Ben Ahmed et al., 2017; Benlic, 2018). Although such information is unstructured and coded, its access by unauthorised individuals could be risky in aviation. Consequently, large volumes of data require time for investigation.
Therefore, such a scenario creates intrusion detection difficulties since the machine occupied times are extended. Although security-tracking methods enhance information protection, breach detection remains difficult even for remote-controlled systems (Adacher et al., 2017; Dubey et al., 2019; Hrastovec & Solina, 2016; Kumar & Zymbler, 2019; Pinto Leite & Voskuijl, 2020; Santos et al., 2017). Thus, aviation experts and investors must encourage continuous tracking to acquire accurate situational awareness. The strategy would prevent false alerts and streamline intrusion detection.
Data monitoring, Control and Regulation
The first step with flight operation is a tracking feature that identifies the system and observes state by gathering, fusing, and evaluating information from various sensing units. The extent and accuracy of the surveillance systems are associated with the completeness and high quality of the data available for the control centre to observe and establish its system state. System monitoring difficulties consist of identifying, defining, and gathering information for the designated scope. While some exact and timely information resources exist, especially for commercial transportations, not all operational criteria are observed in a prompt manner.
The aircraft components may not be monitored completely, or only with substantial effort and cost. In contrast, others are not observed because of secrecy or other concerns. The information from different sources varies in quality. Data quality may likewise differ with time as sensing units or system conditions transform. Correlation and the fusion of information resources can be difficult due to differences in factors, such as latency, for information gathered from various resources (Badea et al., 2018; Sun et al., 2018). Data resources need to be assessed and checked for quality and then merged to make it possible for monitoring that satisfies the ground control system’s requirements at a price regular with the value that the information gives.
Successful and reliable monitoring and controlling of flight data requires accurate identification, characterisation, recording, and recovery of the needed information based on availability, efficiency, quality, and price considerations. Fulfilling flight standards’ needs and requirements will be a challenging due to data inaccessibility or cannot be merged while structure or conflict of interest is observed. Consequently, most flight data are not stored or retrievable.
Effective monitoring is expensive and could be burdened by access and usage restraints because of privacy problems. According to Li et al. (2017), data monitoring and controlling are challenging because flight data is sometimes not regularly available with quality, particularly information accuracy and suitability. While information retrieval equipment purchase is a general-purpose concern for flight investors, the cost of advanced architecture may hinder quality systems. While some space procedures give telemetry information, most do not. While they might be surveyed by the Department of Protection (DoD) radar systems, those details are not presently offered to the FAA for air traffic control objectives.
Specifications and information streams require functional information reporting and storage. Therefore, big data would complement existing communication infrastructure by providing advanced capability via data mining initiatives. In actual time, the methods for information collection include wireless links from aeroplane cabins to satellite-based systems or ground networks. Effective monitoring systems utilise aircraft communications architecture.
According to SME (2015), big data analytics would enhance flight-to-flight communications via wireless links. As a result, data monitoring and control can be revised using close group contacts against ground control centres. In cases where geolocations and radar visibility are lost, a close group or flight could become a network link to reactivate lost and broken transmission. Thus, big data could bridge the gap for aircraft communication. Key elements relating to collecting data from each of these resources consist of availability, latency, upgrade rates, integrity, protection, styles, avionics requirements, application, solution prices, range policy, and bandwidth utilisation.
Successful monitoring and control of systems will efficiently spot the elevated threat and lower false alarm rates. This can be challenging to attain, given that an IASMS will certainly attempt to identify and assess the threat of uncommon events, which is difficult unless the high quality of input information can meet the demands of a CONOPS (SME, 2015). Effective data management improves information flow, flight preparation data, aeroplane settings, airport status, course standing, and pre-departure information.
Flight placement information comes either from route radar or from nautical setting reports. Information on aircraft speed and elevation comes from on-board sensors and varies in quality, relying on the on-board altitude dimension system’s accuracy (Abdelghany et al., 2017; Bertsimas et al., 2014; Dou, 2020; Lališ et al., 2018; Lee, 2017; Ni et al., 2019; Rios Insua et al., 2018; Santos et al., 2017; S. Wang et al., 2018). Some information provided differs among airlines because of the variation in data gathering and recording structures. Future improvements to the traffic monitoring system will consist of position records created by the ADS-B structure. Such improvements would boost the precision, dependability and data reliability.
The resources and data quality provided by an aircraft must be comprehended and tracked to be accurate and valid. Furthermore, some data feeds must be retrievable to trigger continuous assess data and identify threat signals. Data storage space and retrieval capabilities enhance high-quality data analyses (Czerny et al., 2017; Dalmau & Prats, 2017; Mitroff & Sharpe, 2017; Rios Insua et al., 2018). Such input could identify when a particular information resource is accurate, misleading, and unreliable. There is a value proposition associated with choosing information for structure surveillance. Some data resources are easily available in genuine time as well as easy to accessibility. The assumption is true were flight data are retrieved through SWIM channels.
In other instances, data accessibility from the control centre may be unreachable. Aircraft placement and speed information are approximated, yet specifications that may be of value may be unrecognisable and encrypted. Trajectory intent is challenging because pilots sometimes do not follow a routine flight plan. As a result, ground controllers may clear a pilot to make personal decisions when the weather status is uncertain and ambiguous. To achieve effective data monitoring, airline operators must fuse information from supplementary sources, such as from ADS-B records or voice acknowledgement of the communication chain.
The capacity for providing sufficient and prompt information is challenging due to privacy fears. The information required to execute safety investigation are related to the activities and competence of the pilot, controller and other participants of the aviation sector. People will certainly hesitate to disclose efficiency information that might jeopardise their income, business evaluations, or break individual privacy.
Data generated by exclusive business and airline companies, including information on their personnel’s performance, will be tough becaus of patent rights. To mitigate privacy problems, enforcement actions, and the FFA has obtained responsibility regulations to secure public disclosure details given under an authorised program. This approach has been successful in generating security data and maintaining privacy. Without such legal securities and trust, valuable security detail might not be available for evaluation, which could restrict the complete capacity to improve flight coordination and enhance passenger safety.
Regulation is a set of procedures enforced and empowered by the authority. Control is an authorised process triggered by the capacity to manage operations. Air transport aircraft count on advanced trip computer systems to secure the aircraft and enhance passengers’ travel experience. As a result, airline operations are governed by computational regulations, which designate flight control modes. Commercial airlines with fly-by-wire flight controls call for computer system regulated flight control settings that provide the aircraft’s functional setting. An inefficient computational tool or the failure of multiple systems causes errors with digital flight control.
Digital flight control systems (EFCS) give augmentation because it enhances engine safety from overstressing by fixing, recognising disturbance, and supplying a yaw damper. In some aeroplanes, control is attained with the pilot’s control column, rudder pedals, trim wheel, or throttles that mechanically move cables, pulleys, or hydraulic servo shutoffs, which in turn relocate control surfaces or alter engine settings. These aeroplanes have flight control computers, which send out digital signals to operate control surface areas or engine controls, educate the flight crew, and offer performance information. Aeroplane manufacturers and designers developed a set of trip control settings to prevent system shock and collapse.
Under this design, pilots regulate the aircraft with the fly by wire protections and manage faulty navigation tools. Restricted mechanical control settings are infused to enable continuous aircraft control throughout the reset process complying with a short-term collapse of flight navigation systems. Flight control regulations permit pilots and ground control centre to assess the aeroplane’s performance under numerous conditions when the navigation systems fail partially or completely loses its working status.
Developers construct the capacity to by-pass the computer systems or activate the auxiliary navigations parameters for safe landing and operations. Thus, the deployment of data mining concepts of visualisation, statistical analysis, and machine learning enables airlines to manage huge data challenges (Oussous et al., 2018). The authors presented the issues in implementing analytical alternatives, such as machine learning, incremental strategies, and granular computing. Chen et al. (2014) examined large data programs and analysed methods of managing huge data challenges. The authors conclude that big data enhances could computing, data visualisation and quantum computing structures.
Thus, big data in aircraft would improve the customer’s travel experience and sustain the aviation sector. Wang et al. (2016) reviewed big data analytics concepts and recommended a shift in four categories. The authors categorised big data features into processing paradigms, decision-making data, software platforms for data presentation and BD opportunities. The authors argued that these classes would enhance BD study in aircraft. The findings highlight technical challenges like crowdsourcing, and divergence, re-engineering use, and data scaling. Thus, the literature review findings are consistent with the thesis objective that big data technologies would improve data access, which is challenging for aviation experts.
Summary for Objective 1
The paper finds that big data provides the platform for effective and productive operations. The results and observations from several literature works prove that huge tons of data are generated from a single flight operation. Consequently, data transmission and safety is the bedrock of aviation sustainability. The impact of big data via used cases, data applications and transmission is enhanced by an effective communication network. The study’s second objective explores communication networks and their impact on data flow, data confidentiality, and security attacks.
Communication Network and Standards in Aviation
Air-to-ground communication utilises specific protocols to provide a two-way network for a simplified message and data transmission. Network communication requirements describe the based standard practice for interaction (Khuwaja et al., 2018). When a pilot collects data from devices installed on the aircraft, they relay these messages via specific links for further instructions. The communication triangle provides smooth operations and quality travel experience.
The aviation standard communication requirement includes voice communication, data communication and visual links. Under a voice communication network, commercial airlines utilise VHF links, UHF, HF, and SATCOM (Cao et al., 2018; Khuwaja et al., 2018; Rusek et al., 2016; Zeng et al., 2019). Data communication networks are enabled with CPDLC, mode S, transponders, sensors units, ADS-B or aircraft communication, addressing and reporting systems (ACDRS). Visual communication links are enabled with Aldis Lamp. These standards are mandatory for flight operations. The combination of these communication standards depends on the carrier or manufacturer’s design. Given the advancement in big data technology, aircraft designers have modified systems communication links to enhance data transmission, message clarity and secured links.
Flight communication networks rely on automated architecture to provide data transmission demands. Whether ground centre, control tower or another network hub, communication network systems must transmit, track and exchange data between various tools or individuals within a system. Interaction networks give various benefits over traditional designs that provide point-to-point lines that enable only a few participants to connect (Ge & Han, 2017). Microelectronics’ breakthroughs have decreased the dimension and weight of the cordless network equipment, allowing the expedition of methods to deploy cordless facilities.
There has been enhancing passion in airborne communication networks as shown by research and market efforts. Various use cases have been envisioned for aerial network deployment, including public security, to give employees protection and service capacity throughout an emergency, flight events, and Web connectivity. Several aviation experts have released findings on the opportunity of using aerial systems for supplying cordless or solutions. As a result, most flight designers are exploring the prospect of convenient big data architecture for the enhanced travel experience. The need for efficient flight data exchange has been a growing concern, as reported in several works of literature (Amare et al., 2018; Dou, 2020; Ge & Han, 2017; Haferkorn, 2018; Z. Wang et al., 2018; L. Zhang, 2020).
Network collapse, data challenges, transmission error, communication inefficiency summarise the need for big data in aircraft. Consequently, data generated in huge volumes have been challenging for ground personnel, flight experts, pilots, and investors. A survey by Cao et al. (2018) revealed that data exchange during flight operations is critical to aircraft safety. However, communication interruptions, database collapse, or system reboot characterise flight operations. The need to provide effective transmission, and decoding architecture against uncertainties is required. These sections seek to discuss data communication architecture, network systems, communication topologies, data exchange standards and performance parameters.
Air traffic communication systems use wide ranges of wireless innovations during various trip phases. From a theoretical point of view, all of them are unconfident as security was never part of their layout. The advancement of cordless protection in aviation did not update the technology. Current contributions from academic studies have manipulated this intrinsic vulnerability and demonstrated attacks on some of these communication technologies. These surveys disclosed considerable inconsistency between the safety and security perspective in aviation. Air traffic control comprises modern technologies, which sustain flight traffic solutions.
ATC system consists of communication web links between pilots, controllers, and innovations for checking traffic (Cao et al., 2018). Information solutions and communication networks supply information to pilots to boost their situational understanding and enhance passengers’ flight experience. The standard requirement for communication in airlines would be explored to understand the processes of data transmission.
Communication Network Components
Air Traffic Control (ATC) procedures allow communication between controllers, pilots, ground communication centres, aviation regulators or aircraft. ATC designs develop information regarding the aircraft’s position, intent, and therefore ensure flight security. Trip information services, weather condition reports, and airport terminal data broadcasts can likewise be offered by voice interaction. The system provides operational interaction between the airline flight crew and the aircraft, as long as the aircraft is in an array of the operator’s transmitter. Voice communication is carried out by analogue radio on VHF and HF.
The voice communication equipment links the pilot and areophane. Controller Pilot Data Link Communications (CPDLC) is a message-based service providing a choice to voice communication (Gurtov et al., 2018). The alternative mitigates communication challenges with VHF architecture. VHF depends on the controller to capture a wrongly understood flight degree direction while navigating other aircraft or communication network. Surveillance Radar (PSR) is the phrase for non-cooperative aircraft localisation systems. The communication architecture uses radar for effective message transmission (Fas-Millán & Pastor, 2019; Gurtov et al., 2018).
PSR is independent of an airline’s devices but depends on the location properties such as surface product and size, distance and alignment of the aircraft in space. As analogue signals bring the received information, the system must deal with various troubling echoes created by terrain, obstacles, weather, groups of birds, or even vehicles. Thus, a PSR detects non-cooperative communication hub, transponders, and other network technologies driven by radar connection. Most aircraft have secondary security radar (SSR) that provides target details on ATC radar displays contrasted to PSR, which just supplies unknown target placement without additional supporting information (Santos et al., 2017; Si et al., 2021). SSR network centres broadcast investigations of aircraft transponders, which respond with the preferred info. SSR uses electronic messages with various regularities and modulations for the interrogation.
Consequently, the ADS-B architecture transmits aircraft identification properties, setting, speed, intent, or codes. These programs generate millisecond data amounting to tones of data daily. The transmissions support informed decision making for flight operations. The architecture ADB-S improves location accuracy and reduction system expenses by replacing existing radar systems. Many airline investors have adopted standards for deployment; however, such systems are limited (Gurtov et al., 2018).
ADS-B’s relevance is critical to aviation sustainability, and its integration with SSR systems is a developing concept for academic learning. Performance-Based Navigation (PBN) defines the area navigating (RNAV) systems, precision, and safety levels. PBN is a structure that specifies navigation performance spec to comply with defined functional efficiency requirements (Song et al., 2021). PBN CONOPS offers the basis for the design and execution of computerised flight routes.
Consequently, performance-based navigation defines airspace design and impediment removal. It likewise facilitates ways to transmit the efficiency and functioning capabilities to use specific flight routes. Once the performance status is recognised based upon functional requirements, the aircraft ability determines the efficiency to be fit and licensed for the procedure. Efficiency demands are recognised in aircraft navigation requirements, which also determine the option of navigating sensing units and tools that may be utilised based on performance needs. The tools and electronic architecture for PBN requirements is supported by system performance.
The RTSP is the standards expressed as efficiency parameters to supply the accepted RASP defined for certain airspace. PBN framework allows the safer layout by setting apart air traffic within flight terminals. PBN concept boosts sequencing efficiency, creates space, and merges traffic routes combined with communication links. The systems reduce aberration between separation procedures, minimises text-based communication, enhance predictability and gateway administration (W. Chen et al., 2021; W. T. Li et al., 2021; Song et al., 2021).
Flight efficiency monitoring or forewarning proficiencies rely on the system setup, style and configurations. Consequently, performance-based navigation navigates system performance, track system efficiency, reveal unfulfilled RNP demands, and track deviation displays. Communication architectures provide the platform for data integration, transmission and analysis. These platforms provide the basis for big data integration in aviation. The research gap explores communication topologies, communication requirements, and standard practice with big data in aircraft.
Flight Data Aggregation and Processing
VHF radio wave is a typical form of interaction in aviation and cover over 96 per cent communication links with ATC systems. The transferring terminal sends out a signal via a straight line in streamlined terms and is picked up by the locating terminal.
VHF communication links offer clear voice communications from the cockpit to other terminals of operations (Xu & Kumar, 2015). As the radio wave signals travel in straight lines, the earth’s curves’ limitations obstruct data communication and data transmission. As a result, data links and message transfers are disrupted at very high altitudes such as hillsides and mountains. Space, which a VHF wavelength travels, depends on several contributing factors. However, closer ranges produce clear communication interpretations than farther locations. Due to disruptions, aircraft are designed with multiple VHL signals to backup when one communication links collapse.
The categories of VHF signals include VHF-L, VHF-R, and VHF-C. VHF-L aid interactions with the energetic ATC regularity, while VHF-C controls datalink between air transponders and ground control centres (Gurtov et al., 2018; Khuwaja et al., 2018). The communication links allow multiple frequencies to avoid detection, mitigate unauthorised tapping and overcome poor receptions (Gao et al., 2018). The VHF-R maintains a listening watch on the emergency regularity or frequency. VHF radios provide quality communication and message transmission over comms satellite towers. However, network navigation is poor when the airline flies over the mountains and ocean.
At such points, aircraft designers installed the HF communication network. Although HF wavelengths are not efficient as VHF signals, the transmitting radio waves operate under high altitudes and over water lands. The wavelength under HF signals escapes the ionosphere to enhance radio signals for effective communication. As a result, pilots push the HF activation button when the VHF signal is lost. The CPDLC is an alternative data communication link during flight. CPDLC is a data communication link that permits without voice radio frequencies. When the aircraft leaves radio frequencies coverages, the CPDLC system activates satellites links for effective commination with ATC navigation tools. Satellite interactions encourage voice communication using the INMARSAT system.
Air transportation relies on data communication to provide safe traveller experience. The need for effective data transmission is a growing concern for investors, aviation experts and passengers. Aircraft communication systems provide continuous data transmission for the pilot crew, ground control centre, and other flight data analysts. The basic communication components include integrating audio systems, communication devices, sensors units, static dischargers, cockpit intelligent systems and voice recorders (Khuwaja et al., 2018).
Recent events have underscored the need for constant, premium interactions between aircraft in flight and the ground for air traffic monitoring and ground-based surveillance of aircraft systems. Nevertheless, aeronautics communications have been restricted to relatively bad quality voice links for the pilots and air traffic controllers when operating over waters or remote terrestrial areas. The communication network is usually unstable and challenging. Additionally, no centres have existed for customers or passengers interactions solutions. The need for big data in aircraft would solve these pending challenges and mitigate the effect of poor quality communication links for effective decision making during turbulent and normal flight operations. Communication and data efficiency has been progressive with advanced transmission networks and topologies, satellite feeds and web links.
Data Monitoring Standard
In commercial aircraft, the aircraft monitoring system consists of a safety-critical system and non-safety systems. The progression attained in fixed sensors and cordless data communication has extended surveillance ability of aerial structures, spacecraft and ground screening architecture. The AWSN enhances safety, security, and system adaptability due to less complex fault appropriations refining and fire threats on the wiring system.
Depending on domestic communication centres, data handling, cordless interaction, and a cordless piezoelectric node can in your area, improve data analysis and processing of real-time statistics. Aircraft monitoring systems include structures health surveillance and cockpit observation. The AMS accumulates information from various sensing units deployed on aircraft structures, mounted inside the aircraft cabin, carrying out structural health and wellness monitoring and cockpit observation (Gurtov et al., 2018).
With the development of digitised equipment, sensors and data systems have become complex. The airline’s structure health surveillance, optical fibre-based worldwide condition assumption, and multi-sensor innovation offer strategies for analysing aircraft’s challenges and guarantees complex frameworks’ safety. Specifically, structural feedback is acquired via tension, pulsation and ultrasound sensor tracking on aircraft frameworks. The response is utilised to assess engine status, wind velocity, and evaluate the plane framework’s residual life. Communication frameworks enhance warning signal and status management.
Communication networks are designed to transmit messages and signals from one node to another. The aerial radio communication sensor support aircraft surveillance. A typical node has three or four subsystems that regulate and gathers data codes for analysis. Communication experts designed these unique nodes to have sensing area, computational unit, cordless transceiver, and user interface. The computation core is the key distinction between the wireless sensing unit and the traditional design. A microcontroller unit (MCU) visibility permits on-board information handling, information storage, and interaction.
Data generated from the sensors units and executable program are implanted in the RAM or ROM compartment to accomplish these tasks. The size of internal information bus for microcontroller varies with classification, identity, processing capacity and energy usage. Several memory sizes and used formulas are commercially offered, which are customised in conformity with the particular tracking activity. Based on the used cases, communication network and topologies are designed to generate huge tons of data (Chang et al., 2020; R. Chen et al., 2020).
The sensor communication node transforms the analogue data to automated messages by microelectronic devices. Some generally used sensing units include pressure sensors, temperature units, and piezoelectric sensors. The node sensors incorporate more than one tracking components, while others include one sensor for precision. The deployment of RF spectrum permits node integrations in a communication network. The node interaction aids the sensor units to collate data from individual components. The user interface is a DAC that transforms electronic data to analogue voltage output for active sensing activity.
Impact of Communication Principles and Standards
The precision of flight data is a critical quality element. Data precision describes an airline’s prognostics and wellness management, such as fault discovery, error seclusion, error prognosis, and diagnosis. Wireless sensor networks have been deployed in equipment stations, ground control centres, industrial environment surveillance, and factories for reduced latency wireless communication establishing an instance for the AWSN. Communications between cordless nodes call for latency to improve efficiency. Thus, aviation’s effective communication standards enhance real-time data accuracy, information reliability, and informed decision-making.
The wireless airborne gadgets transmit vital aircraft information to data aggregation. The cordless airborne tools sometimes is interfered by extreme atmosphere and RF signals, which violate radio signal frequency. Security devices monitor aircraft movements in the airspace. Communication gadgets complete the two-way audio function and signal interaction between aircraft and ground control tower, which aids ground communication, flight interactions and long-distance communication.
Literature reviews on communication systems and network requirements for ZigBee wireless sensing unit networks focused on utilising existing cordless systems. The articles cited wireless chained ZigBee repeaters and numerous identical transmission streams of the same data. These mechanisms offer the standard tools for developing safe communication network topologies. Grekhov et al. (2020) noted that redundant networks transmitters had demonstrated fault-resistance capabilities using fault detection and recuperation systems with interference and network re-join with modified node functions. The author concludes that these techniques have led to more trustworthy systems. Wireless avionics and user interfaces of navigation have triggered the shift from wired networks to cordless communication capabilities (Ge & Han, 2017).
