The inherent human bias in data can be inherited by AI systems so that “the model learns certain biases against marginalized identities,” which “can be harmful in clinical settings” (Sezgin et al., 2022, p. 4). This human prejudice can manifest through inaccurate recommendations, offensive language, overlooking patient complaints, or affirming suicidal ideation (Sezgin et al., 2022). Clinical biases, such as historical under examination of marginalized populations or implicit biases of healthcare providers, can unintentionally introduce bias into the prediction models, which might entail unreliable diagnoses and treatments. AI systems may also fail to provide “up-to-date and reliable information, which may cause misinformation dissemination” (Sezgin, 2020, p. 2). Furthermore, as Yang et al. (2021) note, technology companies may employ advertisements to compensate for their development and deployment costs, which may introduce additional “bias toward a specific treatment or service” (p. 6).
“Clinical utility is a major concern for institutions,” meaning that successful implementation of AI systems requires strict evaluation frameworks to interpret model results and assess the quality of AI intervention (Sezgin, 2022, p. 4). Yang et al. (2021) report that the appropriateness level of clinical advice given by voice assistances (VA) ranges between 14%-29%, and Fisher’s exact test to assess accuracy between four different models showed no significant outperformance. The latter calls for enhanced assessment methods, as all four models use different AI systems. However, current evaluation methods rely on “black-box” deep-learning frameworks to generate a model interpretation, which due to limited transparency, results in “lower trust…and decrease the likelihood of the model being deployed” (Sezgin, 2022, p. 4).
The deployment of AI-specific to healthcare settings, initial training, and further fine-tuning to increase the accuracy of prediction models could involve years of careful testing and development that might entail considerable operational costs. This could be exacerbated by the underdevelopment of “hospital networks and EHR systems,” which would necessitate further investment to upgrade the systems (Sezgin et al., 2022, p. 4). Moreover, the deployment of VA for emergencies requires “establishing agreements and contracts for compliant services, creating data flow channels, and training AI,” which would entail extensive expenditure (Sezgin et al., 2020, p. 2). Although HIPAA compliance requirements ensure VA data protection, they may be deficient at regulating “new technologies and implementations that limit the utility of these technologies” (Sezgin et al., 2020).
To minimize human bias within gathered data, Sezgin et al. (2022) recommend complementing deployment with “active bias testing” to supervise, report, and respond to potential bias within produced model predictions (p. 4). This mechanism would ensure hospitals can employ VA effectively without inducing maltreatment or giving harmful advice. Additionally, Yang et al. (2021) suggest healthcare providers might have to delete search history and factory reset devices with each new patient to “minimize any bias that may occur from personalization.” (p. 2). Sezgin et al. (2020) note that one of the measures Google has taken to minimize misinformation is blocking “third-party developers adding VA apps for the Google Assistant,” which limits external human bias (p. 2).
Healthcare-specific assessment frameworks need to be realized to carefully assess the implications of AI intervention and ensure safe AI adoption. The development of explicit procedures and comprehensive evaluation frameworks is necessary for a significant adoption of VA technology (Sezgin et al., 2022). The authors further note that the collaborative effort between developers, healthcare providers, and data scientists to inform effective evaluation methodologies “is imperative” for a successful implementation of VA (p. 4). Yang et al. (2021) add that the development of model interpretation frameworks should involve evaluating whether a VA response provided or excluded potentially harmful information to patients.
To ease the financial burden, VA service providers may incorporate cloud computing platforms into the current hospital systems that are otherwise unable to manage the computational requirements of AI implementation. This would help alleviate the cost, as cloud computing services offer the specialized hardware designed to “run such models and can easily handle off-the-shelf networking and dynamic load balancing” (Sezgin et al., 2020, p. 3). Additionally, to prevent VA-driven advertising, the stakeholders, including hospitals and developers, should devise a healthcare-specific pay structure so that the former can attract companies and the latter ensure return on investment (Yang et al., 2021).
In summary, the VA implementation within healthcare settings involves challenges of the inherent human bias within data fed to AI systems, the lack of efficient evaluation systems for AI model interpretation, and financial hurdles. The provision of bias minimization mechanisms, the development of healthcare-specific evaluation frameworks, and the integration of cloud computing are recommended. Additionally, the analysis of competing VA systems’ implementation strategies and establishing a well-balanced reimbursement structure could be beneficial to ensure the successful implementation of VA technology.
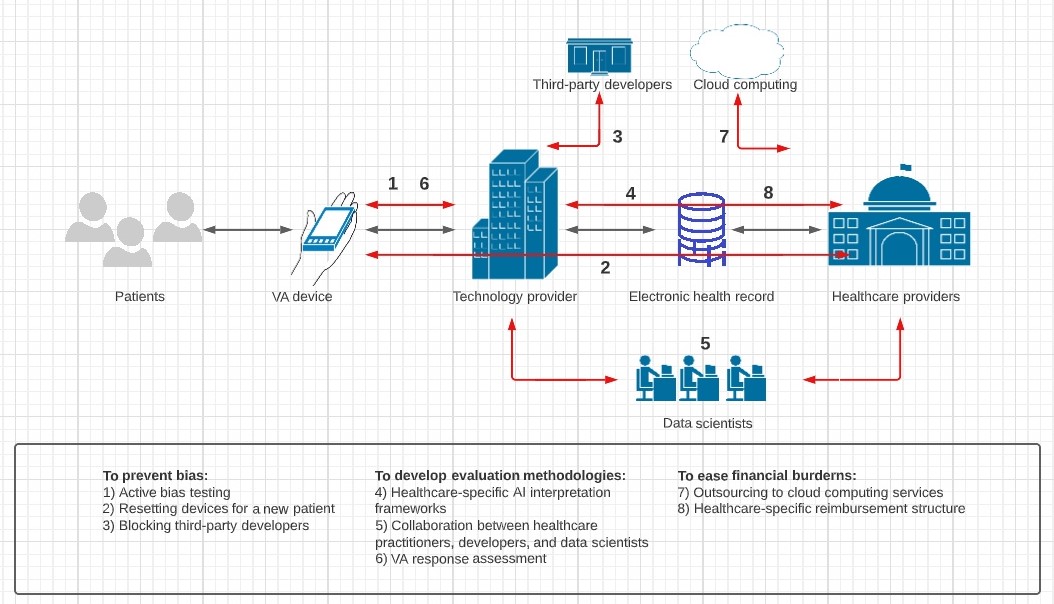
References
Sezgin, E., Huang, Y., Ramtekkar, U., & Lin, S. (2020). Readiness for voice assistants to support healthcare delivery during a health crisis and pandemic. NPJ digital medicine, 3, 122.
Sezgin, E., Sirrianni, J., & Linwood, S. L. (2022). Operationalizing and implementing pretrained, large artificial intelligence linguistic models in the US health care system: Outlook of Generative Pretrained Transformer 3 (GPT-3) as a Service Model. JMIR medical informatics, 10(2), e32875.
Yang, S., Lee, J., Sezgin, E., Bridge, J., & Lin, S. (2021). Clinical advice by voice assistants on postpartum depression: cross-sectional investigation using Apple Siri, Amazon Alexa, Google Assistant, and Microsoft Cortana. JMIR mHealth and uHealth, 9(1), e24045.