In an era of intense technological progress, much attention has begun to be paid to the phenomenon of big data as a promising new idea for organizing and processing data. A reference to Figure 1 shows that public interest in the topic of big data has become exceptionally high in the last decade, which means that more and more users are becoming involved in this terminology. In general terms, big data should be understood as continuously increasing data sets of the same context that can be collected and presented in different formats. There is no universal definition of big data in research, as its use varies greatly depending on the scientific field and its application value. Suvarnamukhi and Seshashayee define big data as “a field related to the analysis and processing of large information that is available from various sources,” and this interpretation of the phenomenon is similar to the one above (Suvarnamukhi & Seshashayee, 2018, p. 712). Another study reports that big data is “a revolutionary concept to analyze the data to get accurate results and analysis in the interest of humans in many fields” (Desai, 2018, p. 737). Thus, a typical pattern in the definition of the term is that big data allows for processing digital data of gigantic volumes to manage it effectively.
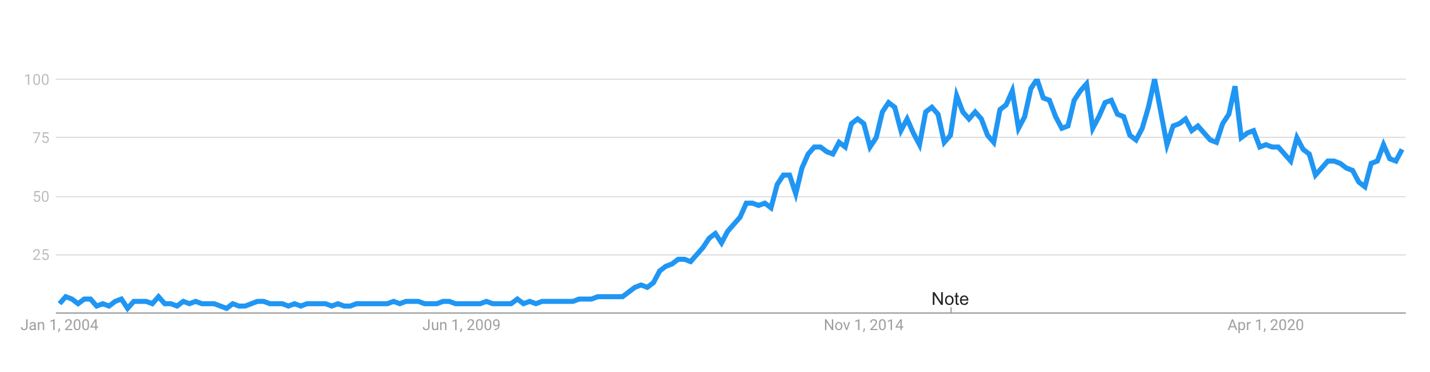
With such volumes of data, processing strategies become critically important. A practical solution is the strategy of parallel processing, in which several computing devices are involved in the simultaneous execution of several fragments of the same program — this allows to save time on processing significantly. For example, while the concept of big data is applicable to electronic medical records, parallel processing of clinical data allows fragmenting the overall task into an analysis of patient personal data patterns, research into the financial stability of the institution, HRM, strategic planning, and a number of other micro-tasks that big data helps manage. Understandably, delegating tasks increases cybersecurity concerns because it naturally creates the need for greater access to strategically important data, increasing the risks of human and technical errors (Le et al., 2018). That is, in fact, cybersecurity is rendered vulnerable in a parallel data processing environment because it creates an open system that is prone to cyberattacks and internal errors.
Data collection and processing are not always unified because data can exist in unstructured and structured forms. Unstructured data is data that is placed in repositories (data lakes) being unclassified, unordered, and collected from receiving devices “as is.” This type of data is not grouped into formats or categories, so repositories end up with data of different forms that are technically difficult to analyze without prior preparation. For example, if a user’s entire email archive is unloaded as textual information, such data will not be structured, though it is strictly textual. The opposite is structured data, which is classified and ordered, usually by highly organized tables. The search function is easy in an array of such data, and the individual component is semantic and meaningful. Data structured in the form of tables, charts, and diagrams are much easier to use for subsequent analysis and easier to perceive in processing.
This classification can be extended by adding the terms “repeating” and “non-repeating” data. In general, repeating data should be defined as any big data, whether structured or unstructured, that tends to repeat over time. For example, repeating unstructured data is data coming from sensors of electronic devices. In contrast to such unstructured but repetitive data, there are also non-repeating data that are unique in themselves — for example, elements of business correspondence or images. By analogy, structured, repetitive data is any highly organized array of data that can be collected systematically, such as quarterly payroll data for employees of the same company. In this case, structured but non-repeatable data could be, for example, the names of employees in a company’s database because they retain the uniqueness requirements and are organized at the same time.
Each of the data types described has business value. Structured and unstructured repeating data allows you to track business dynamics, while non-repeating data can be used to summarize all company data (all employees in an organization) and form a single database. Meanwhile, big data is associated with cybersecurity risks (Maayan, 2020). This refers to the likely threats of DDoS attacks and any malware that aims to steal data; understandably, the loss of an organization’s strategic data can be a crisis for the organization. The lack of structure in data also increases vulnerability to cyberattacks (Kish, 2019). In particular, the chaos of unstructured data makes it more difficult to protect data and use unified security domains.
During the processing of big data, three processes should be distinguished, which are summarized by the acronym ETL: extracting, transforming, and loading. The first phase, extracting, consists of obtaining data from external electronic devices. This is followed by the second phase, transforming, during which extracted data is converted and cleaned into a usable format for further use in business projects. Finally comes the download phase, during which the information is uploaded to repositories and other repositories to remain there until claimed; only the portion of the data that has been transformed in the previous phase is downloaded. There are several advantages to such a three-stage system, as it allows data to be extracted from multiple sources at once, conveniently tailored to business needs, and consistent use of computing power; meanwhile, ETL is also associated with a number of risks (Etleap, 2022). In particular, ETL is quite resource-intensive and difficult to adapt to changes since any transformations at one level affect the others.
References
Desai, P. V. (2018). A survey on big data applications and challenges [PDF document]. Web.
Etleap. (2022). Analyst-friendly and maintenance-free ETL. Web.
Kish, D. (2019). The truth about unstructured data. Security Magazine. Web.
Le, D. N., Khari, M., & Chetterjee, J. M. (2018). Cyber security in parallel and distributed computing [PDF document]. Web.
Maayan, G. D. (2020). Big data security: Challenges and solutions. Dataversity. Web.
Suvarnamukhi, B., & Seshashayee, M. (2018). Big data concepts and techniques in data processing. International Journal of Computer Sciences and Engineering, 6(10), 712-714.