As a result, communication experts designed experimental efficiency analysis to analyse network dependability and recognise the likelihood of unavoidable system collapse. Such testing is restricted to specific events and environmental conditions. The network model focuses on inter-component communication instead of protocol behaviour. It enables the contrast of different networks based on data safety, security, and scalability. Algorithms and methods for searchable security have been examined in cryptography. The primary emphasis has been efficiency and protection formalisation.
These studies required exact key phrase search and its findings prove impractical. Airline communication has been analysed in three perspectives: marine vessels, airline cabin, and air traffic control. Instead of focusing on an individual’s inner cognitive procedure, researchers focus on cognitive activity between individuals and technical systems (Ge & Han, 2017; Zheng et al., 2018). The analysis or the impact of a message on the receiver depends upon what the receiver recognises. In other words, that pilot’s experience in practice determines his or her communication capacity.
Therefore, the standards of the communication network are independent of the pilot’s experience or specialisation. Experience is considered crucial to proficiency, which is built throughout the program of a profession course. Some flight workers are better at detecting system errors due to personal experience with navigation and communication tools. Tasks performance comprises a context for learning and the build-up of expertise in practice areas (Köroğlu & Eceral, 2017; Kim, 2018). Gurtov et al. (2018) revealed what occurs in the airline cockpit as a dispersed cognition system. Drawing on audio and video data records, the authors investigated how data and communication systems are propagated during flight operations. The communication triangle includes pilots, cockpit systems, sensors nodes, audio controllers, ground control centres, and audio and video com-links.
According to Keller et al. (2020), data generated during flight operations or communication messages are made possible via standard transmission links. The dedicated network describes the interaction network integrated with departments, units, or systems to satisfy monitoring, safety, and security scheduling. Expert administration has been developed for massive dedicated networks, and small-scale systems are depends on auxiliary supervision.
The air traffic networks are categorised as an exclusive communication network, consisting of partial self-built nodes. The consistency of the broadcast link reflects the degree of transmission and the impact of transmitted data. As an integrated structure, the interaction network has its intrinsic characteristics. The network’s dependability describes the communication network’s capacity to meet the interaction needs of customers, investors, researchers, and pilots. The integrity of the communication networks refers to the sustainability of data transmission under various conditions. Integrity is an essential technological efficiency index regardless of the network’s planning and style or in the procedure and maintenance phase.
The invulnerability of the network suggests the dependability of the network system under operational service. With the advancement of the communication protocols, the effectiveness index is critical in the data transmission network. The network’s effectiveness is a dependability index based on the network efficiency, which represents the degree of the network system satisfying the communication efficiency requirements (Fas-Millán & Pastor, 2019).
The communication system is an intricate integrated structure made up of transmission, interchange, process nodes and support frameworks. Communication networks could be affected by equipment integrity, network design, topology, geographical location, and maintenance. Avionics networks have a collection of rigid integrity and security needs. In existing implementations, these networks are based on wired innovation, which supplies a high degree of integrity and security. Furthermore, it simplifies the safety and security management of the network since particular assumptions have been made. Avionics Wireless Network (AWN) deployment shows positive advantage in reducing wired configurations across varying topologies.
While providing these advantages, the AWN must provide adequate security levels compared to wired transmissions. Replacing the wired network with a cordless network brings an entire collection of obstacles connected to dependability, safety and security. Modern aircraft are a collection of a reliable and efficient computer system. Avionics Data Networks (ADNs) inter-connect numerous avionics subsystems, transmitting information and messages control within a predefined period.
Consequently, non-critical activities during flight operations depend on ADN architecture. Therefore, ADN provides viable service to critical and non-critical operations. Managing these features is challenging and vital to the ADN’s implementation. Effective communication networks’ requirements include sets of sensors, actuators, and buses linked on the collaborative avionic network (CAN) or ARINC buses. The AFDX or similar technology links gadgets to critical subsystems like pressure unit control, exit terminal control and fuelling systems. The AFDX architecture light-powered mechanism like display screens, travellers details, oxygen masks, audial news, and relay board (Fas-Millán & Pastor, 2019).
As reviewed in different literary works, wireless network communication must have an access node, end node and relay node. These components provide the basis for quality communication links between the aircraft, pilot, and ground control centres. The access node functions as a user interface to the AWN that communicates with navigation systems. The end node is a peripheral component that connects to end devices in an aircraft. The end node acts as an interface node attaching the end devices with the AWN and other avionics systems. Relay nodes connect navigation devices to the end nodes. In a multi-hop link, relay nodes permit internal interaction between accessibility buses and end nodes.
Summary of Objectives 2
Data transfer, generation, evaluation and transmission relays communication networks for optimum performance. The choice of network communication equipment depends on the aircraft, manufacturer, or technology. However, communication links must be reliable and efficient to overcome notable communication issues in aviation. As approved by aviation regulators, these networks standards mitigate system collapse or communication disruption during high altitudes, bad weather conditions, and geographic locations. Communication over any network nodes or logical links should have discretion.
The content of the communication is substantial, not the communication itself. Thus, network confidentiality requires that data links be reliable, confidential, and secured. The reliability of the communication network depends on its properties, attributes and quality. Studies have been focused on the dependability aircraft network architecture as it affects power system, bus structure, and information transmission. Consequently, some researchers examined network architecture based on the interaction system’s reliability formula and structure consistency analysis. The idea of integrity analysis of the ATC communication network system utilises different failure metrics such as MTBF, MTTF, and MTTR to evaluate standard items’ reliability.
The integrity analysis can be evaluated using non-redundant architecture, an utterly repetitive system, and a partial redundant system to assess the entire network’s consistency according to the system’s topological framework. With the expansion of traffic control frameworks, the essential services lugged by the interaction network are raising, and the task of the integrity of the communication network is immediate. A standby device shows several other devices or task functions with similar responsibilities. Therefore, one device’s failure triggers the fault surveillance or conversion gadget to activate the same function.
The consistency of the interaction network reflects various variables and mix of monitoring and modern technology. Aircraft reengineering triggers the evaluation of the network consistency to enhance secured and effective communication network and optimise network designs. Aircraft reengineering would improve system performance, enhance data safety and security while monitoring of the interaction network. As the system becomes multifaceted, social influence on integrity is enhancing.
Thus, aviation experts must strengthen the study on integrity communication architecture and standard requirements to aid trend adjustment, technological advancement, and scientific administration. This strategy would enhance the used o big data frameworks for effective communication integration with high performance and reliability services. The network’s performance is a dependability index based on the network performance, which stands for the network system’s degree, satisfying the interaction performance demands. Value chain network hinders airline operations where integration and collaboration are not sustained. Therefore, the next section explores products, suppliers and supply chain in aviation.
Objectives 2: Product, Suppliers and Value Chain in Aviation
The impact of globalisation, civilisation and specialisation on production has been overwhelming. When organisations manage different activities, it is inevitable for any business sector to execute their operations. As a result, supply chain management has been a collaborative investment. The effective and successful combination of these companies’ operations provides the business with enhances differentiation and advantage. According to Govindan et al. (2018), an investment supply chain’s performance requirement must be evaluated to maximise profit and satisfy customers’ requirements. Supply chain management establishes a system that facilitates the clients’ need and aligns with the investment objectives.
Therefore, the managers must gauge the performance of different parts of the supply chain. By implication, an efficient supply chain management enhances business performance. According to Badiezadeh et al. (2018), supply chain management describes several connecting firms and stakeholders’ collaborative objective that facilitates business circulation. Business circulation could be upstream or downstream. These streams provide life support for resource circulation. The production administration concept has been transformed from manufacturing activities. It has expanded to warehousing, transportation, and operations from purchasing raw materials via different activities until an item or services reaches the end-user.
Several literature works have analysed the performance of industries to ascertain the economic viability of the aviation sector. The authors examined cost behaviours of independent variables such as airline firms, airports, suppliers, producers and maintenance management. Mohib et al. (2017) examined the performance and sustainability of airline carriers. The findings show that poor monetary performance, negative returns on spent resources and questionable sustainability strategy characterised these business investments.
The authors emphasised that returns on capital employed are lower contrasted to various markets in the value chain. Many businesses outsource their supply chain and depend on producers to conduct multi-faceted tasks. The efficient mix of operations enhances performance in the business environment. To understand airport denationalisation’s financial stability, Lamba and Singh (2018) researched the role of non-aeronautical revenues for airport terminals. The findings show that non-aeronautical profits for over 54 per cent of revenues, showing value based on income generation for investors. The number of key aspects for lasting growth was determined including adjusting facilities for developments such as brand-new aircraft, producing a business version covering traffic created via retail services and home entertainment solutions and adjusting funding via the cyclical economic environment.
Value Chain Processes in Aviation
In aviation, the value chain comprises several agents of investments. The value chain can be separated into the downstream and upstream class. The aviation value chain’s upstream field comprises aircraft producers, outsourcing companies, resource capital firms, and travel infrastructure merchants. Under the travel infrastructure merchants, the value chain includes airports, ANSPs, Communication network investors, variable suppliers, fuel vendors, premium insurance merchants, or airport service team. The downstream value chain consists of e-commerce agents, circulation systems, travel representatives, travel integrators, and freight forwarders (Tretheway & Markhvida, 2014).
Airline companies have restricted control of each value chain of the multibillion-dollar investment. Over the years, airline companies have progressively divested their possession rate of interest in numerous industries of the value chain, either because of changes in national legislation, governing treatments, or strategies to enhance company competitiveness and financial efficiency.
Consequently, airline owners have invested in the specific supply chain such as gas suppliers, ground handling services, customer services, and other solutions. In some markets, airline companies have also participated in airport services, although this is currently developing. Despite the current vertical disintegration of the aviation value chain, the assembly of business agents is not a conglomerate of firms that operate in isolation. There has been significant regulation of standards and operations across the value chain. The regulatory standards have effectively mitigated sharp practices, upholding standard practice, reducing market prices, and enhancing travellers’ flight experience.
ICAO have developed requirements and laws to improve and standardise airport layout (Jin & Kim, 2018). The IATA established standards to buy and exchange travel papers, facilitated the clearing of economic purchases between value chain companions, and established channels for providers to obtain slots at airport terminals, which have to be linked in service times. The categorisation of the service industry seeks to sustain the sector, improve quality and enhance performance.
Productivity degrees and returns on investment differ along with the aviation value chain. Considering that the pendulum of policy turned towards market liberalisation of the airline industry, policymakers seek to encourage airline competition. According to Tretheway and Markhvida (2014) , performance level and ROI differs among the aviation value chain. The impact of global competition in aviation has seen the lower cost of air transportation and enhanced travel options for passengers.
However, the sector has witnessed low profitability and net earnings due to serval predisposing factors. Although most airline carriers accomplished affordable profitability, specifically with some affordable service providers and cargo carriers, financial stability has been challenging. As a result, aviation regulators induced or forced mergers or encouraged route reassignment. The strategy provides enhanced service continuity and equity sustainability. Post-deregulation financial protection and the competitive landscape in the airline company sector have changed exceptionally (Jin & Kim, 2018).
Boosted competitors combined with disturbances in traveller flows have increased due to natural disasters, terrorist attacks, outbreaks of transmittable diseases and economic collapses. These inputs put pressure of global return on investment. The indicator of growth must cover the cost of operations and generate huge ROI (Jin & Kim, 2018). By implication, the value chain’s overall performance most cover individual ages that make up the chain. Poor efficiency or productivity by one arm of the value chain could threaten the whole system’s sustainability.
Airline companies make the largest financial investment, primarily in new or substitute aircraft, airframes, engines, navigation tools, devices and corporate sources. Airline company financial investment around the globe accounts for over $806 billion (Jin & Kim, 2018). Flight terminals have extremely reduced property turnovers relative to airlines. The proportion of annual income to invested capital is 2 to 0 for airlines (Tretheway & Markhvida, 2014).
Therefore, the value chain must seek ways to improve each component and reduce the cost of operations. The gap in the literature accounts for the huge losses in aviation. However, big data could bridge this gap by providing a sustainable model for marketing and operations. The ease with operations can be enhanced with advanced technology and task management. A study by Jin and Kim (2018), analysed the rate of returns on different value chain components. The findings show that the profitability index varied with each component. Consequently, airline carriers were the last class on the rate of investment returns than other aviation supply chains’ markets. The author argued that most carriers posted a lower return on shareholders’ capital than other chain sectors. Contrasted to leading sectors that include distribution systems, travel representatives, and cargo forwarders air carries investments with 4 per cent returns on investment.
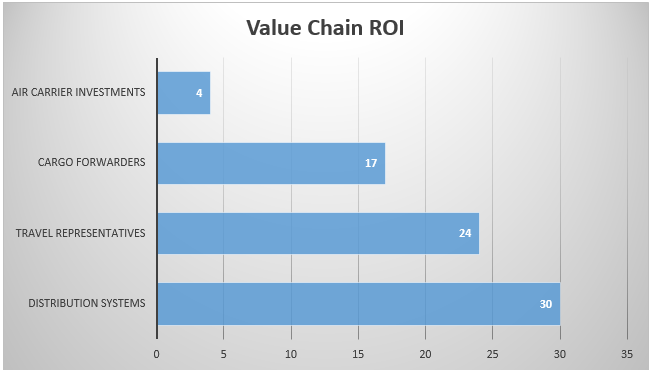
As found in Figure 1, the value chain components show the least profitable of the system. According to the research analysis, airport investors have been productive and profitable compared to airlines operations in terms of financial returns; however, it is still the second earner in the value chain. The return on spent capital is roughly 1.3 per cent listed below the industry’s eight per cent cost of operations. Although some airport management services have achieved profitable returns on investments, 27 per cent of airport operating business have accomplished returns of other value chain markets. The ROI for these air carriers are attributed to non-aeronautical solutions.
A credit and equity rating is another metric used to evaluate the investment’s sustainability performance. With extremely few exemptions, airline shares are not rated as investment quality. The proof shows poor investment opportunities in aviation under the current technology systems. Although airlines around report low returns on investment, the industry attracts a huge cost of investment. Economic experts have questioned the reason for more capital investments in a business that has low returns. A feasible explanation hinges on the industries leverage and returns on equity. Economic investment decisions depend on revenue and effectiveness in resource allocation. The effective management of resource allocation produces capital gains in little investments.
The thesis seeks to investigate the impact of big data on aviation. Big data technologies have shown favourable influenced on business management, administration, communication and data analysis. The aviation industry holds the largest volume of data generation. The assumption provides a valid reason for data analysis using an advanced and reliable concept. Big data is critical to improving individual sectors that make up the aviation value chain under the value chain proposition.
As a result, enterprises must preserve the affiliation between the inner and external value chains to facilitate productivity and enhance profit. Dou (2020) stated that airline operators and aviation regulators are confronted with data streams from numerous networks at an increasing price. Organisations with effective data management control have been reaping the benefits of outsourcing contracts. As a result, huge capital investment has been used to outsource data interpretation in aviation. An organisation without big data frameworks must collaborate with the value chain to improve quality service delivery. Thus, interactions between internal and external agents of the value chain could mitigate poor management practices. Investors with big data frameworks leverage the advantages of innovative analytics to drive their operations.
Big data sustains integrated planning and SCM as it recognises trends and choices. Data triangulation from market operations, sales, social networks, and data inputs provides the capacity to forecast and proactively plan supply chain tasks. Consequently, the IoT and RFID could be used for predictive maintenance to prevent unplanned downtimes. IoT can supply real-time data to expose the information of production practises.
ML algorithms could analyse data precisely and forecast unavoidable machine failures (Chen et al., 2021). As a result, big data services could prevent delivery disruptions by evaluating GPS information with traffic and climate evidence to optimise and prepare distribution routes. Big data applications enable supply chains managers to adopt a proactive reaction against chain threats. These instances offer benefits stemmed from the evaluation of huge data sources to boost supply chain agility and price optimisation. While the technology is relatively new, big data would positively affect aircraft management and operations. SCM concentrates on the flow of products, solutions, and information from the manufacturing plants to target customers with entities and tasks (Nguyen et al., 2018).
Although demand and cost analysis are known variables in SCM, customer needs vary and affect the supply chain. A selection of analytical analysis methods has been deployed in SCM consisting of statistical procedures. With the developments in technology, BDA is an effective and accurate forecast tool that reflects client demands, facilitate the evaluation of supply chain efficiency, improve performance, minimise response time, and classify threat assessment.
Big data is described as high-volume, high-speed, high- variability, high worth, and high validity information demanding ingenious kinds of data processing that allow improved comprehensions, decision-making, and procedure computerisation. Volume refers to the comprehensive dimension of data collected from sources over a specified period. For example, flight data of altitudes, acceleration, fuel gauge, engine status, and pressure gauge are retrieved in volumes and transmitted to ground control centres.
The generated information is created from sensors, bus nodes and other navigation tools. Data velocity describes the rate of information generation and transmission from one location to another database terminal. By implication, data velocity describes the speed of information collection, the integrity of information transfer, the effectiveness of data storage capacity and excavation rate of finding valuable understanding associated with decision-making models and formulas. Data variability describes data creativity in varied forms gathered from diverse sources. The sources could be artificial intelligence, Internet of Things, mobile phones, online media networks, and automated sensors. For example, SCM’s substantial data vary because of the diverse resources and mixed styles, particularly from numerous sensing units, merchant shops, and maintenance warehouses (Badiezadeh et al., 2018; Ghalehkhondabi et al., 2020; Nguyen et al., 2018).
Data value describes the nature of the information that has to be discovered to support decision-making. It is one of the most essential yet most evasive of big data technology’s five Vs. Data validity describes information quality and the understanding that unpredictability and unreliability exist. Data validity or veracity describes the consistency and accuracy of information. In summary, big data handles a collection of intricate, complex datasets that triggers challenging results under traditional processing procedures.
The literary works indicate numerous unstructured data sources throughout the supply chains with varied compromises among quantity, rate, and range, value, and veracity characteristics. Although the demand predictions in supply chains are attributed to lower limits of speed, quantity, variability, these projections can utilise information from all sources across the supply chain. This mix of information sources utilised in SC projections with their varied or spatial attributes emphasises big data supply chain and demand predicting initiatives. The big data applications in the supply chain have been reported many of supervised works of literature.
Many criteria are influencing the demand in supply chains. Nonetheless, these variables are absent in researches utilising conventional techniques. As a result, the forecasts might only supply a partial comprehension of demand variants in supply chains (Choi et al., 2018; Mohib et al., 2017). According to Grekhov et al. (2020), there is a non-linearity in-demand trend due to vendors’ rivalry, the bullwhip effect, and demand-supply disparity. Big data can be deployed to extract information from a huge quantity of data for decision-making. Decreased functional expenses, improved SC dexterity, enhanced service delivery are the advantages of applying big data in SCM.
Big data exist in all phases of supply chains, including procurement, warehousing, logistics, shipping, engineering, and sales monitoring. The conventional value chain of aviation does not have elasticity and inimitability. For aviation business, cost organisation is essential security for competition and development. An effective cost monitoring strategy enhances the decision-making process of business investments. Therefore, aviation investors must adopt cost management practice using big data technology. The adoption of big data in the value chain conquers imperfections, which provides new concepts for price monitoring, value creation and competitiveness of the transport market.
Value chain explains the variety of tasks needed to bring a product or service from conception to the final customers and disposal after usage. Porter’s model outlines six essential areas of the value chain: purchase, human sources, details innovation, logistics, procedures, sales and solutions (Opresnik & Taisch, 2015). Among these, essential areas, values and management are intangible assets in the basis of an effective value chain. Big data offer producers beneficial tools to execute their procedures sustainably. Xu et al. (2019) demonstrated how big data could increase remanufacturing systems’ efficiency and preserve assets. Dubey et al. (2019) examined responses to develop a structure that could deploy big data to identify factors for a sustainable production system. The findings showed that lower solution costs, business integrity between stakeholders, valuing consumers’ privacy, and enhancing data-sharing protection benefit big data deployment for sustainable production systems (Chang et al., 2020).
Huang et al. (2019) created a theoretical approach to demonstrate BD’s application in production safety administration. The findings showed that BD enhanced business growth and resource allocation for profit maximisation. Big data technology in value chain management has a positive impact on business growth. Santos et al. (2017) investigated BD’s benefits and the obstacles of gathering, integrating, and refining the data. The paper revealed that applying BD to minimise the supply chain’s threat could improve economic and social sustainability.
Big data could solve investment challenges at the rate of business requires. However, some technological and business barriers limits investment expansion and BD deployment (Li et al., 2018). Big data allows investors to design marketing methods, share data, predictive demand trends, and link organisations to regulate procedures (Kusiak, 2017). Bumblauskas et al. (2017) investigated big data impact on predictive maintenance. The findings show that prompt decision-making capacity reduced over billing and operation cost (Bumblauskas et al., 2017). As a result, managers used BD analysis to enhance an asset’s lifecycle. Opresnik and Taisch (2015) researched BD architectures in technological advancement and agile production. The findings showed a positive correlation between performance and BD deployment (Opresnik & Taisch, 2015).
Ren et al. (2019) evaluated the study of BD architectures that sustain lasting smart manufacturing plants. The authors argued that agility in a production system describes the efficiency of managing unpredictable events and transforming the cases into positive benefits (Ren et al., 2019).
An SC is a network of producers, transporters, clients, storage facilities, and traders. Supply chain administration manages resource distribution to guarantee the availability and solutions with the most affordable feasible expense (Madni & Sievers, 2018). Choi et al. (2018) revealed the use of BD in numerous SCM themes such as predicting revenue and threat assessment. It is crucial to consider the data schedule when establishing result simulations (Kaur & Singh, 2018). Utilising information evaluation, supply chain supervisors can check these circulations and use the outcomes to accomplish their tasks (Arunachalam et al., 2018).
Big data enhances the supply chain by boosting the presence, durability, robustness and business performance. Big data also boosts information management, raising SC by facilitating resource flow. As a result, big data influences cycle time, cross-functional reviews, decision-making, and efficiency optimisation. Therefore, transforming the available information to structured messages, which can alleviate business risk administration, is a growing concern.
Dealing with differing distributors, contractors, and logistic carriers produce large data sets for optimisation tasks in a supply (Gupta et al., 2018). BDA can be incorporated with other methods such as risk resource management to boost supply chain efficiency (Gupta et al., 2019). Hofmann (2017) showed that big data minimises the bullwhip impact in SCM.
The findings show that information sharing via large databases can minimise the uncertainty in expenditures. Big data can boost the environmental, monetary, and functional monitoring of the supply chain to mitigate climate modification (Hofmann, 2017). Badiezadeh et al. (2018) established a network data analysis with big data to analyse SCM performance efficiency. The findings show signification correlation and improvement in business investments enhances the supply value chain (Badiezadeh et al., 2018). The correlation between goods and cost variations. The authors revealed that target information enhanced precise promotions in a supply chain’s green atmosphere.
Supply Chain Management
Numerous international companies are outsourcing their supply chain logistics procedures as a means to rebrand operations. Arunachalam et al. (2018) studied serval companies and the impact of BD in the supply value chain. The findings reveal that data mining facilitated the choice of suppliers. More importantly, big data analytics provides the enabling platform to select a supplier based on critical factors.
The number of value-creating platforms has been helpful in coordination business operations (Arunachalam et al., 2018). Logistics providers manage the huge volume of product flow and have access to a substantial quantity of data. Any kind of quantifiable standard of item delivery is vital for value development (Govindan et al., 2018). Developments in tracking accuracy, sensing networks and advancements tag logistics have opened logistics and SC management. Therefore, maximising investment operations must be sustained to avoid system collapse.
For example, disrupted delivery and very early shipments could incur a cost for the organisation. Thus, planned distribution and precise shipment dates are an essential risk variable for logistics business. Weather prediction and automobiles’ efficiency information reduces the threat of incorrect distribution times (Li et al., 2018). The research findings show that BD in logistics can be specified as modelling and examining logistics systems using information databases created by GPS tools, mobile phone and logistics companies’ procedures (Mehmood & Graham, 2015). There are essential procedures in the logistics industry that requires an effective management system and data analysis. According to Hassani et al. (2019), demand forecast, transportation, stock administration, human resource management and monitoring can be enhanced by utilising big data.
Jin and Kim (2018) surveyed the impact of big data on logistics and found that a combination of BD and intelligence decreased the organisation’s cost of delivery. The findings classified the cost of delivery to include damaged goods, order cancellation due to extended time of delivery, and uncertainties. Data mining methodologies for SC monitoring can be categorised in threefold. The first fold covers data evaluation to match customer requirements and logistics procedure (Jin & Kim, 2018). The second fold covers data monitoring to match delivery processes based on systematic decisions and facilitates supply chain managers’ performance. Niu et al. (2019) examined several contending airfreight service providers and revealed the impact of data mining on its operations. The findings showed that freight provider benefits from RFID technology by upgrading the demand signals.
Although investors plan its fleet operations and service delivery framework based on-demand rate, the need for quality predicting and forecasting tools are vital for business performance. The adoption of control frameworks and data mining enables delivery agents to create customer clusters and map solution models based on individual needs (Niu et al., 2017). The era of target marketing using social media presence has triggered data mining technologies for product and service efficiency.
Therefore, the deployment and expansion of BD technology in SCM cannot be overemphasised. According to Santos et al. (2017), the adoption of BD technology in Bosch Automobile mitigates the problems of supply chain logistics. In the end, the organisation harness its operations to improve performance and enhance productivity. From a logistical view, big data enhances shipment forecaster, reduce waste time, and improve timely delivery.
Based on the research objective, the analysis seeks to evaluate the impact of big data on the aviation value chain. The review examines value chain concepts as it affects the industry. Consequently, the study is guided to optimise the supply chain between aircraft manufacturers and investors. The requirements include efficient quality classification, responsibilities on specific value chain levels, strategic coordination procedures, and planning. According to Phillips et al. (2019), the price to expand business investments is higher than adopting a robust value chain management. Accordingly, effective value chain management would enhance other sectors of the investment. In this context, the combination of data assimilation and communication networks would enhance aviation’s value chain. According to the survey by (Li et al. (2021), investments with efficient communication networks have 78 per cent understanding of the dynamics of the value chain and the capability of the outsourcing contractors.
By implication, investors who understand its supply chain’s capabilities could integrate production, operations and management components. Thus, communication enhances internal transparency of each production line for prudent resource allocation. The use of appropriate data evaluation and transfer techniques influence internal transparency. A survey by Tretheway and Markhvida, (2014), showed that only 25 per cent of aviation investments provide a monthly forecast of its operations and market trend, while 50 per cent of airlines do it quarterly. The findings show that organisations with daily updates performed better as they understand trend shifts and demand fluctuations.
Data value chain network (DVNC) facilitates investor’s capacities to manage its supply chain and logistics. DVNC describes variables that create ad-share information via network model. Hence, value creation processes, have become energetic, agile, and dynamic. New information attributes have emerged, needing technologies and frameworks to process data and manage value from such information. DVC facilitates the deployment of big data technology to create value in any organisation. In an organisation perspective, scientists define BDVC as a system that controls unstructured messages from data collection, analysis, decision-making, support, stakeholders section, and technologies (Arunachalam et al., 2018).
The framework allows investors to consolidate value and use informed decision to enhance efficiency. The DVC phases include data discovery, data assimilation, acquisition, conversion, storage, processing, analysis and visualisation. BD application facilitates DVC to enhance performance. In answering the thesis objectives, the researcher classified agents of data value chain.
The review proves that big data technology would enhance the value chain process in aviation. By implication, the airline investors can reorganise and revamp their investment portfolio with an informed business decision. Sustainability in airline investment means business operations must be funded, and service quality must be efficient. Flight delays or flight cancellation must be reduced to avoid losing customers. Consequently, aircraft maintenance must be done to avoid cancellations and high cost of maintenance.
The attribute motivates the next thesis objective on the impact of big data on maintenance. Since aircraft maintenance received over 40 per cent of investment capital, investors must apply advanced maintenance strategies to predict aircraft faults and avoid overbilling or outsourcing repairs to inefficient and unqualified third-party agents. As a result, the next section reviews existing aircraft maintenance procedures and explores solutions with BD.
Aircraft Maintenance
Due to the strict specifications in aviation, the plane maintenance method is regulated. The FAA and EASA must permit the MRO institutions to operate in the supply chain. The principal job of the MROs is to ensure the airworthiness of the aircraft’s, in the course of the periodic plane inspections or unscheduled collection maintenance. MROs are not regularly private in the aircraft routine maintenance, as many OEMs and part vendors deliver servicing companies to the airline’s drivers (Rezaei Somarin et al., 2018).
The clients either assets the rarely substituted parts or buy from the MRO companies, producers, or reps. Tier one distributors are crucial agents in the products and suppliers segment the supply chain. Tier one distributors collaborate with sub-tier suppliers to manufacture parts such as the aircraft structure, motors, interiors, avionics systems, landing equipment and other intricate parts (Rezaei Somarin et al., 2018). The aircraft manufacturer’s responsibility is to supervise the assembly of the aircraft and ensure standard practice as per the specifications. Research studies indicate that an even more nimble and efficient source chain system can be built to integrate additive manufacturing (Rezaei Somarin et al., 2018; Tretheway & Markhvida, 2014).
Aeroplanes value chain comprises types of machinery and subcomponents, market type, distribution and production framework. Considering the complexity of supply chain arrangements and the necessity for faster maintenance frameworks, the industries often sustain greater amounts of stock. There has consistently been a constant struggle to minimise the supply degrees and countless tactics such as anticipating the parts demand, utilising protocols to anticipate repair criteria or part failings, and optimising the supply chain setups have been analysed and implemented.
The MRO companies are empowered by regulatory bodies to provide aircraft parts arranged or unscheduled routine maintenance activities. Airplane MRO investments could be interior departments of the end customer company or even a legal companion from various MRO systems. Aircraft components typically comprise of numerous parts that require examinations and such demand are commonly erratic. These are crucial variables stretching the stock levels to the limitations, though routine substitute takes over 10% of additional parts (Tretheway & Markhvida, 2014). Components needing frequent replacements are difficult to forecast the repair routine and are expensive. These aspects make it challenging for products and suppliers as it triggers the overall ineffectiveness in the supply chain network in aviation.
High inventory expenditures may keep the aircraft operational, but monetary costs become higher than usual. The supply chain associated with aviation encounter issues associated much higher expenses. Problems might start as very early with aircrafts procurement. The need for specific engine parts and future repair logistics influences the investor’s decision-making process. Beyond the regular parts, some components must flow custom production, which calls for additional layout activities, further rearing the SC and controls’ unpredictability. Aircraft customisation and design choices create challenges, supply chain disruptions, and high procurement cost.
The aircraft field’s attributes, the higher level of customisation, and the unevenness of components affect the aviation supply chain. According to Eckstein et al. (2015) it is difficult to supply equipment and services while sustaining positive earnings. The criteria to generate high-quality parts with pricey products opportunities pressurise the producers, as lead times are stretched due to traditional production strategies. Blending the high cycle inventory and lengthy procurement lead times, investors must maintain higher security inventory by securing a capital-intensive budget for procurement. The economic situations of automation influence the supply of aviation products.
Consequently, the quest for products creates disruptions in the supply chain. Notable, the demand for titanium, aluminium, carbon dioxide fibres, and their composites stretches the supply chain. The lack of design autonomy, endangered product, and unchecked parts cause additional supply chain constraints. Sustainability and eco-friendly concerns have become significant in the contemporary style and production tasks in the aviation sector. Regulators are committed to minimising the influence on the climate to strengthen the manufacturing tasks and reduce aircraft emissions. However, for small production volumes, the standard production techniques are inefficient.
Consequently, large production plants design products via heavy machining, through which the product waste might be over 97 per cent. Government policies and aerospace regulators bodies have established lawful conditions to reduce discharges, warranting the weight decline as the main purpose of aircraft OEMs. Airline operators are willing to invest huge capital funding on aircraft with remarkable fuel productivity, given that gas expenditures take over 38 per cent of their operational costs. Nonetheless, standard production methods have currently arrived at its peak in attaining the weight declines. The endless craving for lighter designs triggers the hunt for even more alternate innovative techniques (Eckstein et al., 2015).
The product and suppliers network that make up the supply chain supply are intricate and multi-directional (Milambo & Phiri, 2019). Concerns focus on the restrictive and taxing production method presently utilised. Considering the current progressions in the additive manufacturing procedures in the routine maintenance business, big data technology enhances service quality. It is important to examine big data in aviation. An effort was made to evaluate the current progress towards combining the additive manufacturing systems with big data.
The additive manufacturing framework facilitates new services, and the innovation is evolving to be among the greatest achievements in the products and supplier network. Uninterrupted materials into intricate 3D forms based upon the electronic data allow considerable time and cost reduction where the innovation is suitable for producing equipment components. The development from 3D forms to prototyping and free form manufacture indicates the characteristics acquired at distinct technology expansion stages. According to the 2016 Wohler’s document, the additive manufacturing sector made a capital investment process of about 6.166 billion USD (Mohib et al., 2017).
Additive manufacturing systems (AM) can be categorised into seven areas of production. The SLS, SLM, EBM, SLA, FDM, and MD are widely used in additive manufacturing systems. The additive manufacturing system is better than the conventional systems because it supports lower power consumption, better mechanical performance, lower product waste, and shorter production time. The capacity to create intricate 3D forms from digital data, getting rid of the demand for complicated tooling reduces the manufacturing lead times, and achievable localised fabrication supports a dependable supply chain network (Mohib et al., 2017; Ren et al., 2019).
The additive manufacturing techniques integrated with various areas of production, including the aerospace markets. Many surveys have investigated, and documented findings on the impact of AM in aviation and their views are summarised in this section Investors in aviation consider markets, routes and regulations in the supply progression method. Registration in an airline partnership indicates that such selections are taken collaboratively with other airline partners. The supply chain network seeks to optimise market value, as the supply establishment network’s general aim is the optimisation of the market worth and network security to facilitated network manipulation (Mohib et al., 2017; Ren et al., 2019).
The investments, lease of planes, selection options for optimising network service are crucial choices in the product advancement process. For instance, the distribution of hub had enforced planning teams to handle their network in its entirety by booking departure waves and coordinating flights. Demand patterns and forecasting are critical data that assist the decision making the process of supply management scheduling divisions utilise several management systems to sustain airline companies in organising their routines. Such systems deliver seamless assimilation along with various other units, featuring. Through collaborating with supply management teams to establish a joint precautionary analysis, concern on-demand within the supply chain is decreased to mitigate request amplification.
Global distribution systems (GDS) utilise different strategies to constrain other airlines from retrieving flight data. Since the GDS proprietor is shareholders in aviation, it is argued that their allegiance to specific investors affects the supply distribution chain. There is a growing competition among airline investors and the supply network. The need for routine maintenance parts has been the drive towards a competitive advantage among airline operators. Therefore, big data technology could ease the strain as predicated in several works of literature.
According to Badiezadeh et al. (2018), the deployment of big data in the supply chain network eases the hassles demand surge and deliberate time-wasting. The demand for data has a knowledge pool for leading airlines as they study business trend, market dynamics, customer traffic and equipment maintenance. For example, British Airways allows the sales of rival tickets on its platforms to monitor demand data and other interest areas.
Ordinarily, the managers intended to collect the required information from online order other than diverting customers to their platforms. Other proof of cooperative product and supplier alignment is the deployment of A380for long haul tours as it improves airport terminals’ traffic. Partnerships and cooperation would be required for aligning air travel opportunities, allowing ticket interlining and product procurement to improve investor’s assets while categorising the dynamics of airline networks. Airline investors conduct joint procurement contracts to accomplish economies of scale as it reduces prices and arranges better investment packages relating to routine maintenance and delivery time.
Consequently, collaborative supply management facilitated resource optimisation through equipment exchange and product swapping such as navigation tools cabin sensors and other system materials. The shared investments of Airbus by Star Alliance partners operated by the partnership agreement is an example of a collaborative supply network. Consequently, Lufthansa signed an agreement with Star alliance for an ‘Aero-Xchange’ market in which investors can contract online swap deals for equipment, fuel and aero engines. Procurement trade is economically viable for airline investors by creating transparent markets and facilitating huge procurement transactions. Promoting B2B platforms and adjoining extranet system improves product-supplier chain network (Badiezadeh et al., 2018).
Based on the above submissions, airline investors must integrate their production schedules to avoid overbilling and improve service delivery. By implication, investors must consider its fleet management system and assign specific aircraft to either long or short routes. Consequently, investors must consider aircraft maintenance scheduling to resolve servicing demands following international standards. Crew booking management will reduce overhead expenditures and improve service efficiency.
To improve resource functionality without breaching regulations and policies arrangements, airline companies must collect and evaluate huge data to coordinate their resources. As a result, big data facilitates the accumulation and analysis of data in aviation. The vast methods and frameworks of big data analysis encourage technology integration in aviation markets. Big data analytics can be infused in-demand systems or platforms to manage procurement schedules, detect fault delivery systems, forecast logistics routes and aircraft requirement. Workflow management systems that sustain supply and product chain distribution of air travel programs need demand data inputs.
In particular, Flight Watch Website gathers evaluate and displays information about booking rates, weather outlooks, workers rotations, and flight terminal limits to mention a few. As airlines often possess or generate a vast network of hubs to assist ground control centres in monitoring, reporting and observing operational details (Choi et al., 2018; Tretheway & Markhvida, 2014). Thus, airports and airline companies must collaborate to minimise equipment turn-around times, reduce traveller disruptions, delays and enhance supply utilisation. As a result, SC systems aid station supervisors to prepare operational guidelines and ensure continuous equipment delivery.
Collaborative supply and demand network is pivotal in triggering the SC processes in aviation. The task of GDS in creation, as well as functions, is prolonged even more. Thus, global distribution systems extend their functions beyond procurement and suppliers but even pricing and promotions to enhance service delivery in aviation. GDS technology acts as pillars and resources of numerous airline company for joint advertising initiatives and partnership programs.
The advertising data collected through GDS is used for promoting and implementing initiatives to improve customer interaction. The pact between investors and supply chain organisations represents the collaborative alignment among contending airlines with the guidance and permission of the GDS. The objectives and benefits of such collective practices are numerous amongst which the significant context are listed here. Under the pricing and promotions chain, GDS manages online competition from several travelling websites by developing a marketspace that encourages prices transparency.
GDS provide platforms that allow airline investors and managers to circumvent the antics of travelling representatives. Such strategy encourages collaborative support among service suppliers, passengers, and it reduces the cost of operations. GDS improves revenue management by forecasting ticket mixes and double-booking charges. The principle of yield network monitoring is administered, while several styles have been established and executed to enhance the chain network.
The significance of GDS for airline distribution and development of purchase points has been identified in different works of literature. The dawn of the Internet and the succeeding overview travel websites, each carrying out different eCommerce business versions, have allowed innovation within the airline establishment. Fasone et al. (2016) argued that e-commerce platforms improved the importance of GDS in airline company e-distribution. Exclusively, the GDS innovation is the reservation engine behind numerous online presence airline companies, travel agents, excursion operators, company vacation brokers, online shops and auction websites.
As previous research also reports, over 78 per cent of online ticketing is empowered through GDS innovations (Kasim & Mahmut, 2020). GDS supports income streams, improved tour sales and supports SCM. Therefore, rather than developing electronic presence, technology-equipped online airline pyramids through GDS subsequently assist the advancement of specific airline supply systems. Abreu et al. (2017) categorised the airline digital power structures handled and equipped by GDS in three groups of an online presence. The categories include forward or backward auction presence, local or reverse exchange markets and global trade zones.
In such case, airline company systems would have the capacity to increase system turnout monitoring by applying yield costs policies that would vary fares based upon the reservation date, distribution network and consumer. Airline distribution conducts publication of air travel schedules, fares rates, and distribution of airline tickets. Airline partnerships are offering after-sales support systems. The framework includes the collection of various CRM techniques. These web sites offer clients the opportunity to discover and gather information concerning their air travels, plans, locations, airports facilities, location weather and travel needs using their PNR numbers.
Aircraft Maintenance and Big Data
Aircraft maintenance is a planned manufacturing procedure that mitigates the challenges of total breakdown. The objective of maintenance capability is to develop the needed resources to deal with anticipated maintenance work and establish the exact variety of service technicians for the uncertainty. Aircraft maintenance comprises scheduled checks or monitoring, consisting of time limitations arising from inconsistencies identified via a scheduled maintenance check. The maintenance checks are triggered by routine checks, aircraft breakdown, pilot reports, or data evaluation (Lestiani et al., 2017).
The uncertainty of routine checks and planning procedure is influenced by spontaneous maintenance. Regardless of the volume of data produced per flight or information generated during engine maintenance, there have been inadequate in offering a competitive advantage or influencing the decision-making process. According to Xu and Kumar (2015), fault detection methods do not utilise the value of data generated from tons of flight information or cost applications from previous maintenance exercise. MRO companies count on the decision maker’s knowledge in approximating ‘safety shields’ for workforce or budgeting, usually overestimating workloads and expenses.
By such actions, MROs neglect or improperly address the decision-making procedure’s uncertainty and assume a substantial threat regarding the ability to complete the workload and perform according to the contracted service price. Big data technology generates global awareness for its capacity to retrieve valuable information and knowledge from unstructured data.
Consequently, with the expansion of sensing technology and ICT, data are accumulated to sustain decision-making. The detection of errors in these data is a crucial application in maintenance remedies, enhancing decision-making. Prompt discovery of system faults might ensure commercial systems’ integrity and security and minimise the danger of unintended breakdowns. Intricacies in the data accumulation, consisting of high dimensionality and dynamic data streams, impose difficulties on fault discovery applications. Data nonlinearity triggers the ‘curse of dimensionality’ that creates corrosion in fault recognition systems (Gao et al., 2018).
Data streams necessitate systems to provide accurate reactions based on new samples. Several approaches have been suggested to adjust existing models and enhance their functionality with data mining to fulfil information streams’ needs. Several research studies have examined the challenges linked with high dimensionality and information streams. Arline maintenance practices have evolved from corrective maintenance applications to preventive fault detection practice. Under corrective maintenance practice, managers deploy a ‘fail and fix’ attitude that triggers unscheduled closures and ecological challenges.
The anxiety of system collapse encourages firms to inspect the engine parts before complete shutdown. However, preventive maintenance activities are guided by an established schedule or the health status of the aircraft. Although unanticipated failures can be lowered with a preventive maintenance approach, the practices maintain equipment disproportionately, causing high maintenance costs. Constant engine maintenance creates more system failures. Studies on aircraft predetermined repairs show that most engine failures are noticed after a comprehensive maintenance activity.
Predetermined maintenance accepts the collapse pattern of most aircraft is predictable. The PM design presumes that aircraft declines inevitably via a defined order. The deduction varies with other predisposing factors such as aircraft ageing, ecological effects, procedure drift, and intricate communications between components and systems. Surveys on failing behaviour argued that maintenance challenges are caused by arbitrary procedures. Based on these facts, corrective maintenance is prone to ‘inadequate repairs’, while PM triggers ‘over-maintenance’. Thus, big data bridges the gap between CM and PM fault-detection approaches because the technology forecasts future failings based on the aircraft’s health status during routine checks.
The distinction between traditional repair approaches and big data induced checks is that signals are triggered by data evaluation and analysis. The principle of predictive maintenance utilises innovations to support a shift from ‘fail and fix’ procedures to a dynamic design that predicts, prevent and fix engine challenges. Based on this understanding, the layers of the existing maintenance framework are summarised. Aircraft maintenance describes a mix of technological, management and supervisory schedules of equipment to retain, recover, or maintain the operation’s status. Therefore, mix approaches sustain the shift from rehabilitative maintenance methods to preventive methods,
Data accumulation during flights provides resources for most predictive maintenance routine. The uses of sensors and other observatory equipment enhance data retrieval processes. For example, a Boeing 787 provides data on equipment status, gas levels, altitude ratings or pressure gauge during flight operations. Big data technologies utilise smart intelligence designs to detect fault lines and hidden signals or anomalies of potential errors to maintain and prevent significant failures and engine collapse. Due to the catastrophic nature of mid-air disturbances, airlines are continuously designed to screen, evaluate, predict, and rate equipment performance.
Most manufacturers are adopting a continuous shift in predictive approaches using advanced learning and technology. Aeronautics sector accounts for three per cent global anthropogenic emission, which is about 14 per cent of the entire transport sector. The market has experienced over 45 per cent of the functional costs generated from fuel charges, surpassing operational costs in other industries (Jin & Kim, 2018).
Therefore, the aviation sector is influenced by financial and social environmental factors, while stringent regulatory structures enforced for sustainable environment conservation have threatened airlines’ sustainability. Needing to abide by standard contamination tolerance, aeroplanes require routine maintenance culture. Efficiency optimisation in aviation targets functional constraints from contamination, fuel efficiency, resource utilisation, and aircraft costs to guarantee the sustainable lifespan of aircraft.
Performance optimisation could either overcome existing or expected issues, which restricts the operational effectiveness of components and equipment of the aircraft. The routines against these restrictions have been much correlated with the element’s ‘wellness’, which suggests adequate maintenance such as health monitoring and abnormalities resistance actions would enhance performance. Thus, airline investors have used serval maintenance architecture to deal with complex equipment ruining, operations and service frameworks. The maintenance architecture depends on the investor’s choice or geographical location.
Existing Maintenance Architecture
As the name implies, AIRMAN is a live surveillance and diagnosis device created to supply early detection of abnormalities and promptly boost efficient resolution minimising aircraft interruption, failures or downtime. The system gives signs of handling large data and fault predictability. Many airlines, such as Emirates and AirAsia, benefited from the device in conserving fuel expenditures. Nevertheless, GE introduced a cloud system called ‘Predix’ to analyse flight information of approximately 34000 engines which gives irregular alarms and a gas-projecting model. The device confirms the exploitability data centres, cloud computing and big data evaluation as essential components.
For example, P&W developed a system for forecasting engine fault detection by monitoring and storing over 5000 commercial engines. The system allows investors to observe over 2000 specifications from an operational engine, which creates a precise and reliable forecast of 94 per cent accuracy. According to studies, the strategy reduced the downtime of the investigated airlines by 56 per cent (Verhagen & De Boer, 2018). Microsoft Azure platform was designed to aggregate, integrate and analyse flight data and optimise energy consumption to improve engine efficiency. Azure systems maximise aircraft efficiency by raising gas combustion retention and maintaining engine performance via comprehensive monitoring. Adopting several predictive surveillance systems such as ADS-B suggested potential application of big data technology and used cases for weather analysis and performance optimisation.
The applicability of data analysis in improving aircraft efficiency is a dynamic trend in aviation. Flight criteria or functional trip information are captured from various sensors in avionics and other components. Maintenance systems gather information from frameworks such as ACMS, QAR and ACARS (Verhagen & De Boer, 2018). IoT based maintenance is crucial to the airline market because the technology provides platforms for predictive fault detection. Contemporary studies initiated by large producers of engines and equipment have adopted several mechanisms to enhance product performance. Therefore, big data technology supports the accessibility of relevant details concerning streams of aircraft information.
Airline management and system maintenance comprise many trouble locations that require effective scheduling, monitoring and repairs. Arranging the maintenance lists is challenging because each unit includes planning, controlling, and implementing varied components, resources, suppliers and vendor. Therefore, repair scheduling must acknowledge the multifaceted distribution of events to enhance performance. Aircraft MROs must accommodate large project networks comprising several activities, multiple components and different maintenance levels, assimilation of products, resources, procedures scheduling, and project preparation (Korvesis et al., 2018).
Consequently, the system must deploy several frameworks, configurations to detect fault lines. The configurations are either fitted internally on the aircraft or gathered from distant sensors or servers wired to collect data information and trouble spots. After collecting tons of data, the system architecture prioritises the trouble locations based on specific risk levels. The risk level could be engine maintenance, heavy repairs, configuration management or component realignment. Heavy repairs are categorised into overall maintenance and detailed scheduling. Several works of literature reviewed aircraft maintenance and its frameworks.
Semaan and Yehia (2019) explored aircraft-scheduling challenges repairs were carried out in one trouble location. The author attempted to fix the scheduling trouble using data analysis approach. The findings recommended that aircraft maintenance scheduling’s practical allocation required detailed information on the equipment’s flight data, configuration, travel time, and previous routine records. Aircraft configurations and data records must be updated before undertaking overall maintenance scheduling. The authors established algorithms and methods in handling optimisation of different examination and repair schedules of systems.
The optimal upkeep timetable incorporated the assessment results, routine checks, delayed uptime, downtime, and detection expenditures. The methods of differential calculus were useful to the transformation of the optimum upkeep timetable. The paper concluded that service-scheduling design utilising hereditary principles improves aircraft repair routine. The model used intelligent machines to create routines for the upkeep automobiles to cover all flights.
Nevertheless, the version does not permit organising direct sources associated with aircraft maintenance. Selcuk (2017) categorised maintenance management into scientific and engineering techniques. The scientific method covers the expansion of dynamic algorithms using statistical techniques to analyse aircraft components’ fault pattern. The engineering technique covers exploratory orientation. Nonetheless, various engineering techniques attempted to resolve approximate numbers of components when handling aircraft routine checks and fault repairs. Additionally, the models used per time depend on location, investors and aircraft. For example, the UK prefers the terotechnological model, while the US adopts the life-cycle cost (LCC) technique.
Dutch aviation designers adopted the EUT design since testing could not guarantee accurate results (Semaan & Yehia, 2019). Therefore, maintenance versions seemed imperfect and approximate yet. By consistently examining the results gotten from numerous algorithms, it is possible to adopt designs that offer an accurate solution. The limitations of model maintenance architecture have been a growing concern in aviation. The majority of applications concentrate on fault detection and lacks systems integration. Aircraft maintenance could be reactive or proactive as it depends on the airline, investor’s experience, and available decision-making tools (Semaan & Yehia, 2019; Xu & Kumar, 2015).
Existing maintenance procedures used the reactive technique to form a corrective practice. Corrective repair patterns are a failure-based design used to mitigate equipment collapse or anomalies. However, proactive maintenance technique could be preventive, conditional based repairs or predictive fault detection. The preventive repair pattern is a schedule-based practice that relies on equipment use history and operations.
Predictive maintenance is a model-based approach or a data-driven technique. Model-based practices utilise physical or knowledge models. Part 21 firms authorise airworthiness recommendations for maintenance based on international regulatory standards. Such airworthiness information includes a major fixing plan, performance optimisation, and minor fault detection. Maintenance information published by the engineering department could be a design illustration or a form record sheet (Semaan & Yehia, 2019). The maintenance department or firm must establish a procedure under regulatory standards. Modification to repair regulations must be documented for reference purpose. EASA Part 145 allows the transcription of repair data into worksheets.
Objective3: Research and Development (R&D) Activities
This section of the paper investigates the impact of big data on aviation. As a result, the researchers examined R&D actives in aviation. With the developmental trend in aviation growth, the study reviewed several literatures on R&D development in aviation. In evaluating the impact of big data in aircraft, several objectives were reached to ascertain the study’s collective purpose. The business models in aviation are undergoing rapid changes, triggered by the demand for cost-efficient solutions. Investors in aviation are searching for a flawless design for safety and enhanced travel experience as passengers clamour for improved air travel service and reduced cost.
Meanwhile, expansions in innovation have undertaken new designs with an array of operational objectives. Research and development programs in aviation make advanced modifications to alleviate aviation challenges and enhance FAA decision-making process. Therefore, investors in aviation must align innovative design with the FAA’s requirements and regulations. Thus, R&D in aviation aims at improving access to quality research, enhancing efficiency and ensuring safety. The unmanned vehicle architecture is evolving to build innovative solutions for public airspace by establishing new devices.
R&D impact aviation sector by mitigating the challenges of harsh weather on reliable air traffic administration and navigating technology to reduce tour time, costs, or environmental impact. R&D aids the development, model, and replicate capabilities to facilitate FAA choice on regulations, principles, and operations. Based on these assumptions, R&D activities support enhanced compatible among countries, aircraft and global aeronautics facilities (Grekhov et al., 2020). Big data in aviation allows the accurate detection of risky failure and possible fault detection analysis. Since aviation relies on innovation, it is imperative to safeguard the service architecture against cyber-attacks, unauthorised access, or terrorist hijack. Therefore, R&D initiatives allow experts to focus on procedures that counter unauthorised access to ensure data safety or flight efficiency.
Capital funding for research and development is huge and challenging. Although individual companies are conducting serval R&D innovation, they may not profit directly from such technology. The study summarised a survey of ten global investors in R&D programs (Grekhov et al., 2020). Currently, innovation expertise is emerging as a strong instrument for open development in technology-based companies. Therefore, using technological tactics and effective resources is an unavoidable responsibility for qualified advancement of organisation firms in machinery fields. Modern technology is vital as a resource for building different kinds of equipment and products, leading to innovation skills and capability.
According to the technology-based ambience of technology, organisations are focusing on open innovation as a fundamental aspect for their competitive aptitudes. Development in aviation is based on the investor’s functionalities and capacities. Consequently, to observe technical modifications in these organisations, development, growth analysis, and market teamwork is crucial. According to the findings of Grekhov et al. (2020), three is a seeming correlation between research and development in aviation. The author argued that research and development activities by either investors or regulatory organisation trigger competitive atmospheres and supports safer operations.
Despite the anticipated favourable results, few businesses have been useful in practising open technology. The majority of commercial instances belong to program market, where ‘available source’ development has become an acceptable phenomenon or B2C environment. Research and development address higher technology requirements by opening the advancement process, incorporating external technologies, and developing threat and earnings sharing contracts. Its special techniques have impacted each era in the fourth wave of aeronautics advancement. Thus, aviation markets require new conceptions for producing technological capabilities to enhance service delivery.
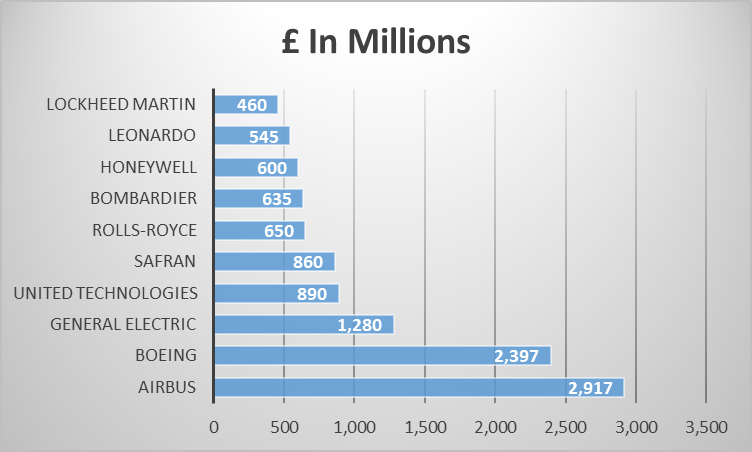
Global investors provide a competitive technology program in aviation. As found in Figure 2, Airbus leads the R&D program with over two million pounds investment. As aviation experts consider the scope of scientific studies and knowledge, to obtain technical advancement by only internal study and design is complicated. Open advancement strategy is administered for creating and utilising a new technology. As a result, some organisation control and manage business dynamic by experimenting with ideas to overcome new demand requirements. As the expansion of the knowledge domain continues to trigger development in aviation, data accumulation via big data must be evaluated to draw recommendations from serval work of literature. The aviation sector is diverse with many businesses in commercial, defence, military projects and applications (Grekhov et al., 2020).
Despite the project and application, the aerospace sector items are identified as sophisticated products because of the continuous technology progression and capital funding. Investor’s complex needs are growing with a new dimension under safety regulations. These physiognomies make the aerospace industry exciting for innovative ideas, similar to other manufacturing fields such as the nuclear energy market. The aerospace sector possesses a record of generating advancements. With its history in innovations, the aviation sectors have triggered the mission of ‘higher, quicker, and farther’, product technologies aimed at improving performance.
The market launched several new advancements, such as commercial trips, fast flight, and space travel, and are relentless to define its attributes. Most of these technologies were components of complex systems, including landing gears, communication cabin, traffic control service, or GPS navigation. These innovations were the outcome of cumulative growth initiatives that combined technologies coming to create complex devices. Consequently, innovative ideas dedicated to satisfy passengers and enhance performance are evolving. For example, the FAA has been observing the quest for a joint strike fighter program (JSF). R&D initiatives in aviation include product improvement, integrated systems, system solutions and large intricate designs. These innovative ideas could be turbine blades, MRAP, bio watch, or the JSF and FCS.
The emerging-market stimulates strategic advantage to firms in aviation. As a result, research and development initiatives create opportunities such as cost and risk sharing, external competence, and employee involvement. The initiative allows more firms to combine resources in creating efficient systems. Because of the collaboration between several organisations, R&D enhances cost spread and risk reduction in manufacturing. The initiative creates a channel for external participation in the production drive. Investors in science development may enter the aviation challenge without having airbuses.
The development drive and open innovation trends trigger investors participation from different sectors of the economy. In this regard, adopting the available innovation technique could enable investors to focus on a provider to capitalise personal knowledge and expertise by building products that attend to new market requirements. Thus, if the innovation fails to achieve its objective, the manufacturers could sell its patent to other business sectors for monetary gains. Business investors can visualise market value in accelerating their product by licensing-in the innovative patent (Grekhov et al., 2020).
The strategy allows investors to transform and consolidate verified innovations at lower costs, therefore minimising the probabilities of breaching the arrangement. Several aerospace providers are endeavouring to include services and product in their prospectus. The strategy classifies service solutions based on utilisation capacities. To achieve this function, firms must cooperate with partners and service system. Thus, an open technology approach allows the organisation to expand the development drive and facilitates product creation using new modes for success.
Knowledge is crucial in research and development initiatives. Consequently, due to the complex nature of R&D in aviation, innovation administration is another significant variable. However, rapid modifications and technical advancement impede resource allocation and decision-making without comprehending current and future trends. Therefore, technology intelligence bridges the knowledge gap in the organisation decision-making process. Technology research studies have stressed the growing importance of external sources of development. Technology networking is a critical measurement of advancement, and the role of inter-organisational connections has been researched several works of literature (Köroğlu & Eceral, 2017).
Accordingly, networking, technology intelligence and knowledge facilities research and development programs. For example, the need for 100-capacity air carrier encouraged Iranian investors to form a partnership with a consortium of companies. Offering its chairs in the transportation segment, the company cooperated with Ukraine to produce Antonov-An 148 aircraft through modern technology switch. Antonov-An 148 aircraft is an aircraft with over 100-passenger capacity.
Research and development allow firms to modify and expand air transport systems, navigation tools aircraft subsystems, communication tools, and crowd control units.
As a result, companies remove the adaptability barricades between organisational understanding and external benefits and or threats. Thus, aviation investors follow member initiative programs to acquire knowledge, review development or attract partnership contract. After evaluating the literary works on R&D, the thesis categorised the overall measurement into organisational readiness, absorptive competence and collective capacities. R&D influences partnership flexibility, communication, data transmission efficiency and storage.
Technology intelligence describes data pattern or information concerning crucial and significant designs, patterns, tasks and complications in technical settings. Technology is turning advancement at its centre because markets can apply originalities and prices that were impossible some years ago. Flight data and health status can be updated on an agency’s site and response transmitted without hassle. Repair routines, data analysis and maintenance records are readily available to preview, thereby raising performance efficiency. Research and development in aviation is a multidimensional approach that utilises advanced technology for innovative solutions (Köroğlu & Eceral, 2017).
Because of big data technology’s competitive nature, investors in product and service delivery are transforming air transportation via research and development initiatives. R&D initiatives have been witnessed in systems integration, legacy applications, business process harmonisation, product lifecycle management, manufacturing, customer service, critical systems, cybersecurity, business performance and competitive edge. R&D help in reorganising information architectures as it addresses a requirement for improvement in aviation. The challenges under this field include procurement lines, standardising methods, processing sequences, overhauling service methods, reorganise PLM bodies or customising the added-value network. Airbus has been driving for innovative changes in executing and implementing business solutions without service disruptions under this category.
R&D Activities
Honeywell aerospace procured CIMPA’s services to integrate material production and content creation devices for its technical paperwork, information resources and unscheduled repair guides. It is challenging to know that aviation firms wait for years to buy an aircraft component. This is an undesirable timespan for companies that need to speed up the pace of their fleet substitute. The aerospace sector, therefore, needs to improve its development. In developing its alternatives, Airbus approved a project to streamline the manufacturing method via a digital connection. It is a concern of utilising digital devices to go much more rapidly.
Airbus delivers this project in R&D and supply chain via its considerable business and technological experience. Rolls-Royce uses systems engineering to modify aircraft designs. The project utilises MBSE to structure units with life span or undergo numerous engineering changes. Lengthy creation timelines that include a raising amount of stability and functionality improvements create product differentiation. In MBSE, the production models operate with the system based on the approved concept. The abstraction subdues minor details but enforces precision in implementing the modified design (Köroğlu & Eceral, 2017).
The efficiency of MBSE makes it difficult to spot differences with over 100 MV-22 aeroplanes. Thus, systems engineering facilitates acquisition initiatives, design modifications, logistics strategies and supply posturing for change application with differing setups. Systems engineering centres on all-inclusive development to accomplish stakeholder requirement or demand (Lestiani et al., 2017).
Conventional or traditional production frameworks use content paperwork. Content documentation is preserved by developers and updated as criteria modification (Madni & Sievers, 2018). However, the modification of complex architectures and designs makes it challenging under traditional engineering systems. The authors argue that systems become intricate with huge work on the designers, and at such conditions, text documentation becomes inconsistent and unfinished. Text documentation creates misunderstandings with implementing demand requirement. In the situation where patent distinctions exist between different engineering disciplines, composed criteria might be misunderstood and result in the performance of a component that could be irreconcilable with the present setup.
There has been a modification in systems engineering due to complexity with text documentation procedures. Important details in production have sometimes be ignored due to the mode of storing patent instructions and command. Big data analytics mitigates these challenges by triggering the adoption of model-based systems. MBSE seeks to reduce the inconsistencies, specifically the end-user requirements and stakeholders involved in the system’s progression (Madni & Sievers, 2018). In MBSE, the model configuration becomes the dominant variable for administering item modifications or managing flight data. Kidd (2014) describes configuration control as an administration activity that handles the definition of an item or system based on existing interpretations. The FAA uses R&D to reinforce plan, organising standards development and modernisation of the aviation system.
Therefore, R&D sustains operations and the growth of NextGen. To accomplish equilibrium with timely deliveries, the FAA categories R&D in aviation under three headings of security, performance and ecological influence. To improve aviation security, R&D raises investor’s understanding to generate practical materials, gadgets, devices, or even techniques to enhance aeronautics safety and achieve the most affordable possible crash rate. To improve efficiency or productivity, R&D broadens technology utilisation to equipment production to enhance accessibility and aircraft capacity. Reduction in environmental impacts allows manufacturers to provide materials and prats that reduce aircraft emissions. The attribute has been widely discussed in several works of literature.
Aircraft Catastrophic Failure Avoidance Study (ACFAS)
The program sustains aviation R&D by designing techniques to determine threats and mitigate disastrous defects, failures, and malfunctions in aircraft, navigations, or equipment. The plan utilises chronological mishap records and FAA suggestions to evaluate turbine-engine activities and related influence. The R&D initiative builds component models connected with engine particles impact. Airworthiness System assists R&D by marketing innovations, treatment, technical information, and performance designs to reduce collisions and minimise incidents about commercial aircraft failures.
The initiative focuses on methodological and long-term repairs of rotorcraft’s structural stability, engine safety, electrical fitting, and mechanical components. The projects examine innovations like damages endurance and longevity issues of aluminium or lithium alloys, metal additive production and other emerging trends. The UAS program supports aviation by ensuring the risk-free, effective and integration of UAS in navigation designs. Aircraft sensors provide flight data to sustain certification methods, airworthiness criteria, functional needs, maintenance methods, and protection activities for UAS procedures (Lestiani et al., 2017). Investigation activities concentrate on innovation analyses, policy expansion, information assortment and production, industry and laboratory validation and innovation transmission.
Modern technologies such as IoT, sensing units, BD and AI have been used in several sectors to support, detect faults, and improve performance. Cheung et al. recommended real-time tracking based on modelling and a cordless sensor network to improve construction websites’ security. Present researches use IoT-based sensing units to examine the ecological conditions at a genuine site. Thus, the sensor information can be offered in real-time. IoT-based sensors provide a crucial solution for lots of study locations, including smart building and health care. Several research types have been conducted, and the outcomes showed a substantial need for IoT-based sensors to boost system efficiency.
Grekhov et al. (2020) proposed a monitoring system with IoT-based sensing units for smart edifices. The suggested system was executed in a simulation atmosphere. The results showed that a much better tracking system could be achieved by using several IoT-based sensing units. The proposed system is anticipated to improve energy performance and facilitate greenhouse structures. When a certain degree of radon gas is reached to protect against unsafe scenarios, the proposed system will raise the alarm to warn users. The suggested system was able to keep track of the radon gas degree, trigger the programmed actions, and notify the customers when a certain degree of gas radon was reached.
The authors recommended a modular indoor air quality monitoring system that accumulates several types of sensor information of gases, temperature, moisture, and chlorine. A single-board workstation was utilised to process the sensor data. The experimental outcomes revealed that the suggested system efficiently kept track of the interior air quality for several gases, temperature and humidity. Nazir et al. (2019) recommended a healthcare design using IoT architecture to discover and protect against the episode of ‘Chikungunya’ infection. The retrieved data were used to categorise individuals as clean or contaminated. The outcomes revealed that the proposed system could discover contaminated individuals and send out a warning alert to healthcare facilities to avoid further contamination.
The application of R&D in aviation improves service delivery and reduces the cost of operations. The performance of the suggested sensor architecture could function effectively compared to a desktop workstation. The results verified that the recommended IoT-based sensor was suitable for eHealth structures. Studies on the manufacturing market revealed significant benefits of IoT technology boosting working conditions, avoiding errors, enhancing medical diagnosis, and assisting supervisors with informed decision-making. Temperature level, humidity and CO2 information were accumulated transferred via cordless interaction.
Based on the speculative results, the authors believed the suggested system is durable enough, can gauge the environmental condition in real-time, and is expected to aid managers in preserving an optimum working condition. Zhang et al. (2021) recommended an environmental monitoring structure using IoT sensing units for error control and management during additive manufacturing production. The sensing units gathered data for temperature level and humidity. The research study disclosed that knowledge of ecological problems could prevent errors in additive production. The study utilised IoT sensors to gather information on errors in the diagnosis of mine hoisting tools. The findings show that IoT sensing units could aid give total diagnosis data along with enhancing diagnosis results. Steel casting was utilised as a real-case application of the projected structure. The suggested system could anticipate the product quality improve the procedure control.
Speculative outcomes verified the usefulness of the proposed system as it the migration to 4.0 framework. The quantity of IoT architecture deployed in the investment sector significantly. IoT allows the shift from local structure to modern digitalised production. As the variety of gadgets collecting sensing data rises, new applications’ capacity to manage varying sensor data increases. Ge et al. created a theoretical structure by incorporating BD innovation in IoT, which support decision making. Using BD processing, the enormous quantity of data gathered by heterogeneous sources can be managed and evaluated, thus assisting managers with better decision-making.
The manufacturing industry is experiencing a boost in data generation with tons of data generated from one flight operation. Developments such as machine learning offer insights to examine databases and offer administration assistance in decision-making to improve system efficiency. Systems like Random Forest (RF) have been used to predict decision trees. RF operates by using the bagging approach to produce parts of training information.
The final prediction result is chosen based on the bulk ballot over the learning algorithms, which can minimise the category design’s precision. Outlier discovery can be used in the pre-processing action to determine incongruities in data outliers. Such record makes the system an effective classifier for decision-making. The crossbreed prediction model is incorporated with a real-time processing system, allowing the sensing unit data from IoT-based sensing unit gadget and fault forecast in real-time. Studies have shown the adoption of IoT sensing units, AI and machine learning designs to determine the environmental problems online (Sadiku et al., 2020: Zhang et al., 2021).
Therefore, the sensor data can be presented in real-time using BD technology. As the number of gadgets collecting sensing unit information in production increases, the potential for new applications that can handle the input of large quantities of sensor information such as tremendous data innovation rises.
Big Data Technology
Big information (BD) describes complicated datasets that cannot be processed by conventional techniques. The researchers analysed intricate data collection based on client service delivery, flight hold-ups, rescheduling, ticket sales, and on-hold booking process. Marketing methods have been competitive, and airline operators produce loyalty programs based on offered information on consumers’ demands. Big data help with precise analysis to avoid overcrowding. While this innovation is managed throughout various platforms to improve the consumer experience, airline companies may obtain a much deeper understanding of competitors’ customer requirements and trade details.
This improvement of the technical and analytical approaches permitted airline companies to deal with clients’ concerns and gain vital insight into their competitive environments (Alt & Zimmermann 2017). By recognising clients and the competitive environment, airlines might increase their consumer satisfaction and acquire a much better grasp of competing activities while implementing brand differentiation strategies. By examining the position of competing events in digital change, commercial airlines may likewise assess their flaws from the digitalisation process and make changes. The aviation organisation is going through extreme growth, which will require airline operators to search for the very best resources to function as an ever-expanding sector (Vranken 2017). The majority of financiers are modifying their organisation designs to acquire a competitive edge.
The aviation sector handles and develops client info utilising digitised architecture. Many airlines cannot control and process the number of details they gather from stakeholders and passengers. Based on this assumption, Big Data has ended up being an expansion tool that links and examines data inputs to improve service delivery. This innovation has produced the concept of a service-oriented design that supports service advancement (Vranken, 2017).
Information acquired from the engine display unit allows effective predictive maintenance. The fuel tracking system could be analysed to develop effective and effective fuelling choices. Weather condition information may be utilised to establish how different flying conditions impact engine operation and select the most reliable mission route. System tracking data might be used to determine upgrade priorities. These operations support profits management, curated travel experience, and service efficiency. Data info is created based upon historical occasions and activities. The technological transformation has made it feasible to collect, store, and analyse considerable information.
With numerous provisions to produce data, datasets grow exponentially, with the conventional techniques accessible to analyse and save info ended up being inadequate and requiring. Airline companies produce and handle complicated data and data registry. The possibility to manage different flight towers utilising BD systems highlights the significance of digital innovations in improving the consumer travel experience and communication channels.
Information management enhances service effectiveness and reliability through the optimal utilisation of navigation facilities. Big data identifies a massive volume of information generated in a high pace by many resources. While processing this information, routines, insights, and relationships are accessed, which is impossible with restricted data. Many organisations are utilising external and internal to create better, brighter, real-time conclusions giving them a definite edge over rivals. This benefit grows as more information is generated.
The aviation industry is undergoing an expansion that mandates airline operators to search for better instruments to function in the expanding sector. Some airline operators are considering altering their basic business models. Schwartz (2014) asserts that digitalisation improves flight operations, reduces flight cancellation, and delays. Digitalisation promotes efficiency by encouraging the implementation of system sequences between flight controllers and pilots. Scherer, Wünderlich, and von Wangenheim (2015) believed that big data technology promotes a new reconnaissance technology.
The implementation strategy supports an efficient air transport system. The new platform will replace the present sensor systems using a satellite-based infrastructure to guarantee security identification with precision. Lee and Park (2016) believe that digitisation and big data applications play a significant role in sustaining small carrier investments. The low return on investments is a substantial challenge for the same carrier airports. However, data applications’ availability eases these airports’ remote management, with its controls positioned in bigger airports. Phillips (2016) suggested the possibility of air traffic personnel using efficient navigation systems to fly and land aircraft in smaller airports. Such prospects translate into high levels of consumer fulfilment because of service expansion along local routes.
The possibility to manage different flight towers using data applications illustrates the significance of digital technologies in improving the consumer travel experience and communication channels. Padrón et al. (2016) stated that digitalisation provides backup to aid contingency planning during sabotage or emergencies. Thus, big data improve service efficiency and reliability via the optimum utilisation of data infrastructures. Market dynamics triggers innovative designs and equipment. As a result, the research explores big data trends as it supports innovate architectures in aviation.
Big Data Trends
Business intelligence (BI) is a concept of using specific strategies to transform information into relevant information. Mostly, the term business intelligence has two different implications, which include human knowledge and learning. The deployment of human insight and innovations is utilised for administration and leadership in various business-related issues. Architects and software engineers still use business intelligence (Søilen, 2015).
BI is explained as structures that build-up, alter, and present information from different sources while reducing the anticipated time to gather business data and empower their productive use in PM. The main goal of business intelligence is to empower supervisors to evaluate data and have the capability to lead the investigation process (Gauzelin & Bentz, 2017). BI enhances dynamic data analysis, business recovery, analysis, and interpretation of regulatory decisions’ necessities (Søilen, 2016). Søilen (2016) demonstrated that BI focuses on a social event, process, and presents data concerning clients, competitors, business areas, innovation, and items. Fourati-Jamoussi and Niamba (2016) describe BI as a methodology that joins a progression of exercises, driven by decision-makers’ specific data needs.
BI is a structure that changes data into information and after that into knowledge, thus upgrading the organisation’s operations and management process (Kurnia & Suharjito, 2018). Consequently, business intelligence can be described as a framework and a response that causes decision-makers to fathom its financial status (Kurnia & Suharjito, 2018). BI assists managers by separating data from different administration assets at a strategic and business level (Kurnia & Suharjito, 2018). The development of business intelligence has created new metrics for data measurement. Thus, hazard management and management strategy are influenced by business intelligence.
BI enhances business performance by utilising data mining in various ways. The interest for more complex and sophisticated systems is growing because storage capacity develops as data increases. This uneven development relationship influences data processing time using traditional business intelligence frameworks. There are assortments of innovative data procedures that help BI frameworks to run effectively. The process of applying business intelligence for a business issue is called the KDD. This procedure is crucial for effective data mining operations (Bhanap & Kawthekar, 2015).
Darma (2016) studied the connection between BI systems and the decision-making process. The author surveyed fifty-four business managers. Data were coded and analysed using regression analysis. Based on the outcome, the author revealed that quality of BI systems influenced the quality decision-making process. Sadiku, Tembely, and Musa (2016) discussed approaches in business intelligence. The researchers gave an account of how organisations are utilising business intelligence to enhance the adequacy of decision-making. Business intelligence is essential for investors to examine and establish their brand.
The authors concluded that business intelligence plays a role in a competitive environment. BI has become a critical plan for administrators since they are mindful of its incentive in giving an aggressive differentiator at all levels of the organisation. Business intelligence collects and evaluates valid information while applying the outcome to different techniques. Business analytics involves the use of abilities, innovation, and calculations of data mining. Thus, it delivers valuable data to help investors and administrators settle on better decisions concerning their investment and have legitimate control of their operations.
Efficient systems for online transaction processing (OLTP) contribute to the advancement and acknowledgement of relative innovation data safety, recovery, and knowledge management. Chen and Piani (2017) reviewed organisation intelligence with data mining applications. The paper discussed the ideas of business intelligence, data storage, and its impact on efficiency. A research study of business intelligence systems for developing data storage links was introduced and dissected.
Patil (2017) revealed that the primary engine driving business analytics in businesses is DM or KDD. DM provides a perspective of previous circumstances, and a comprehension of the likely future results, stimulating successful outcomes. Therefore, clusters are created by analysing the clients’ conduct of trade, product choices, and buying behaviour. Straightforward extrapolation is utilised to describe the concept of data mining.
Besides, clients gained helpful and adaptable data access through query languages, UIs, the streamlined inquiry process, and management. Application-based data frameworks, text mining, spatial data mining, web mining, multimedia, dynamic sensor, and logical databases have also prospered (Maksood & Achuthan, 2016). Issues related to the transfer, diversification, and information sharing have been extensively considered.
Therefore, acquiring information from Internet users requires effective approaches. Content-based frameworks, structure deep net querying, and web link clicking facilitate this practical approach (Maksood & Achuthan, 2016). Organisations use data mining for the development of business through disclosure of useful examples. Thus, questions enable us to recover data of which have a pre-knowledge, while DM encourages researchers to find complex realities in the databases.
Information mining and intelligence support business analytics. Business analytics includes the use of abilities, development, and calculations of information mining. There are two functions of company analytics; the back-end where the first usage of information mining occurs and the front-end reporting measurements. Queries recognised with information in different information shows software enables researchers to extricate valuable information (Bhanap & Kawthekar, 2015).
Data trade has witnessed several phases in data accumulation, information administration, and intricate information examination. The assessment and development in data frameworks given that 1970 has advanced from stratified data frameworks to conventional database systems, information modelling instruments, and evaluating treatments (Bhanap & Kawthekar, 2015). Blockchain is a secured and connected pc registry with a growing database. The journal records are processed and handled by cartography. Blockchain innovations can function as the technical basis for transactions, trade, financial investment incomes, electronic source transmission, clever investment, and implementation (Tapscott & Tapscott 2017).
Underwood (2016) studied Blockchain innovations and listed the advantages of its homes. These benefits are related to supply, promo, and its characteristic feature. Because Blockchain is dispersed across several geographical locations, it does not have a central database. The distinguishing function of Blockchain technology gets rid of the risks of the main database, and its openness supplies effective versatility.
Blockchain enables a virtually real-time authorisation of listed transactions, which decreases the transfer intervals of payments. The capability to digitise and digitalise a financial investment portfolio is distinguished by deploying Blockchain innovation. Blockchain technology empowers an organisation to allocate external and internal resources in reducing trade times and costs of operations. The technology relieves insecure or difficult trades because of the lack of payments of obtaining trust. In deploying Blockchain innovation to improve the travel experience, airline companies may get more substantial cost economies, bolstered security, and effective resource allocation options.
This innovation resolves the security-related concern of airline company operators and a substantial influence on the airline company market’s costs. Additionally, embracing this innovation a significant one-upmanship for the organisation. The most notable result of welcoming Blockchain is its impact on complete customer satisfaction using a better client loyalty strategy, which considerably enriches the airline’s brand name awareness (Ebarefimia 2017). The finding is an extensive survey that examined the effects of the use of Blockchain innovation in aviation. A favourable connection has been found between Blockchain engineering and boosting passenger satisfaction (Ebarefimia, 2017). For that reason, Blockchain implementation in aircraft applications enhances the customer’s travel experience.
AI combination in the air travel market has been disruptive as it changes the company technique towards its operations and profit maximisation. As airline companies drive growth through fleet expansion and technology, they seek to improve the consumer’s travel experience by enhancing efficiency and preventing human mistakes. AI technology revolutionises the air travel market with an accurate and precise mode of operations utilising device knowing. Machine learning has been adopted in fleet operations control, customer service, passenger retention, and machine processes. Under fleet operation management, AI has been deployed in dynamic pricing, pricing optimisation, flight rescheduling prediction, fraud detection, crew scheduling, and customer service. Augmented reality (AR) is a practice that evokes the composite view of a real object with virtual articles.
Palmarini et al. (2018) described AR at the attribute of blending virtual and actual posts in a real-life environment and the capacity to operate in real-time. AR engineering in the aviation sector is modelled to build eccentric interfaces using wearable visualisation methods to present information as electronic and graphic databases (Palmarini et al. 2018). According to Palmarini et al. (2018), conventional repair techniques cannot be used on contemporary aircraft and need to be upgraded to manage the intricate procedures and avionics.
By integrating AR design, maintenance workers could perform complex jobs without handbooks, which frequently contribute to frustration and error (Palmarini et al. 2018). AR could reduce MRO personnel’s training time because it has been demonstrated to decrease the compulsory manual maintenance jobs. Using advanced AR innovations, understanding the operator’s environment transforms into an interactive environment (Palmarini et al. 2018).
The use of AR innovation for aircraft repairs and maintenance has been extensively accepted and helpful (Palmarini et al., 2018). By ramification, the quality of MRO on aircraft operations increases flight efficiency and customer experience. Automation improves efficiency and security, and airline operators are cautious of the threats of using automated functions. Airline automation changes the process of task accomplishment by introducing new synchronisation needs of the operator. Automation is the machine implementation of works once achieved by people (Noy, Shinar & Horey 2018). Automation innovation has been adjusted in-flight cabin, seat reservation, staff lineups, and ticketing. Automation has become a radical technological enhancement in aviation because it boosts functional performance and flight security (Noy, Shinar & Horey 2018).
Automation technology has been adapted in-flight cabin, seat booking, staff rosters, and ticketing. Automation has become a radical technological improvement in aviation because it enhances operational performance and flight security (Noy, Shinar & Horey 2018). Automation positively affects decreasing workload and raising task efficiency (Washington, Clothier & Williams 2017). Although automation improves performance and safety, airline operators are wary of the risks of using automated functions.
RFID is a remote sensor used to distinguish tagged objects or individuals. RFID innovation distinguishes friendly planes and ships during World War II. The sensor track assets, wildlife and human observation. Tags vary in sizes, shapes, and abilities and could be dynamic and latent. A dynamic tag contains a battery, the energy that works the tag. The device’s fundamental motivation is to connect with the tags and pass the tag information to a host workstation.
The information collected by an application can be a distribution centre framework, database, framework, or hidden flaws to mention a few. Web mining gives dominant methods to help individuals understand the user experience by finding customer’s behaviour patterns. These patterns can be utilised to assess the user experience. Using conventional strategies such as statistical analysis and data capture techniques, researchers could gather user information, but it is difficult to evaluate the user experience (Jin & Kim, 2018).
Consequently, the online weather service organisation needs viable strategies that empower them to find the hidden knowledge. Approaches like surveys and interviews can help organisations obtain the user experience; however, the process is costly and time-consuming. Consequently, the feedback strategy could be used to collect data, but its usefulness is restricted. The organisation needs a considerable measure of time to discover valuable data from the analysis. Data mining innovation gives appropriate and productive techniques to understand the user experience. Given the discoveries of data mining, web developers can improve customer experience and deliver more customised services (Nguyen et al., 2018).
Web mining techniques give helpful knowledge to enhance the user experience. The clustering method assists organisations by segmenting valued users to offer them customised content based on individual attributes. Web mining improves the connection structure of the website and its content design. Flight data generation influences human activities and enables managers to comprehend travel routes and investigate it more profoundly. With the enhancement of the customer information level and the difference in customer satisfaction, individuals want more differentiated items, customised services, and lower cost of products.
Web mining has become exceptionally crucial for online presence management, making versatile websites, business, and service personalisation. The development of business intelligence has exposed metrics for data measurement. Therefore, BI enhances business performance by utilising data mining in various ways. The interest for more complex and sophisticated systems is growing because storage capacity develops as data increases. Thus, risk management and management strategy in aviation are influenced by business intelligence.
The principle of big data and predictive analytics has reverberated around sector conference rooms for years. Just recently, the information acquisition from aeronautics infrastructures and sensors installed on aircraft became the focus in different aeronautics areas as service providers, airports and aviation investors brainstormed on possible uses. The volume of data extracted from these sensors and systems requires a complex analysis that cannot be done under traditional computing capabilities (Choi et al., 2018).
A queuing design that uses an actual arrival timetable as input but mimics arrival delay based on readily available arrival ability is adopted to estimate hold-up decrease possibility of slot controls. The verdicts show the possibility of slot controls to reduce delay at airports and its consequences. The results prove the impact of big data in solving the challenges of traditions queuing models. The evaluation results were significant, showing that the system could be valuable for airport terminal authorities to conduct entrance tasks. Delays at airport terminals have become a growing concern for investors, leading to flight delay or cancellation in most scenarios.
Consequently, investors are conducting individual research using big data technology to improve queuing issues and enhance service delivery. The outcomes show that down-line routine disturbances are symmetrical to the number of flights influenced by the GDP. In the documented ground delay program (GDP), aircraft management appears to be the factor for many flight delays anticipated by the system. Weather condition outcomes are substantial under VMC. Temperature, exposure, and wind showed considerable impacts in at least one of the moment durations. The existence of these weather condition impacts suggests that the versatility of VMC is a lot more receptive to altering problems.
The current practice of measuring flight delays in the benefit-cost investigation is deficient in establishing an innovation change’s distributional influences. The framework specifies various classifications of hold-up per flight and procedure for computing each hold-up’s expense by stakeholder recognising that the airline companies have various business strategies and therefore have various preferences. Scholars highlight the significance of the human knowledge needed to examine patterns to develop, organise improve understandings (Jin & Kim, 2018). The findings show that experts must establish methods to get insights from big data technology, albeit structure on mathematical estimations. Human intelligence could be deployed with big data designs to remove the reliance on robots and AI. The author agreed that individual influence could regulate the need for big data innovations.
Several academics have considered the impact of big data, and actors use these understandings in social space. Although the experts use empirical data to verify BD impact, theoretical arguments exist in his field. Furthermore, it is unverified whether the BD impact disputes are consistent with the findings in different literary works. The dispute around human intelligence and algorithmic aptitude seem to exacerbate the need for balanced research.
It has been argued that he value of details processing systems and the inclusion of that make up the various other fifty per cent of the system. Likewise, modern technologies’ duties in automating complex systems have been examined along with the associated difference between human intelligence and smart workstations. The assumption suggests that it remains to be seen whether BD supplies modifications that differ from what various other innovations such as knowledge monitoring systems have currently produced. On this premise, it remains unclear under what specific problems business players can generate understandings via inductive or logical methods, or a mix of both.
Used Cases and Decision Making
The requirement for evaluating data has developed concept and adoption of big data technology, which supplies accumulative value and knowledge. Such information must be used appropriately and data resources defined. Big data technology adapts fast properties to transmit unstructured and codified data for analysis. The system categorises these phases into formula planning, data analytics and studying. The approved formula or model is chosen, adopted and used further evaluation.
Consequently, the decision making procedure is the choice phase, where techniques are utilised to examine the impacts of the recommended solutions or strategies from the formula stage. As the volume of big data expands, organisations are becoming much more curious about how to manage and evaluate such information. Hence, business experts are rushing to seize the opportunities provided by BD for-profit and insight to unlock financial value and enhance faster decisions. For that reason, companies are transforming towards BD analytics to assess substantial amounts of information much faster and reveal unobserved configurations, ideas, and customer intelligence.
BD can be used to forecast demand modifications in aviation and accordingly match their supply. By assessing products usage, maintenance reports and geospatial information on supplies, organisations can automate fault repair decisions, which will certainly minimise downtimes lead, flight cancellations, faulty engines, high operations costs and procedure interruptions. Additionally, replacement cost computation could be arranged concurrently, which could enable reductions in operating expense and a rise in revenue margins. BD technology can detect the cause expenditures and offer a far better solution to mitigate occurrence or prevent overdue repairs at a higher procurement cost.
An additional area where big data analytics can be of value is efficiency administration, where the aviation investors, suppliers, and manufacturing shareholders could benefit. With the rising requirement to expand productivity and efficiency, performance information can be checked and utilised analytical tools. Specifically for the manufacturing, telecommunications and aviation sectors, big data improves monitoring to maximise cost and lower expenditures.
In the maintenance process, BD analytics decreases performance irregularity and mitigates value problems by providing early caution alerts. The design reduces scrap rates and logistics delivery by assessing disruptions to the manufacturing process before they occur. Besides, real-time data evaluations and investigation enable supervisors to make swifter choices for high-quality monitoring. Likewise, BD enhances quality management by predicting customer requirements and demand. Accordingly, big data offer smart transmitting, according to real-time website traffic information based on individual information.
Industries such as financial investment or retail financial, gain from big information analytics in the location of threat administration because the analysis is a crucial element for investments as BD aid in choosing financial investments by analysing the chance of gains versus the likelihood of losses. Additionally, the network sources of BD can be analysed for appraisal of direct threat exposures. Accordingly, BD can benefit companies by allowing the metrology of threats. Performance analytics can be utilised to incorporate the risk profiles handled in isolation across different divisions and threat accounts. The strategy aids risk reduction since a detailed view of risk factors is given to decision-makers.
Additionally, BD tools can manage the rapid growth in network information and lower data challenges by increasing the capability to scale and store investment data. In addition to improving cyber observation and data-intensive remedies, organisations can incorporate streams of data and computerised analyses to protect equipment and workstations against attacks. Fraud detection department deploys BD to resolve sharp practices and detect unauthorised transactions. Analytics are utilised in automated detection. However, companies are harnessing the potentials of data mining capability and artificial intelligence to enhance their systems.
Stream computing describes the handling of enormous data from numerous sources with low latency in real-time. New sources of information producing situations, which include universality of area services, mobile tools, and sensor prevalence, a new paradigm necessitated. It can be related to the high-velocity circulation of information from real-time resources such as IoT, sensors, transaction data and mobile data. The essential presumption of this standard is that the prospective worth of data lies in its sparkle. Thus, data are evaluated in the stream to create outcome as opposed set computing where data are first stored before they are investigated. There is a crucial need for identical designs and scalable computing platforms. With stream computing, firms can evaluate and react to changes in data. Streaming handling structures include S4 or Spark.
BD streaming’s essence is to react and evaluate data using constant inquiries to enable analysis within the stream. Big data stream comprises an adaptation of data as an immeasurable tuple, evaluation and assembly of variable outcomes. In a stream workstation, applications are denoted as information circulation chart comprised of operations and interconnected streams. In a streaming investigation framework, applications are collected in unstructured queries that are examined, classified and analysed. Streaming applications are a bunch of administrators associated by streams. Streaming frameworks should have the option to distinguish new data, fabricate models and access whether the new approaching information varies from model expectations.
Streaming analytics relies on the assumption that all unstructured information is handled in the data hub. Such handling incorporates eliminating copies, filling missing information, information standardisation, parsing, and extraction, which are done in a solitary design due to high information paces of streams. When another stream shows up, the processing hub is activated to remove older tuples as indicated in the sliding window. A window is alluded to as a coherent holder for information tuples acquired. It characterises how habitually information is invigorated in the compartment and analysed.
BD stream is significant when there is a need to acquire valuable information from current happenings in a productive way to empower associations to rapidly respond to issues or identify new patterns, which improves performance. Notwithstanding, there are difficulties, for example, adaptability, coordination, adaptation to internal failure, idealness, consistency, heterogeneity and deficiency, load adjusting, protection issues, and precision which emerges from the idea of data streams that should be managed. The FAA is keen on comprising the enormous information pool in aviation framework.
BD framework characteristically requests association with other datasets that are not identified with the offered dataset to make the shrouded information. Since associating two datasets is a troublesome assignment, the association’s undeniable advantage ought to be found. Businesses and aeronautics networks are examining the advantages and coordination capacity (Valeika, 2016). Data researchers frequently need to control the dataset for investigation. Nonetheless, the immediate control of scripting in BD framework is troublesome because of its scale (Fisher, 2012). Third, the representation strategies of scrutinised data with many factors in the enormous information framework should be considered.
The representation of huge outcomes may not fit in a normal size screen and requires complex display procedures. Govindan et al. (2018) recognised the issues of representation in BD applications, including visual commotion, enormous picture insight, data misfortune, performance demand and the high pace of picture change. Smart designs have countless applications in the aviation market. For example, business owners could track food trays in resort dining establishments, and workers would be informed whenever plates are left in the bar or guest rooms. The pervasive nature of big data enables the application of smart devices and intelligent assets. Big data application combines with innovative designs to improve service quality and safety. Depending on the case scenario, BD could become the mainstream of aviation investment.
Cargo vehicles could be offered best routes based on real-time web traffic info raising gas efficiency, delivery time, and reduced delivery costs. Investors could harness business profit using the appropriate smart device, which is powered by big data technology. Infrastructure could additionally be equipped with smart sensing units. A recent innovation is smart designs that showed the possibility of developing concrete casting with sensors that give security purposes information. The smart device allows investors to conduct operation within the no-fly wing as the concrete slabs relay movement and body heat to detect unauthorised movement.
Consequently, the smart device generates maintenance data required to sustain the strength integrity of the building. Automation is a significant impact on aviation workforce. Up until now, media attention on this subject has concentrated on concerns that robots and artificial intelligence could displace human intelligence. Nonetheless, a Next-Generation labour force’s production, requiring humans and smart devices, will be a crucial trend.
Developments in robotics, AI and IoT would create safer skies, prevent flight delays and enhance service delivery. An automated workstation supported by an attendant powered by online intelligence could offer cafeteria pointers based on their social media control. The capability of individuals and smart devices to cooperate properly would support the goal of the NextGeneration workforce. Capital funding and synergy with technologies would enhance employees’ ability to provide a spot-on assessment of data retrieved from flight cabins, weather details, and other information. Technologies such as VR will support the labour force by enhancing aircraft maintenance routine and update parameters.
The findings from several literature works acknowledge that big data empowers individuals to become creative, transform unstructured data to aid business intelligence and enhance service delivery. Digital transformation would reduce dependence on low skilled personnel and improve service delivery. Although big data may not replace human personnel, the rate of human errors could significantly affect business performance. Therefore, low-skilled employees can be encouraged by modern technology to execute complicated tasks.
Educating programs to train workers will be vital to preparing the industry’s staff for the adjustments ahead. Partnership and data sharing within and outside the terminal will support campaigns that improve demand forecasting and optimisation. Successful cooperation between airline owners, airport workers, non-airport staff, taxi drivers, and the ecological community would greatly benefit the industry. Therefore, joint efforts will be required among shareholders, policymakers, aircraft producers, data management administrators and cybersecurity experts.
Security is among the leading concerns for travel, tourist and air travel, specifically increasing national instability. The level to which clients are ready to trade their personal space for more security will determine how security will develop. Clients are ready to share their information as it allows airline management to enhance their security. The modern-day security environment will enhance security and must make these issues disappear.
Evaluating innovation such as biometrics, sensor scanners, X-rays machines, and automated target acknowledgement will enhance security checks’ effectiveness and efficiency. Security officers will be supported by video analytics to evaluate crowd behaviour and feelings, and facial acknowledgement innovation to find recognised wrongdoers in a crowd. These innovations produce a virtual safety perimeter, which is not reliant on physical checkpoints. An interoperable travel identity between federal governments, airline companies, and airports worldwide will streamline information architecture, enhance service quality and make workstations less susceptible to failure.
Consumer mindsets to making their information readily available for security and security plans are essential. Clients should discover the proper balance between the threats of providing up some of their privacy and the advantages of increased security and security. Security and security are an international cause, specifically in the battle versus terrorism, and partnership between authorities can assist the secure environment. The success of the innovations pointed out in the area on common traveller security depends on the development of global data trade between airline operators and security services.
Using biometrics, body sensors, cameras, and facial acknowledgement innovations to increase checkpoint security raise ethical and health issues. Customer perception towards noticeably intrusive security steps differ significantly. Offering the proper details of security screening might relieve some of these issues. Empower instructional organisations to develop programs that prepare employees to operate with smart innovations. Since data stimulate market performance, a multi-stakeholder technique covering public sectors provide regulative structures that specify the proper usages of tourist information. These structures will specify who owns the information, who can utilise such data, and secure its database.
Clear interaction from the business’s management on digitalisation can motivate and fight this inertia approval of the digital change. Numerous air travel, travel, and tourist business focus on their relationship with clients and direct their digital financial investments to sites and mobile user interfaces. Nevertheless, it is vital not to disregard operations and acknowledge the huge capacity for performance and expense savings that innovation can bring. All stakeholders should expand digital categorisation and enforce proactive actions to optimise socio-economic benefits. Digital technology provides chances for aviation’s extremely competitive market. However, optimising digitalisation’s value rests on the tourist, travel, and investor’s capability to work cooperatively.
Discussion of Results
The thesis considered specific areas of interest as it relates big data in aircraft. The researcher discussed technologies used cases, applications, product and supplier chain, research and development, and big data trends in aviation to answer the study objectives. Several works of literature were reviewed and content analysis summarised to draw recommendations and conclusions. Importantly, aviation and its attending technology drive some aspects of passenger behaviour and desires, global trend and ecological worries. Digitisation develops new difficulties, not fully comprehended.
The impact of digitisation allows companies to exchange trade data through safe and secure innovations. Digital technologies will enhance consumer knowledge and travel experience. The governing structures pressure significant influence on change and could disrupt new technology designs. Innovation spreads faster than protocols, suggesting that authorities are pushed to launch laws for incipient modern technologies. The issue with bogus news on social media shows the threat of not approving the new digital patterns or resolving relevant prospects and challenges. Aviation, especially airlines, records low-income margins due to poor market capitalisation versus technology business and rising customer expectations.
Big data has resulted in every sector ranging from power, agriculture, mining, aviation, healthcare and their links. Aviation is a significant sector that has viewed substantial enhancements with Big Data. Flight data analysis has improved client satisfaction, strengthening the stability engines and prospective revenue. Presently the air business is not at the peak of its performance. However, the influences have been felt. For example, a Norwegian service provider infused big data in telephone call facilities to permit behaviour analysis and evaluation.
Creating this kind of innovation would strengthen client associations, boost revenue and enhance productivity. Consequently, Delta investors are equipping their log cabin staff with intelligent gadgets utilised primarily for on-board investments. The technology has been influenced by smart technology in aviation. Most airline companies have triggered comparable ventures to improve and enhance service delivery. For example, Tunisair, British Airways, Emirates and other investors are designing similar strategies to enhance passenger travel experiences and improve safety. When passengers are transported from one location, big data suggest that the aircraft is under no threat.
Such information is gathered from the ground control centres, air traffic unit, and tower sensors. Consequently, the terminal beacons and internal navigation systems report inaccuracies on an aircraft via a continuous communicating channel. By implication, the aircraft’s health status is monitored and observed from several locations. The capacity to detect fault lines and relay these messages to the appropriate department determines the impact of big data on aviation. Along with the correct locations and data sensors, information transfer would control business investment. Big data information analytics has become a concern for airline drivers.
The idea of utilising information to enhance company choices recorded the spotlight, specifically along with the number of records generated per flight. Data generated from systems and subsystems enhances predictive servicing designs to detect components capabilities and compute maintenance routine. Consequently, climate records allow forecasters to identify risky routes and provide balanced confirmatory messages with other transponders from the flight cabin.
Designers use aircraft engineering to verify, identify and observe how devices operate, factors affecting their system and methods to improve their performance. Post-delivery records are reports by manufacturing companies after delivering an aircraft. The records are passed to the service personnel for reference purpose. Sometimes such details or records are misinterpreted or dumped by inexperienced service personnel. Big data connect the gap in recording keeping by provided advanced platform for documentation and evaluation.
Big Data and Service Quality
Web mining is a component of data mining that allows analysts to separate valuable informed from the Internet. The authors concluded that web-mining algorithms play a distinct function in data collection and analysis. As a typical service test, the service provider and customers’ gap is reflected in the low customer commitment. The gap can be closed when services are viewed as a voyage or a cyclical progression of experiences over channels (Mebrahtu & Srinivasulu, 2017).
Subsequently, the consumer journey map is an instrument that fixates the customer’s past and present experience after utilising an item and buying or service. As a result, the consumer journey map can be an ideal device for examining customer loyalty and experience. The tool is utilised to create imaginable options to enhance the value or decrease exercises that adversely impact consumer fulfilment. To acquire insight into Internet-based weather service’s contribution and visualise the customer’s experience, the researchers mapped the user experience in the analytic tool. Quality involving operational measurements and service staff members is crucial for accomplishing an airline’s one-upmanship through distinction (Rahim, 2016; Hapsari et al., 2017).
Khanh (2017) proposed that service measurements could be security, comfort, in-flight conveniences, disposition of the ground and flight group, financial strength, on-time execution and luggage conveyance. The author’s recommendations include on-time execution, air terminal registration, flight facilities, seating arrangement, solace, seat area, airline company interior decoration, post-flight services, nutrition service and client programs (Khanh, 2017). Haghighat (2017) verified that the airline service qualities could segment travellers. The researchers determined five parts, which include applicant value, quality explorers, comfort-oriented clients, time-oriented travellers and specific specifics.
Airline managers package their service based on the first class, company visitors and economy seats. Business travellers have special needs and requirements, and they have esteemed more than economy class travellers (Hapsari et al., 2017). The variables of quality and service are significantly related. Researchers find it hard to discover if the customer experience is an item of service quality or vice versa. As Joudeh and Dandis (2018) suggested, the service practice evaluation is affected by the traveller’s perception of the service provided. Thus, client division is necessary to the airline company business, and studies suggest that long-standing consumers have higher service quality desires, which depend upon the flight experience.
There could be contradictions regarding the link between service quality and client experience. In simple terms, service quality is a combination of events offered to a client, while consumer experience reveals the individual in accepting the service. To assess the online weather service’s capability, a critical phase of the analysis is to determine the online weather forecast size. However, there is no metric to demonstrate information about weather services. Therefore, researchers and weather experts rely on articles and web journals from financial gathering to discover proof to evaluate online businesses’ size.
Consequently, weather experts evaluate organisations to find the pattern of weather service development. During this procedure, they considered the attributes of those organisations and the emphasis of their development. Given the findings, the authors distinguished a few patterns of service development. With the tremendous growth of data accessible online, the Internet is a stage for storage and information transfer. Because of the heterogeneity and unstructured nature of data on the Internet, information search is lumbering and tedious (Maksood & Achuthan, 2016). As a result, web mining was introduced to solve the challenges of data collection and processing.
The utilisation of big data applications in airports relieves these services’ recognition because many customers are not acquainted with the place. Chen, Batchuluun, and Batnasan (2015) argued that the recognition of passenger’s needs using data applications supports airports’ multi-functional environment. Many airports offer clients services, including restaurants, shopping centres, and retail outlets as customers’ travel to their destinations. The utilisation of data big applications for navigation in airports relieves these services’ recognition because most clients are not familiar with the area. Access to varied information applications increases the identification of service areas that affect passenger complete satisfaction. Thus, airline company investors are accepting BD technology to assist in more uncomplicated check-in to their clients.
Jeeradist, Thawesaengskulthai, and Sangsuwan (2016) emphasised that information technology has substantially reduced the idle time invested with customers awaiting manual check-ins. Digital applications have been used to integrate the commercial and customer support services at airports, making it suitable for guests to access functions readily available in the airport from clever gadgets, tablets, notebooks, and other electronic systems.
Such programs act as an interactive interaction platform for customers, in which they might participate straight with air travel workers to send inquiries, remarks, grievances, or concepts. Khuong and Uyen (2014) stated that airline operators’ availability of services via electronic interaction innovations implies a traveller might get ready for a trip from any geographical location. By implication, a passenger may have access to different airport services when trapped in traffic (Skorupski & Uchroński, 2016). Such procurements could be sent to the tourist throughout aircraft boarding, thereby decreasing idle times and improving gratification to unique client segments.
Digital communications in airports have been crucial in improving passengers’ curated travel experience because it promotes more simple and economic interaction involving airport workers and customers. Electronic and cloud-based technology at airports increases virtual capacity production in airports, thereby boosting property optimisation. Lee & Park (2016) additional assert that the much more comfortable control of lead times furnished by strategic data applications at airports improves launch events, which empowers assistance shipment to a more significant number of travellers.
Big Data and Aircraft Maintenance
The development in predictive maintenance enables operators to anticipate when a specialised error will appear and intervene to fix it (Tokgöz et al. 2018). Predictive maintenance decreases running expenses and boost company advancement (Tokgöz et al. 2018). It offers detailed and predictive insights to boost organisational operations. Milkau and Bott (2015) defined strategic maintenance as incorporating complex systems, internet-based innovation, and info analytics to interact between consumers and market representatives. Some airports utilise legacy programs, digital data technique boosts service availability, employee performance, and enhances safety.
It stresses the requirement for airport operators to embrace applications that utilise cloud options as a backup technique. The rising competition, advancement, and alliance of allowing innovation promoted modification from the global perspective. The airline 4.0 paradigm execution is an essential step in developing companies, which depends on digitising information and practices (Zangiacomi et al. 2017). The strategy to adopt ingenious innovations will stimulate data flow through different distribution chain levels, allowing the communication between the stakeholders and guests.
Digital architecture now represents the most innovative paradigm change in the airline company market, covering the complicated, cohesive style of products, making systems, and processes. Digitalisation aids accurate assessment and usage of the information from different phases of the service lifecycle. The technology implementation provides delicate information to command, improve services, dependability of the makers, demands from clients, and parts circulation (Zangiacomi et al. 2017). It is vital to gather data with information management to support its utilisation by complex systems. By ramification, data combination is a chief requirement for digital knowledge. Understanding management should focus on integration instead of concentrating on integrating different understanding parts and producing new abilities.
The increasing ease of access to data is an obstacle in procedure management since assessing the datasets and decreasing. Based on the context of information assembly, engineers can develop a system to share valuable data with each stakeholder. The adoption of service procedure management (BPM) to develop activities enhances service standards and information management. The maintenance, repair, and operations (MRO) procedure is difficult under the BPM within this design. The MRO activities differ and depend on parts’ requirements, and the task schedule is not predictable (Vieira & Loures 2016).
MRO treatments’ significance in the aviation business’s sustainability improves productivity and reliability to offer quality service shipment. Business offering MRO services attempt to minimise maintenance costs and turn-around duration to take full advantage of earnings. Adopting the item lifecycle management (PLM) might decrease maintenance cost and shipment time, but PLM’s combination using MRO treatments does not constantly happen. Among the probable reasons, is the scarcity of PLM architecture designed and used to support product maintenance or service operations (Vieira & Loures 2016). MRO in aviation requires processing and item knowledge to make the most of time and improve service quality. Such details are limited and may be problematic for consumers to collect pertinent information for decision-making.
It is important to get real-time assessment and details concerning the device parts and engines based on reliable service delivery, evaluating its localisation and processing status. WIP keeping track of guarantees methodical access to procedure data and permit stakeholders to manage the job sequence and treatment tasks. Likewise, it is crucial to have accurate product data within each unit by directly reporting details with accuracy and punctuality to permit fast treatment execution. Therefore, real-time access to WIP understanding is a growing need for BD applications. The MRO procedure’s understanding is related to services and product details understanding, given that it clarifies the functions and procedures of MRO stages.
According to Vieira and Loures (2016), the benchmark information is critical in evaluating MRO performance since it recognises historical stats and WIP details. Numerous research study studies have revealed RFID innovation’s ability in components and resources monitoring, logistics management, WIP monitoring, and tool management in airline company operations (Deng et al., 2019; Vieira & Loures 2016; Tokgöz 2018).
Deng, Santos, and Curran (2019) suggested an internet-based product support system to handle MRO based upon support, use, and efficiency. The author argued that the method would combine the item development procedure with maintenance and support procedures. The authors classified the understanding included in MRO services into various stages based on a hierarchical style for its utilisation. The influence of item life management on MRO treatments works. Knowledge management has been challenging in academic research and methodological viewpoint.
Digitalisation is a significant source of competitive edge. Information analytics can be used to forecast client behaviour, procedures, or handling risk. It provides comprehensive and predictive insights to enhance organisational operations. Since the airline market is extremely cost-sensitive, rivalry in the business is fierce. Data management enhances critical competitive benefits for investors (Zaharia & Pietreanu 2018).
These market agents include passengers, investors, equipment suppliers, stakeholders, and manufacturers. The definition is suitable for considering the objectives of this research. Data transmission and processing supports digital approaches that provide direction, create initiatives, assess performance, assess growth, and redirect observational goals. The authors suggested that airline operators could implement data applications to improve client-based design or digitised architecture. The client-based model allows operators to change their services to suit new customer requirements and create curated experiences that enhance consumer loyalty. Big data architecture creates new price regimes for clients using different innovative designs (Ross, Beath & Sebastian, 2017).
Upadhya (2016) emphasised that electronic communication programs have been harmonised with functional ‘beacons’ accessible from the airport, which enable location identification by customers. Therefore, ‘beacons’ enhances customer experience during preflight and post-flight services. The technology provides airport directions to avoid flight delays (Upadhya 2016). The beacon-based location program reduces the time-wasting procedures at security checkpoints (Straker & Wrigley 2016).
Some airports, such as Dubai International, have embraced facial recognition technology that minimises clients’ need to present boarding passes after the check-in session. Tower controllers deploy BD systems to control runway and lighting equipment, which is crucial in maintaining aircraft balance and identifying security threats. Maintaining a safe and secure aircraft movement is the basis of efficient and stable operations to guarantee client safety, security identification, curated travel experience, and sustainable business operations. Using these apparatus lowers the expenses of predictive maintenance and mitigates the vulnerability to internal and external threats.
Big Data and Communication Management
Martin-Domingo & Martín (2016) highlighted that an efficient information release requires identifying vital elements that affect consumer curated travel experience. Guests complain of shortages of flight program data, which makes their journeys agonising and inconvenient. BD technology adoption to reduce these hassles enhances the traveller’s curated travel experience traveller and supports a particular airport’s choice. Clients prefer routine updates about flight delays because of their aggravations with queues with unknown waiting periods. The self-important nature of gatekeeper, security demands and practices stimulates travellers’ disappointment (Padrón et al. 2016).
Data applications such as line metering devices supply notifications to guests on shorter lines and estimated waiting duration. Digital technology supports the release of security stations in airports, where clients walk through using their bags without intrusive searches (Schwartz 2014). Many tourists invest more time shopping and other commercial activities at airports, and a number of these consumers complain of data network failures. The lengthy waiting time in creating orders is a challenge in many airports. Nevertheless, the adoption of innovated technologies allows clients to pre-order products from the terminal to enhance the travel experience (Kalakou, Psaraki-Kalouptsidi & Moura 2015).
Company lounges in particular airports have taken advantage of these inventions, where a company tourist’s tea service is provided on arrival from the landing lounge. Schwartz (2014) asserts that airport navigation is another challenge experienced by customers. For the most part, after long travel flights, passengers who could be exhausted find it challenging to find their locations. Because of this obstacle, they are sometimes overdue to boarding, triggering flight cancellation or rescheduled departures.
The concern is challenging because fight cancellation involves offloading their luggage, which develops hold-ups at hub airports that rely on the connection. To prevent such humiliations, airline company operators might implement mobile programs with GPS enhancements to assist passengers and reduce such defects. GPS motors with wireless sensors play an important role in easing the uniqueness of limited access to services.
Digital data and innovations support such category. The execution of data partnerships involving airlines represents a more substantial quantity of travellers use airports. Digital apps allow the timely interventions of queries and complaints and the adoption of proactive approaches to address passenger inconvenience (Wong & Wong 2017). Among the main areas appreciated by passengers in airports would be service quality. By implication, the passenger’s capability to socialise with employees is valuable since it enriches operations’ timeliness and reduces uncertainty. Such interaction permits passengers to receive advice or aid in a timely fashion. Additionally, it shapes the airport’s positive customer perceptions, thereby recommending acquaintances and relatives, which is critical in fostering a favourable brand image and better business prospects (Jasimuddin, Mishra & Saif Almuraqab 2017).
Digital strategy and technology in airports have improved the marketing of airport infrastructure. Based on its effectiveness, airline operators and managers can categorise passengers according to their features, needs, and travel routine. This feat can only be possible using the information generated from its operations. Digital technologies and data applications support such classification. The appropriate categorisation and communication of passenger prerequisites facilitate the expeditious travel processes for more straightforward transit (Jasimuddin, Mishra & Saif Almuraqab 2017). Despite diverse challenges, air transportation has soared in global markets.
An efficient digital implementation boosts productivity. Crespo-Almendros and Del Barrio-García (2016) argued that BD architecture should be included in various client requirements and save operating costs. The authors stressed that many airline company operators had introduced web channels for ticketing to avoid travel agencies’ challenges. The authors thought that online ticket solutions are cheaper and more efficient than workplace management procedures (Crespo-Almendros and Del Barrio-García, 2016). A survey on Online ting examined the level to which operators might integrate its marketing procedures with online platforms. Their study was based on details stemmed from monetary and nonmonetary online data. The research study findings suggest that distinct online services must be used for different users according to their understanding of internet use. Based on this assumption, the IoT architecture adds to consumer relations’ general performance in the air travel sector.
Conclusions
This thesis explores the trends, status of big data in airlines and its implications in aviation investment. The paper highlights areas of interest based on these submissions, which include technologies, used cases, applications, products, suppliers, research and development.
Successful and reliable monitoring and controlling of flight data requires accurate identification, characterisation, storage, and retrieval of the needed information based on availability, efficiency, quality, and price considerations. Fulfilling flight standards’ needs and requirements will be challenging because some unstructured messages cannot be merged while structure or conflict of interest is observed. Consequently, most flight data are not stored or retrievable. Effective monitoring is expensive and could be burdened by access and usage restraints because of privacy problems. Data monitoring and controlling are challenging because flight data is sometimes not regularly available with quality, particularly information correctness and suitability.
While information retrieval equipment purchase is a general-purpose concern for flight investors, the cost of advanced architecture may hinder quality systems. While some space procedures give telemetry information, most do not. While they might be surveyed by the Department of Protection (DoD) radar systems, those details are not presently offered to the FAA for air traffic control objectives. Specifications and information streams require functional information reporting and storage. Therefore, big data would complement existing communication infrastructure by providing advanced capability via data mining initiatives. In actual time, the methods for information collection include wireless links from aeroplane cabins to satellite-based systems or ground networks. Effective monitoring systems utilise aircraft communications structures.
Data analyses allow the cabin crew and pilot to reinforce their decision-making procedure with accurate information and business intelligence. For example, decoded data generated during flight form the basis for real-time decisions and flight patterns. The computational procedures to optimise flight routes enhance cost minimisation on fuel, reduce air transportation, prevent bad weather flights, and access safer destinations. Airlines create enormous quantities of data during online communication, online cost comparison and ticket buying, online check and seat preference, personalisation of supplies, and beverage selection.
Most airline businesses use sophisticated algorithms to accumulate and analyse the huge amounts of information made by flight detectors and data sensors attached to each aircraft. These sensors and recording device detectors track flight movements, forecast maintenance, identify errors decrease gas consumption, and re-evaluate turn-around-time at airports.
Data evaluation as a form of business intelligence has been a formative decision in aviation. The competitive edge of most airlines relies on data analytics. The capacity to analyse quantum data into specific points creates the difference in price regime, promotion packages, customer requirements, travel needs, and quality. An investor gains knowledge by applying informed decisions on flight operations and management. As a result, the management enhances the passenger’s travel experience using data analytics. By implication, investors such as Emirates airlines utilise business intelligence gathered across many touch-points to execute behavioural promotions, product differentiation, customised services and solutions.
As part of the used cases for flight data applications and evaluation data, recorders ad programs help airlines identify security trends and present information for safety performance indexes. The aggregate review enables operators to verify flight information beyond their restricted databases. Investors or airline management could use benchmarking tools to evaluate the safety performance for international flight routes and security patterns. These applications permit pilots to compare and escalate the decision-making process for unbiased and valid functionality. Data assembly allows collaborative frameworks and partnership among airlines as it offers comprehensive diagnoses when required.
Data transfer, generation, evaluation and transmission relays communication networks for optimum performance. The choice of network communication equipment depends on the aircraft, manufacturer, or technology. However, communication links must be reliable and efficient to overcome notable communication issues in aviation. As approved by aviation regulators, these networks standards mitigate system collapse or communication disruption during high altitudes, bad weather conditions, and geographic locations. Communication over any network nodes or logical links should have discretion.
The content of the communication is substantial, not the communication itself. Thus, network confidentiality requires that data links be reliable, confidential, and secured. To improve resource functionality without breaching regulations and policies arrangements, airline companies must collect and evaluate huge data to coordinate their resources. As a result, big data facilitates the accumulation and analysis of data in aviation. The vast methods and frameworks of big data analysis encourage technology integration in aviation markets.
Big data analytics can be infused in-demand systems or platforms to manage procurement schedules, detect fault delivery systems, forecast logistics routes and aircraft requirement. Workflow management systems that sustain supply and product chain distribution of air travel programs need demand data inputs. In particular, Flight Watch Website gathers evaluate and displays information about booking rates, weather outlooks, workers rotations, and flight terminal limits to mention a few. As airlines often possess or generate a vast network of hubs to assist ground control centres in monitoring, reporting and observing operational details.
Thus, airports and airline companies must collaborate to minimise equipment turn-around times, reduce traveller disruptions, delays and enhance supply utilisation. Airline management and system maintenance comprise many trouble locations that require effective scheduling, monitoring and repairs. Arranging the maintenance lists is challenging because each unit includes planning, controlling, and implementing varied components, resources, suppliers and vendor.
Therefore, repair scheduling must acknowledge the multifaceted distribution of events to enhance performance. Aircraft MROs must accommodate large project networks comprising several activities, multiple components and different maintenance levels, assimilation of products, resources, procedures scheduling, and project preparation. Consequently, the system must deploy several frameworks, configurations to detect fault lines. The configurations are either fitted internally on the aircraft or gathered from distant sensors or servers wired to collect data information and trouble spots.
The aviation sector is diverse with many businesses in commercial, defence, military projects and applications. Despite the project and application, the aerospace sector items are identified as sophisticated products because of the continuous technology progression and capital funding. Investor’s complex needs are growing with a new dimension under safety regulations. These physiognomies make the aerospace industry exciting for innovative ideas, similar to other manufacturing fields such as the nuclear energy market.
The aerospace sector possesses a record of generating advancements. With its history in innovations, the aviation sectors have triggered the mission of ‘higher, quicker, and farther’, product technologies aimed at improving performance. The market launched several new advancements, such as commercial trips, fast flight, and space travel, and are relentless to define its attributes. Most of these technologies were components of complex systems, including landing gears, communication cabin, traffic control service, or GPS navigation. These innovations were the outcome of cumulative growth initiatives that combined technologies coming to create complex devices. Consequently, innovative ideas dedicated to satisfy passengers and enhance performance are evolving.
Gaps and Recommendations for Big Data in Aircraft
Aviation research has been limited to performance, service quality, and profit maximisation. This research study confirms the gap in the literature concerning big data in aviation. Comitz and Kersch (2016) asserted that the adoption of data strategic applications and digitalisation technology had exposed the classification of service gaps in air travel. Digitalisation innovation guarantees that the integration of disparate services.
The authors emphasised that integrating distinct providers and connecting them with clients has been critical in implementing and enhancing airline performance. For example, digital communications have significantly improved advertising campaigns and generated invaluable client information that improved service delivery. Such information influences the effective deployment of products and services to meet specific demands. Digitalisation has been instrumental in preventing duplication of solutions, particularly gate holing departments, luggage selection, and retail places (Comitz & Kersch 2016). It ensures that airline operators and airports are managed under global service delivery standards to boost curated travel experience.
Knowledge gaps affect the implementation of BD technology in the aviation market. Jeeradist, Thawesaengskulthai, and Sangsuwan (2016) argued that differences in financial strength and profit maximisation affect the adoption of big data applications among airline investors. Like Africa, airlines in certain areas experience shortfalls in earnings that curtail the deployment of resources in data applications. However, Arab North American airline investors have made substantial investments in BD platforms to boost service delivery and profitability. Managers who cannot embrace digital applications rely on foreign migrants at high prices, creating understanding gaps in operations.
As a result, airline company operators should set up various procedures to meet customers’ demands. Managers examine trends in innovation demands based on geographical differentiation and develop particular applications based on the research study outcome. There are drawbacks to data applications, most notably the reduction of private passengers. The reduction of such social relations can result in the entrenchment of adverse perceptions about airport operations, particularly customers with special needs. As a result, airline operators must install advanced navigation tools and devices to meet customers’ demands and improve safety.
Like the visually impaired, tourists with special requirements cannot operate most electronic kiosks because they utilise touchscreen technologies (Straker & Wrigley 2016). Passenger perception and attitude is another challenge to a knowledge gap. Some passengers may not accept customised programs in an airport due to customer information misuse, which makes digital implementation undesirable to a segment of their customer base. Some airports sped funds to create awareness of the need to adopt data innovations to increase their services’ increased value and effectiveness.
Investors need to survey different technologies based on their preferences, safety, and flaws to address their customers’ concerns. For example, managers examine technology demands based on geographical differentiation and create specific applications based on the study outcome. Digital programs should be user-friendly and interactive to optimise customer perceptions and value (Straker & Wrigley, 2016). Phillips (2016) concluded that most airports lack sufficient staff for specific needs.
For example, the aged passengers may not understand digital applications and require staff support to locate boarding sections, carry their luggage, and shop items. Based on this challenge, airline operators and managers should employ more support personnel to create awareness of digital applications and assist those who cannot use smart devices. This strategy would reduce inconvenience and improve passenger curated travel experience.
Consequently, support staff should engage in self-assurance attempts for travellers that resist digital communication technology. Digitised kiosks should be armed with example sound straps to boost the wide variety of alternatives for the disabled and impaired, in obtaining the essential services. Straker and Wrigley (2016) cited building design as another impediment to adopting data applications in airports. Many airports have thick glass multipurpose layouts, creating difficulties for network connectivity, and locating customers. Such airport architecture makes it challenging the managers and staff to secure safe transit of passengers from the terminal building into their destination.
As a result, investors must consider the architectural layout of terminals to match the deployment of electronic communication devices and digital applications (Straker & Wrigley 2016). Most travellers in developing nations have a higher affinity for manual security checks rather than utilising digital technologies. Chen, Batchuluun, and Batnasan (2015) listed demographic issues as an impediment and a significant literature gap. These passengers understand the process of manual check-in, based on its use in their localities.
Cultural drift and expectations hamper the adoption of digital applications in aviation. Such obstacles introduce debatable variations in adopting digital innovations and applications.
Some airports install digital applications, while others contract out the connection due to the expense ramification. As a result, it is hard for travellers to gain access to real-time information and services. Chen, Batchuluun, and Batnasan (2015) kept in mind that outsourcing digital implementations often hinders efficiency from improving the traveller experience. Such restrictions develop distorted operations and imbalanced guest experience as they travel through various airports and nations (Chen, Batchuluun & Batnasan 2015). Digitalisation offers a range of efficiencies and possibilities, in operations and management. Aviation investment is competitive, with operators competing for clients and profits. Thus, the cost implication of digitalisation may discourage investors who comprehend its benefits.
The airline market is experiencing its most substantial modification, given its deregulation. Digital improvement and monetary developments are forcing significant worker efficiency and service quality (Crespo-Almendros & Del Barrio-García, 2016). Considering that data applications’ adaptation might increase the value and income, digitalisation applies in the air travel sector. The air travel market sustains its development in cost configuration, security architecture, and brand distinction. Regardless of the strength of competition emanating from lower travel prices and the restrictions in maintenance, these service airlines’ expense setup is a substantial reason in embracing BD designs and digitalisation equipment.
A critical characteristic of oligopolies in air travel is the need for substantial capital investments to increase efficiency. The assumption creates high repaired costs, stimulating considerations for expense reductions in operations using digital options (Crespo-Almendros & Del Barrio-García 2016). Additionally, dealing with large numbers to achieve scalable advantages and cost decreases could substantially enhance sustainability (Crespo-Almendros & Del Barrio-García 2016). Based on this assumption, strategic management procedures can be established to lower operating costs and improve profitability. Other innovations include 3D-printing, huge data, and the Internet of Things (IoT).
The security nature of airline maintenance makes the sector vulnerable to data frameworks. Digitalisation and globalisation have reduced the gap between airlines and external developers. However, the shrink in area and environment raises a new predicament for threats and attacks. Relating to synergistic security dangers, the expense of security is expensive. Interdependent security threats are a risk induced by specific actions and operations (Comitz & Kersch 2016). A synergistic security threat could be a damaging object or a terrorist interference positioned within an aeroplane. Chen et al. (2016) studied aspects that influenced the interconnected solution.
The authors embraced a method of investing substantial funds into issues affected by private actions. The authors utilised the Nash equilibrium model to examine synergistic options. Under this design, air travel operators must invest in security to maximise profits. These techniques assist investors in executing digital innovation and lower operational expenses. Strategic information management in security architecture consists of automated control cabin, Blockchain innovation, and enhanced truth. Brand name distinction supports digitalisation in air travel. Because digitalisation decreases the expense of financial investment, most financiers understand the requirement for implementation. Hence, airline financiers are executing strategic information applications that are hard to mimic. The capacity to incorporate big information, Blockchain innovation, AR, IoT and 3D printing differentiates airline companies with performance.
Air transportation has become a growing industry in terms of cost and profit. Therefore, the need for quality service delivery cannot be overemphasised. Airline operators must encourage satisfaction by improving the travellers’ flight experience. While airline operators have separated services based on the traveller’s financial capability, it is vital to have a standardised service delivery benchmark. Although service separation enhances the firm’s competitive advantage, consumer experience builds the probability of service sustainability (Huang, Lee, & Chen, 2017). Consumer satisfaction is one of the best research variables for airlines in the competitive market, and the flight experience is an indicator of for analysis.
The development of an effective quality service system is an indicator of business growth. Service quality is the buyer’s impression of the proficiency of the airline and its services. Most airlines experience a business culture’s ill effects where the operating cost is prioritised other than enforcing quality service delivery that drives the consumer experience. How airline operators promote air travel services creates issues because the promotions neglect to show a practical perspective of in-flight performance. This challenge creates a disparity between travellers’ assumptions about airline quality and their experience. Service quality is a focus for airline organisations in competing and engraving their picture in travellers’ psyches.
Many airlines have enforced service quality through package personalisation, which incorporates ground and in-flight activities, particularly from maintaining travellers and gaining new ones. Other than those practices, in-flight dinners and food service is another significant part of the airline task. The in-flight nourishment services are viewed as a major aspect of the marketing strategy for business or tourist explorers. Traveller’s satisfaction is one of the best resources for airline organisations in the present competitive market, and the long flight experience is something extraordinary for the client.
Recommendations
Aircraft security provides an appealing opportunity to use AI against attacks. With AI, displays boards are not required, unlike what human intelligence needs. The advantage of AI algorithms is that substantially BD sets can be utilised and analysed in little time. AI is beneficial is in identifying functions and attributes of possible terrorists, behavioural and changes and activity. Since considerable information is gathered from flight manifest, such data are processed to determine abnormalities that alert security officers for extra human attention and examination. AI improves behavioural detection systems to produce a unique analysis of all travellers.
The system is efficient in risk-based security techniques and vetting, thereby accelerating screening lanes into numerous alternatives. Synthetic intelligence algorithms can effectively evaluate unstructured information to make traveller threat evaluations more effective, efficient and precise. The prevalent usage of video cameras and tracking gadgets offers real-time monitoring. One obstacle in establishing efficient structures for AI is human capital. There is a severe lack of AI scientists and designers for such aviation abilities, producing an extremely competitive environment. Aircraft security requirements might require to be filled with business items rather than developing personalised AI systems. As a result, aircraft relations must enact laws to enforce the adoption of BD technology in all areas of the industry.
Leveraging on BD technology would enhance smarter maintenance, safer flights and service quality. For example, United Airlines leveraged big data technology by encouraging customer loyalty. The management analyses customer data to set promotion packages for new and returning customers. The move draws new customers and encourages returning customers to advertise the airline service. British Airways deployed AI services called the KYC feature to attract passenger feedback.
The results are reviewed and used for administrative purposes. EasyJet utilises BD analysis to determine pricing regimes, which give the organization a competitive edge over its competitors. The technology provides on-demand pricing formula that regulates travel cost based on passenger number, route schedules and travel periods. Southwest airline applies inflight AI to evaluate flight reports, ATC, communication networks and climate status. Thus, BD technology would reduce operation costs, enhance service delivery and passenger’s travel experience.
References
Abdelghany, A., Abdelghany, K., & Azadian, F. (2017). Airline flight schedule planning under competition. Computers and Operations Research, 87, 20–39. Web.
Abreu, C. G. S., De Souza, A. L. L., & Câmara, M. K. (2017). Operational efficiency and market concentration: a comparative analysis of Brazilian air passenger transport companies. Sistemas & Gestão, 12(3), 308–315. Web.
Adacher, L., Flamini, M., & Romano, E. (2017). Sectors co-operation in air traffic management. IFAC-PapersOnLine, 50(1), 4222–4227. Web.
Aktürk, M. S., Atamtürk, A., & Gürel, S. (2014). Aircraft rescheduling with cruise speed control. Operations Research, 62(4), 829–845. Web.
Al-Jader, R., & Sentosa, I. (2015). Structural equation modeling of e-service recovery satisfaction and customer retention in the airline industry in Malaysia. International Journal of Marketing Studies, 7(4), 105-120. Web.
Alt, R., & Zimmermann, H. (2017). Electronic markets on big data services. Electronic Markets, 27(3), 191-195. Web.
Amare, T., Helvik, B. E., & Heegaard, P. E. (2018). A modeling approach for dependability analysis of smart distribution grids. 21st Conference on Innovation in Clouds, Internet and Networks, ICIN 2018, 1–8. Web.
Anderson, A. B. A., Kumar, A. J. S., & Christopher, A. B. A. (2019). Analysis of flight delays in aviation system using different classification algorithms and feature selection methods. Aeronautical Journal, 123(1267), 1415–1436. Web.
Arikan, U., Gürel, S., & Aktürk, M. S. (2017). Flight network-based approach for integrated airline recovery with cruise speed control. Transportation Science, 51(4), 1259–1287. Web.
Armatli Köroğlu, B., & Özelçi Eceral, T. (2017). Rethinking space in production networks: Network centrality and R&D activities in Ankara defense and aviation cluster. Gazi University Journal of Science, 30(2), 17–33.
Arunachalam, D., Kumar, N., & Kawalek, J. P. (2018). Understanding big data analytics capabilities in supply chain management: Unravelling the issues, challenges and implications for practice. Transportation Research Part E: Logistics and Transportation Review, 114(2), 416–436. Web.
Atkinson, S. E., Ramdas, K., & Williams, J. W. (2016). Robust scheduling practices in the U.S. airline industry: Costs, returns, and inefficiencies. Management Science, 62(11), 3372–3391. Web.
Badea, V. E., Zamfiroiu, A., & Boncea, R. (2018). Big data in the aerospace industry. Informatica Economica, 22(1/2018), 17–24. Web.
Badiezadeh, T., Saen, R. F., & Samavati, T. (2018). Assessing sustainability of supply chains by double frontier network DEA: A big data approach. Computers and Operations Research, 98(1), 284–290. Web.
Ben Ahmed, M., Ghroubi, W., Haouari, M., & Sherali, H. D. (2017). A hybrid optimization-simulation approach for robust weekly aircraft routing and retiming. Transportation Research Part C: Emerging Technologies, 84, 1–20. Web.
Benlic, U. (2018). Heuristic search for allocation of slots at network level. Transportation Research Part C: Emerging Technologies, 86, 488–509. Web.
Bertsimas, D., & Gupta, S. (2016). Fairness and collaboration in network air traffic flow management: An optimization approach. Transportation Science, 50(1), 57–76. Web.
Bertsimas, D., Gupta, S., & Lulli, G. (2014). Dynamic resource allocation: A flexible and tractable modeling framework. European Journal of Operational Research, 236(1), 14–26. Web.
Bhanap, S., & Kawthekar, S. (2015). Data mining for business intelligence in social network: A survey. International Advanced Research Journal in Science, Engineering and Technology, 2(12), 129-131. Web.
Blasch, E., Kostek, P., Pačes, P., & Kramer, K. (2015). Summary of avionics technologies. IEEE Aerospace and Electronic Systems Magazine, 30(9), 6–11. Web.
Bogicevic, V., Yang, W., Bujisic, M., & Bilgihan, A. (2017). Visual data mining: Analysis of airline service quality attributes. Journal of Quality Assurance in Hospitality and Tourism, 18(4), 509–530. Web.
Bumblauskas, D., Nold, H., Bumblauskas, P., & Igou, A. (2017). Big data analytics: Transforming data to action. Business Process Management Journal, 23(3), 703–720. Web.
Cao, X., Yang, P., Alzenad, M., Xi, X., Wu, D., & Yanikomeroglu, H. (2018). Airborne communication networks: A survey. IEEE Journal on Selected Areas in Communications, 36(9), 1907–1926. Web.
Chang, J. Y., Jeng, J. T., Sheu, Y. H., Jian, Z. J., & Chang, W. Y. (2020). An efficient data collection path planning scheme for wireless sensor networks with mobile sinks. Eurasip Journal on Wireless Communications and Networking, 1–23. Web.
Chen, J., & Piani, N. (2017). Research on business intelligence with data mining applications. International Journal of Business and Economics Research, 6(2), 19-24. Web.
Chen, J., Batchuluun, A., & Batnasan, J. (2015). Services innovation impact to customer satisfaction and customer value enhancement in airport. Technology in Society, 43(1), 219-230. Web.
Chen, R., Zhang, D., & Li, B. (2020). Spatial–temporal calculation simulation of ecological footprint of resource and environmental pollution in green communication. Eurasip Journal on Wireless Communications and Networking. Web.
Chen, W., Huang, Y., Yang, H., Li, J., & Lu, X. (2021). A passenger risk assessment method based on 5G-IoT. Eurasip Journal on Wireless Communications and Networking, 1–20. Web.
Choi, T. M., Wallace, S. W., & Wang, Y. (2018). Big data analytics in operations management. Production and Operations Management, 27(10), 1868–1883. Web.
Cimmino, N., Ponnusamy, S. S., Garriga, A. G., & Mainini, L. (2020). A modelling and simulation framework for the integrated design of aircraft systems. Proceedings of the Institution of Mechanical Engineers, Part G: Journal of Aerospace Engineering, 234(10), 1648–1660. Web.
Comitz, P., & Kersch, A. (2016). Aviation analytics and the Internet of Things. Integrated Communications Navigation and Surveillance, 1(1), 1-7. Web.
Crespo-Almendros, E., & Del Barrio-García, S. (2016). Online airline ticket purchasing: Influence of online sales promotion type and Internet experience. Journal of Air Transport Management, 53(1), 23–34. Web.
Czerny, A. I., Cowan, S., & Zhang, A. (2017). How to mix per-flight and per-passenger based airport charges: The oligopoly case. Transportation Research Part B: Methodological, 104, 483–500. Web.
Dalmau, R., & Prats, X. (2017). Controlled time of arrival windows for already initiated energy-neutral continuous descent operations. Transportation Research Part C: Emerging Technologies, 85(5), 334–347. Web.
Darma, J. (2016). The effect of quality of business intelligence system on quality of decision making (survey on financial institutions in Medan city: North Sumatera. European Journal of Accounting, Auditing and Finance Research, 4(5), 49-56. Web.
De Bruecker, P., Beliën, J., Van den Bergh, J., & Demeulemeester, E. (2018). A three-stage mixed integer programming approach for optimizing the skill mix and training schedules for aircraft maintenance. European Journal of Operational Research, 267(2), 439–452. Web.
Deng, Q., Santos, B., & Curran, R. (2019). A practical dynamic programming based methodology for aircraft maintenance check scheduling optimization. European Journal of Operational Research, 281(2), 256-273. Web.
Dou, X. (2020). Big data and smart aviation information management system. Cogent Business and Management, 7(1). Web.
Dubey, R., Gunasekaran, A., Childe, S. J., Papadopoulos, T., Luo, Z., Wamba, S. F., & Roubaud, D. (2019). Can big data and predictive analytics improve social and environmental sustainability? Technological Forecasting and Social Change, 144(5), 534–545. Web.
Dubey, R., Luo, Z., Gunasekaran, A., Akter, S., Hazen, B. T., & Douglas, M. A. (2018). Big data and predictive analytics in humanitarian supply chains: Enabling visibility and coordination in the presence of swift trust. International Journal of Logistics Management, 29(2), 485–512. Web.
Ebarefimia, S. (2017). Impact of Blockchain technology in enhancing customer loyalty programs in airline business. International Journal of Innovative Research and Advanced Studies, 4(6), 257-263.
Eckstein, D., Goellner, M., Blome, C., & Henke, M. (2015). The performance impact of supply chain agility and supply chain adaptability: The moderating effect of product complexity. International Journal of Production Research, 53(10), 3028–3046. Web.
Fas-Millán, M. A., & Pastor, E. (2019). Controller-pilot data link communications display oriented to multiple remotely piloted aircraft pilots. International Review of Aerospace Engineering, 12(1), 1–11. Web.
Fasone, V., Kofler, L., & Scuderi, R. (2016). Business performance of airports: Non-aviation revenues and their determinants. Journal of Air Transport Management, 53, 35–45. Web.
Fourati-Jamoussi, F., & Niamba, C. (2016). An evaluation of business intelligence tools: A cluster analysis of users’ perceptions. Journal of Intelligence Studies in Business, 6(1), 37-47. Web.
Gao, S., Dai, X., Hang, Y., Guo, Y., & Ji, Q. (2018a). Airborne wireless sensor networks for airplane monitoring system. Wireless Communications and Mobile Computing, 2018(32), 1-18. Web.
Gauzelin, S., & Bentz, H. (2017). An examination of the impact of business intelligence systems on organizational decision making and performance: The case of France. Journal of Intelligence Studies in Business, 7(2), 40-50. Web.
Ge, X., & Han, Q. L. (2017). Distributed formation control of networked multi-agent systems using a dynamic event-triggered communication mechanism. IEEE Transactions on Industrial Electronics, 64(10), 8118–8127. Web.
Ghalehkhondabi, I., Ahmadi, E., & Maihami, R. (2020). An overview of big data analytics application in supply chain management published in 2010-2019. Production, 30(1), 1–22. Web.
Govindan, K., Cheng, T. C. E., Mishra, N., & Shukla, N. (2018). Big data analytics and application for logistics and supply chain management. Transportation Research Part E: Logistics and Transportation Review, 114(6), 343–349. Web.
Grekhov, A., Kondratiuk, V., & Ilnytska, S. (2020). RPAS communication channels based on WCDMA 3GPP standard. Aviation, 24(1), 42–49. Web.
Gupta, S., Kar, A. K., Baabdullah, A., & Al-Khowaiter, W. A. A. (2018). Big data with cognitive computing: A review for the future. International Journal of Information Management, 42(6), 78–89. Web.
Gurtov, A., Polishchuk, T., & Wernberg, M. (2018). Controller–pilot data link communication security. Sensors, 18(5), 1–12. Web.
Haferkorn, P. (2018). Risk communication from an audit team to its client. In Springer Briefs in Applied Sciences and Technology. Web.
Haghighat, N. (2017). Airline service quality evaluation: A review on concepts and models. Economics, Management and Sustainability, 2(2), 31–47. Web.
Hapsari, R., Clemes, M. D., & Dean, D. (2017). The impact of service quality, customer engagement and selected marketing constructs on airline passenger loyalty. International Journal of Quality and Service Sciences, 9(1), 21–40. Web.
Hassani, H., Huang, X., & Silva, A. E. (2019). Big data and climate change. Big Data and Cognitive Computing, 3(1), 1–17. Web.
Hofmann, E. (2017). Big data and supply chain decisions: The impact of volume, variety and velocity properties on the bullwhip effect. International Journal of Production Research, 55(17), 5108–5126. Web.
Ho-Huu, V., Hartjes, S., Visser, H. G., & Curran, R. (2018). Integrated design and allocation of optimal aircraft departure routes. Transportation Research Part D: Transport and Environment, 63(8), 689–705. Web.
Hong, J. W., & Park, S. B. (2019). The identification of marketing performance using text mining of airline review data. Mobile Information Systems, 2019(1), 1–8. Web.
Hrastovec, M., & Solina, F. (2016). Prediction of aircraft performances based on data collected by air traffic control centers. Transportation Research Part C: Emerging Technologies, 73, 167–182. Web.
Huang, P.L., Lee, B. C. Y., & Chen, C.C. (2017). The influence of service quality on customer satisfaction and loyalty in B2B technology service industry. Total Quality Management & Business Excellence, 1–17. Web.
Jasimuddin, S., Mishra, N., & Saif Almuraqab, N. (2017). Modelling the factors that influence the acceptance of digital technologies in e-government services in the UAE: A PLS-SEM approach. Production Planning & Control, 28(16), 1307-1317. Web.
Jeeradist, T., Thawesaengskulthai, N., & Sangsuwan, T. (2016). Using TRIZ to enhance passengers’ perceptions of an airline’s image through service quality and safety. Journal of Air Transport Management, 53(3), 131-139. Web.
Jin, D. H., & Kim, H. J. (2018). Integrated understanding of big data, big data analysis, and business intelligence: A case study of logistics. Sustainability, 10(10), 1-15. Web.
Joudeh, J. M. M., & Dandis, A. O. (2018). Service quality, customer satisfaction and loyalty in internet service providers. International Journal of Business and Management, 13(8), 108. Web.
Kalakou, S., Psaraki-Kalouptsidi, V., & Moura, F. (2015). Future airport terminals: New technologies promise capacity gains. Journal of Air Transport Management, 42(1), 203-212. Web.
Kasim, K., & Mahmut, B. (2020). Evaluation of airlines performance using an integrated critic and codas methodology: The case of Star Alliance Member Airlines. Studies in Business and Economics, 15(1), 83–99. Web.
Keller, J., Mendonca, F., Cutter, J., Suckow, M., & Dillman, B. (2020). Justification and development of competencies to transform a collegiate aviation flight program. The Journal of Competency-Based Education, 5(3), 1–13. Web.
Khanh, G. (2017). Customer satisfaction of Vietnam Airline domestic service quality. International Journal of Quality Innovation, 3(1). Web.
Khuong, M., & Uyen, L. (2014). The factors affecting Vietnam airlines service quality and passenger satisfaction: A mediation analysis of service quality. International Journal of Innovation, Management and Technology, 5(5), 327-333. Web.
Khuwaja, A. A., Chen, Y., Zhao, N., Alouini, M. S., & Dobbins, P. (2018). A survey of channel modeling for uav communications. IEEE Communications Surveys and Tutorials, 20(4), 2804–2821. Web.
Kim, H. (2018). What constitutes professional communication in aviation: Is language proficiency enough for testing purposes? Language Testing, 35(3), 403–426. Web.
Kim, Y. (2016). On the optimal configuration of framed slotted ALOHA. Journal on Wireless Communications and Networking, 1(1), 12-19. Web.
Korvesis, P., Besseau, S., & Vazirgiannis, M. (2018). Predictive maintenance in aviation: Failure prediction from post-flight reports. Proceedings – IEEE 34th International Conference on Data Engineering, ICDE 2018, 1423–1434. Web.
Kumar, S., & Zymbler, M. (2019). A machine learning approach to analyze customer satisfaction from airline tweets. Journal of Big Data, 6(1), 1–16. Web.
Kurnia, P., & Suharjito, S. (2018). Business intelligence model to analyze social media information. Procedia Computer Science, 135, 5-14. Web.
Lališ, A., Socha, V., Křemen, P., Vittek, P., Socha, L., & Kraus, J. (2018). Generating synthetic aviation safety data to resample or establish new datasets. Safety Science, 106(2), 154–161. Web.
Lamba, K., & Singh, S. P. (2018). Modeling big data enablers for operations and supply chain management. International Journal of Logistics Management, 29(2), 629–658. Web.
Lee, I. (2017). Big data: Dimensions, evolution, impacts, and challenges. Business Horizons, 60(3), 293–303. Web.
Lee, Y., & Park, J. (2016). Impact of a sustainable brand on improving business performance of airport enterprises: The case of Incheon international airport. Journal of Air Transport Management, 53(1), 46-53. Web.
Lestiani, M. E., Yudoko, G., Yassierli, & Purboyo, H. (2017). Developing a conceptual model of organizational safety risk: Case studies of aircraft maintenance organizations in Indonesia. Transportation Research Procedia, 25, 136–148. Web.
Li, B., Ming, X., & Li, G. (2017). Big data analytics platform for flight safety monitoring. IEEE 2nd International Conference on Big Data Analysis, 2017, 350–353. Web.
Li, J., Xu, L., Tang, L., Wang, S., & Li, L. (2018). Big data in tourism research: A literature review. Tourism Management, 68(2018), 301–323. Web.
Li, W. T., Zhao, M., Wu, Y. H., Yu, J. J., Bao, L. Y., Yang, H., & Liu, D. (2021). Collaborative offloading for UAV-enabled time-sensitive MEC networks. Eurasip Journal on Wireless Communications and Networking, 2021(1), 1–17. Web.
Madni, A. M., & Sievers, M. (2018). Model-based systems engineering: Motivation, current status, and research opportunities. Systems Engineering, 21(3), 172–190. Web.
Maksood, F., & Achuthan, G. (2016). Analysis of data mining techniques and its applications. International Journal of Computer Applications, 140(3), 6-14. Web.
Marinus, B. G., & Poppe, J. (2015). Data and design models for military turbo-propeller aircraft. Aerospace Science and Technology, 41, 63–80. Web.
Martin-Domingo, L., & Martín, J. (2016). Airport mobile internet and innovation. Journal of Air Transport Management, 55(1), 102-112. Web.
Mebrahtu, A., & Srinivasulu, B. (2017). Web content mining techniques and tools. International Journal of Computer Science and Mobile Computing, 6(4), 49-55. Web.
Mehmood, R., & Graham, G. (2015). Big data logistics: A health-care transport capacity sharing model. Procedia Computer Science, 64(1), 1107–1114. Web.
Milambo, D., & Phiri, J. (2019). Aircraft spares supply chain management for the aviation industry in Zambia based on the supply chain operations reference (SCOR) model. Open Journal of Business and Management, 07(03), 1183–1195. Web.
Milkau, U., & Bott, J. (2015). Digitalisation in payments: From interoperability to centralized models? Journal of Payments Strategy & Systems, 9(3). 321-340.
Mitroff, S. R., & Sharpe, B. (2017). Using big data to solve real problems through academic and industry partnerships. Current Opinion in Behavioral Sciences, 18, 91–96. Web.
Mohib, A. A., Azad, R., Haque, S., & Hossain, M. F. (2017). Logistics and supply chain : An overview of business jet aircraft manufacturing. Journal of Economics and Sustainable Development, 8(10), 20–29.
Nazir, S., Ali, Y., Ullah, N., & García-Magariño, I. (2019). Internet of Things for healthcare using effects of mobile computing: A systematic literature review. Wireless Communications and Mobile Computing, 2019, 1-21. Web.
Nguyen, T., Zhou, L., Spiegler, V., Ieromonachou, P., & Lin, Y. (2018). Big data analytics in supply chain management: A state-of-the-art literature review. Computers and Operations Research, 98(9), 254–264. Web.
Ni, X., Wang, H., Che, C., Hong, J., & Sun, Z. (2019). Civil aviation safety evaluation based on deep belief network and principal component analysis. Safety Science, 112(6), 90–95. Web.
Nikolopoulos, K., & Petropoulos, F. (2018). Forecasting for big data: Does suboptimality matter? Computers and Operations Research, 98, 322–329. Web.
Niu, B., Li, J., Li, G., Poon, S., & Harrington, P. B. (2017). Analysis and modeling for big data in cancer research. BioMed Research International, 2017, 2–4. Web.
Noy, I., Shinar, D., & Horrey, W. (2018). Automated driving: Safety blind spots. Safety Science, 102(1), 68-78. Web.
Opresnik, D., & Taisch, M. (2015). The value of big data in servitization. International Journal of Production Economics, 165, 174–184. Web.
Padrón, S., Guimarans, D., Ramos, J., & Fitouri-Trabelsi, S. (2016). A bi-objective approach for scheduling ground-handling vehicles in airports. Computers and Operations Research, 71(1), 34-53. Web.
Palmarini, R., Erkoyuncu, J., Rajkumar, R., & Torabmostaedi, H. (2018). A systematic review of augmented reality applications in maintenance. Robotics and Computer-Integrated Manufacturing, 49(1), 215–228. Web.
Park, S. Y., & Pan, B. (2018). Identifying the next non-stop flying market with a big data approach. Tourism Management, 66, 411–421. Web.
Patil, P. (2017). Application for data mining and web data mining challenges. International Journal of Computer Science and Mobile Computing, 6(3), 39-44.
Phillips, S. (2016). New technologies simplify the airport experience. Airport Business, 30(5). 18-22.
Phillips, S., Thai, V. V., & Halim, Z. (2019). Airline value chain capabilities and CSR performance: The connection between CSR leadership and CSR culture with CSR performance, customer satisfaction and financial performance. Asian Journal of Shipping and Logistics, 35(1), 30–40. Web.
Pinto Leite, J. P. S., & Voskuijl, M. (2020). Optimal energy management for hybrid-electric aircraft. Aircraft Engineering and Aerospace Technology, 92(6), 851–861. Web.
Rahim, A. G. (2016). Perceived service quality and customer loyalty: The mediating effect of passenger satisfaction in the Nigerian airline industry. International Journal of Management and Economics, 52(1), 94–117. Web.
Ren, S., Zhang, Y., Liu, Y., Sakao, T., Huisingh, D., & Almeida, C. M. V. B. (2019). A comprehensive review of big data analytics throughout product lifecycle to support sustainable smart manufacturing: A framework, challenges and future research directions. Journal of Cleaner Production, 210(11), 1343–1365. Web.
Rezaei Somarin, A., Asian, S., Jolai, F., & Chen, S. (2018). Flexibility in service parts supply chain: a study on emergency resupply in aviation MRO. International Journal of Production Research, 56(10), 3547–3562. Web.
Rios Insua, D., Alfaro, C., Gomez, J., Hernandez-Coronado, P., & Bernal, F. (2018). A framework for risk management decisions in aviation safety at state level. Reliability Engineering and System Safety, 179, 74–82. Web.
Ross, J., Beath, C., & Sebastian, I. (2017). How to develop a great digital strategy. MIT Sloan Management Review, 58(2), 7-9.
Rusek, K., Guzik, P., & Chołda, P. (2016). Effective risk assessment in resilient communication networks. Journal of Network and Systems Management, 24(3), 491–515. Web.
Sadiku, M., Tembely, M., & Musa, S. (2016). Toward better understanding of business intelligence. Journal of Scientific and Engineering Research, 3(5), 89-91. Web.
Santos, M. Y., Oliveira e Sá, J., Andrade, C., Vale Lima, F., Costa, E., Costa, C., Martinho, B., & Galvão, J. (2017). A big data system supporting Bosch Braga Industry 4.0 strategy. International Journal of Information Management, 37(6), 750–760. Web.
Scherer, A., Wünderlich, N., & von Wangenheim, F. (2015). The value of self-service: Long-term effects of technology-based self-service usage on customer retention. MIS Quarterly, 39(1). 177-200. Web.
Schwartz, V. (2014). Dimanche à Orly: The Jet-age airport and the spectacle of technology between sky and earth. French Politics, Culture and Society, 32(3), 24-44. Web.
Selcuk, S. (2017). Predictive maintenance, its implementation and latest trends. Proceedings of the Institution of Mechanical Engineers, Part B: Journal of Engineering Manufacture, 231(9), 1670–1679. Web.
Si, Z., Chuai, G., Gao, W., Zhang, J., Chen, X., & Zhang, K. (2021). A QoS-based joint user association and resource allocation scheme in ultra-dense networks. Eurasip Journal on Wireless Communications and Networking, 2021(1). Web.
Skorupski, J., & Uchroński, P. (2016). Managing the process of passenger security control at an airport using the fuzzy inference system. Expert Systems with Applications, 54(3). 284-293. Web.
SME, M. (2015). Enhancing flight data monitoring and analysis can increase flight safety. Journal of Aeronautics & Aerospace Engineering, 04(02), 1–5. Web.
Søilen, K. (2015). A place for intelligence studies as a scientific discipline: Halmstad Sweden. Journal of Intelligence Studies in Business, 5(3), 35-46. Web.
Søilen, K.S. (2016). A research agenda for intelligence studies in business. Journal of Intelligence Studies in Business, 6(1), 21-36. Web.
Song, W., Wang, J., Bai, Y., Wang, L., Li, X., Tian, S., & Qi, X. (2021). Optical satellite sensor and positioning accuracy analysis for the Hongqi-1-H9 wide-range satellite in different terrains. Eurasip Journal on Wireless Communications and Networking, 2021(1), 1–15. Web.
Straker, K., & Wrigley, C. (2016). Translating emotional insights into digital channel designs. Journal of Hospitality & Tourism Technology, 7(2), 135-157. Web.
Sun, Z., Sun, L., & Strang, K. (2018). Big data analytics services for enhancing business intelligence. Journal of Computer Information Systems, 58(2), 162–169. Web.
Tapscott, D., & Tapscott, A. (2017). How Blockchain will change organizations, MIT Sloan Management Review, 58(2), 10-13.
Tokgöz, A., Bulkan, S., Zaim, S., Delen, D., & Torlak, N. 2018. Modeling airline MRO operations using a systems dynamics approach. Journal of Quality in Maintenance Engineering, 24(3), 280-310. Web.
Tretheway, M. W., & Markhvida, K. (2014). The aviation value chain: Economic returns and policy issues. Journal of Air Transport Management, 41, 3–16. Web.
Underwood, S. (2016). Blockchain beyond bitcoin. Communications of the ACM, 59(11), 15-17. Web.
Upadhya, V. (2016). Airport transformation through digital passenger experience. Airport Business, 30(4), 38-41.
Verhagen, W. J. C., & De Boer, L. W. M. (2018). Predictive maintenance for aircraft components using proportional hazard models. Journal of Industrial Information Integration, 12, 23–30. Web.
Vieira, D., & Loures, P. (2016). Maintenance, repair and overhaul (MRO) fundamentals and strategies: An aeronautical industry overview. International Journal of Computer Applications, 135(12), 21-29. Web.
Vranken, H. (2017). Sustainability of bitcoin and Blockchain. Current Opinion in Environmental Sustainability, 28(1), 1-9. Web.
Wang, S., Sun, G., Chen, W., & Zhong, Y. (2018). Database self-expansion based on artificial neural network: An approach in aircraft design. Aerospace Science and Technology, 72(10), 77–83. Web.
Wang, Z., Zeljic, K., Jiang, Q., Gu, Y., Wang, W., & Wang, Z. (2018). Dynamic network communication in the human functional connectome predicts perceptual variability in visual illusion. Cerebral Cortex, 28(1), 48–62. Web.
Washington, A., Clothier, R., & Williams, B. (2017). A Bayesian approach to system safety assessment and compliance assessment for unmanned aircraft systems. Journal of Air Transport Management, 62(1), 18-33. Web.
Wong, E., & Wong, W. (2017). The development of reusable luggage tag with the Internet of Things for mobile tracking and environmental sustainability. Sustainability, 9(1), 58-60. Web.
Xu, B., & Kumar, S. A. (2015). Big data analytics framework for system health monitoring. Proceedings – 2015 IEEE International Congress on Big Data, 2015, 401–408. Web.
Xu, H., Yuan, H., Ma, B., & Qian, Y. (2015). Where to go and what to play: Towards summarizing popular information from massive tourism blogs. Journal of Information Science, 41(6), 830-854. Web.
Zaharia, S., & Pietreanu, C. (2018). Challenges in airport digital transformation. Transportation Research Procedia, 35(1). 90-99. Web.
Zangiacomi, A., Oesterle, J., Fornasiero, R., Sacco, M., & Azevedo, A. (2017). The implementation of digital technologies for operations management: A case study for manufacturing apps’, Production Planning & Control, 28(16), 1318-1331. Web.
Zeng, Y., Wu, Q., & Zhang, R. (2019). Accessing from the sky: A tutorial on UAV communications for 5g and beyond. Proceedings of the IEEE, 107(12), 2327–2375. Web.
Zhang, L. (2020). Preliminary exploration of the reliability of civil aviation ATC communication network. Journal of Physics: Conference Series, 1533(2), 1-5. Web.
Zhang, X., Geng, J., Ma, J., Liu, H., Niu, S., & Mao, W. (2021). A hybrid service selection optimization algorithm in internet of things. Eurasip Journal on Wireless Communications and Networking, 2021(1), 1–13. Web.
Zheng, Z., Sangaiah, A. K., & Wang, T. (2018). Adaptive communication protocols in flying Ad Hoc network. IEEE Communications Magazine, 56(1), 136–142. Web.